Enhancing Online Action Detection: Addressing Common Challenges via LSTR with Improved Modeling.
IEEE International Conference on Consumer Electronics(2024)
摘要
Most online action detection methods focus on solving a $K+1$ classification problem, where the additional category represents the ‘background’ class. However, training the ‘background’ class and dealing with data imbalance during training are common challenges in online action detection. $T_{0}$ address these challenges, we propose an effective model to mitigate the negative effects by incorporating an additional pathway between the feature extractor and action identification model Furthermore, we present two configurations for retaining the feature distinctions and supporting the final decision of the Long Short-Term Transformer (LSTR), aiming to enhance its performance in the $K+1$ classification. Experimental results show that the proposed method achieves an accuracy of 71% in mean average precision (mAP) on the Thumos 14 dataset, outperforming the 69.5% achieved by the original LSTR method.
更多查看译文
关键词
Online action detection,LSTR,action classification
AI 理解论文
溯源树
样例
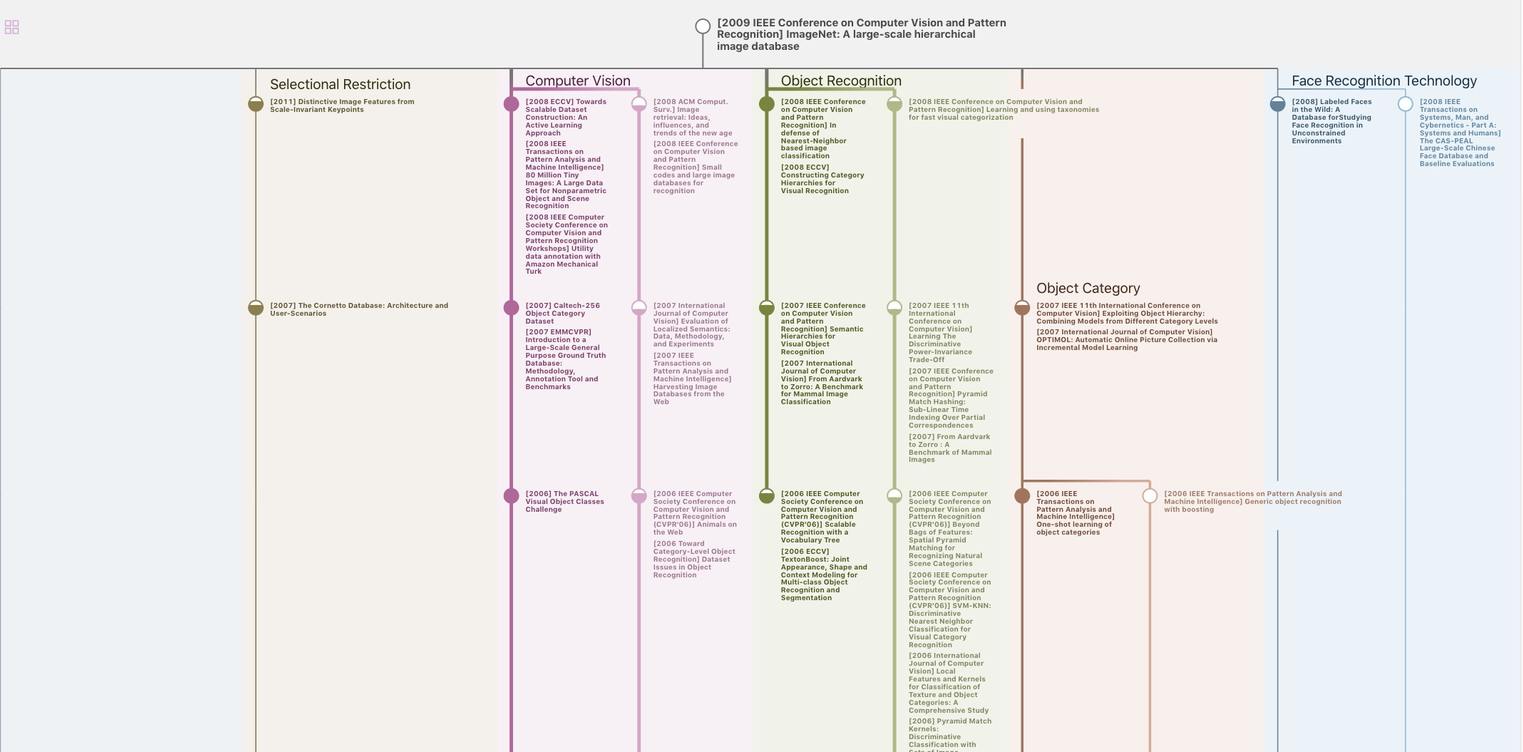
生成溯源树,研究论文发展脉络
Chat Paper
正在生成论文摘要