Graph Involutional Networks with Dynamic Feature Fusion for Skeleton-Based Action Recognition.
IEEE International Conference on Consumer Electronics(2024)
摘要
Graph convolution has been the fundamental operator of contemporary network architecture for skeleton-based action recognition tasks. In previous approaches, graph convolution focuses on aggregating local features and learning the discriminative motion pattern from different semantic representations. However, these local processing methods are inefficient for capturing long-range dependencies between distant nodes, such as clapping. Moreover, the combination of different semantic representations is usually implemented through direct operations, which can become a bottleneck for the network performance. To alleviate the above issues, we propose a novel Graph Involution (GI) operator for capturing richer dependencies and a Dynamic Feature Fusion (DFF) module to enlarge the receptive fields and adaptively fuse the different semantic representations. We leverage the GI operator and DFF module to construct an effective feature extractor and light weight model, DFF-GIN, which achieves comparable results on NTU RGB+D 60 datasets for skeleton-based action recognition.
更多查看译文
关键词
Feature Fusion,Action Recognition,Dynamic Fusion,Skeleton-based Action Recognition,Dynamic Feature Fusion,Receptive Field,Motion Patterns,Semantic Representations,Graph Convolution,Long-range Dependencies,Lightweight Model,Feature Fusion Module,Action Recognition Task,Computational Cost,Human Bone,Spatial Dimensions,Undirected,Convolution Operation,Batch Normalization,Model Architecture,Long-range Correlations,Semantic Features,Skeleton Data,Vertices,Motion Features,Human Activity Recognition,Attention Module,Multi-scale Feature Extraction,Body Joints,Edge Devices
AI 理解论文
溯源树
样例
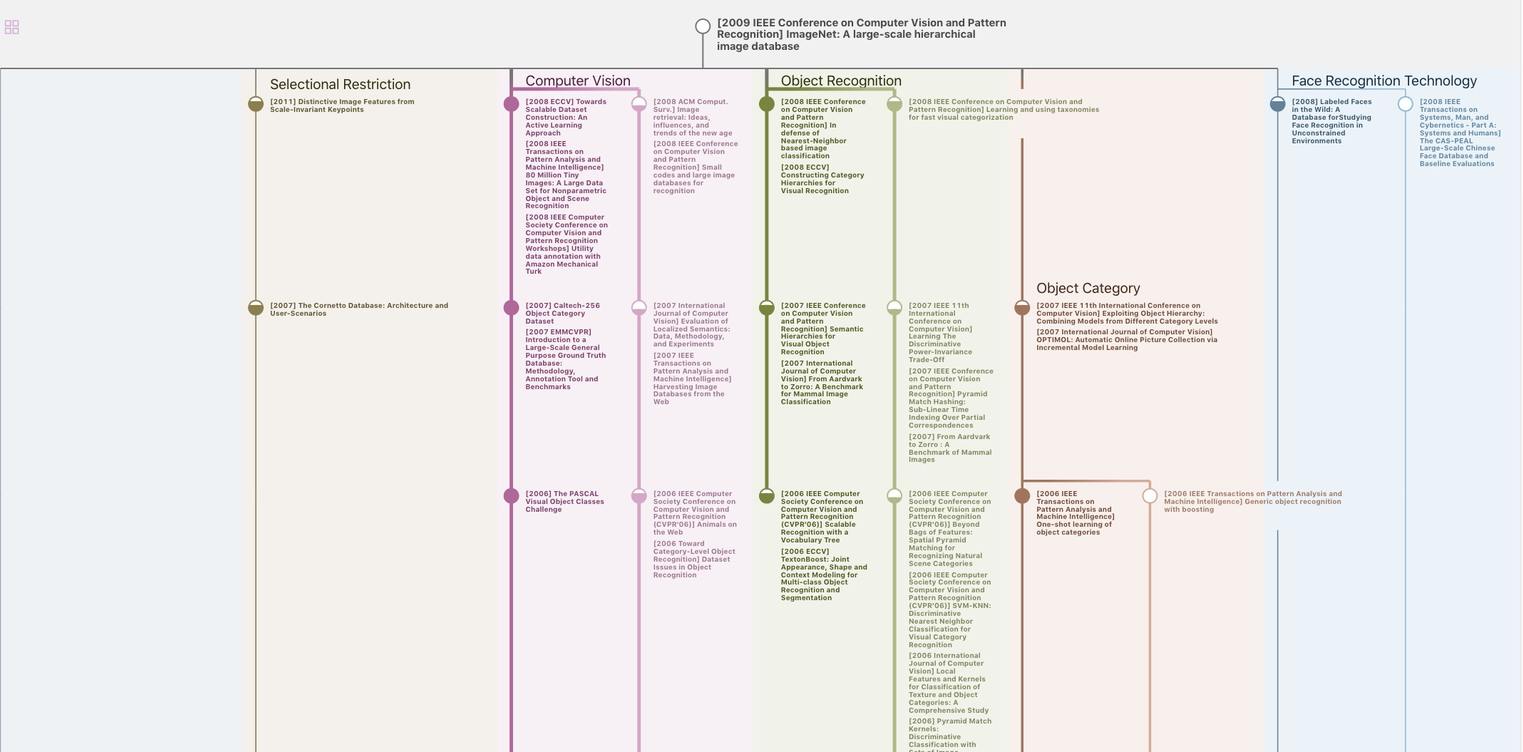
生成溯源树,研究论文发展脉络
Chat Paper
正在生成论文摘要