A Fast Parametric and Structural Transfer Leaky Integrator Echo State Network for Reservoir Computing
IEEE TRANSACTIONS ON SYSTEMS MAN CYBERNETICS-SYSTEMS(2024)
摘要
While leaky integrator echo state network (LI-ESN) has been a basic yet very effective tool for reservoir computing, its identical distribution assumption between training and testing samples is hurdling its applicability for time series scenarios where samples received in a sequential manner may often have different yet similar distributions (i.e., distribution shift) and even samples during current time period are insufficient. In order to circumvent such a serious challenge, by taking the samples during previous and current time periods as the source and target domains respectively and then using the proposed transfer learning strategy, the fast parametric and/or structural transfer LI-ESNs (i.e., PT-LI-ESN and PST-LI-ESN) are proposed in this study to keep LI-ESN's reservoir capacity and fast training, and simultaneously enhance its accuracy for insufficient target domain. In particular, except for its dynamically increasing reservoir size in one-by-one way so as to improve its accuracy, PST-LI-ESN still features fast training without any successive calculation of the inverse matrices during transfer learning. Experimental results on a heap of datasets from three benchmark sources indicate the effectiveness of the proposed PT-LI-ESN and PST-LI-ESN as well.
更多查看译文
关键词
Dynamically increasing reservoir,leaky integrator echo state network (LI-ESN),reservoir computing,time series,transfer learning
AI 理解论文
溯源树
样例
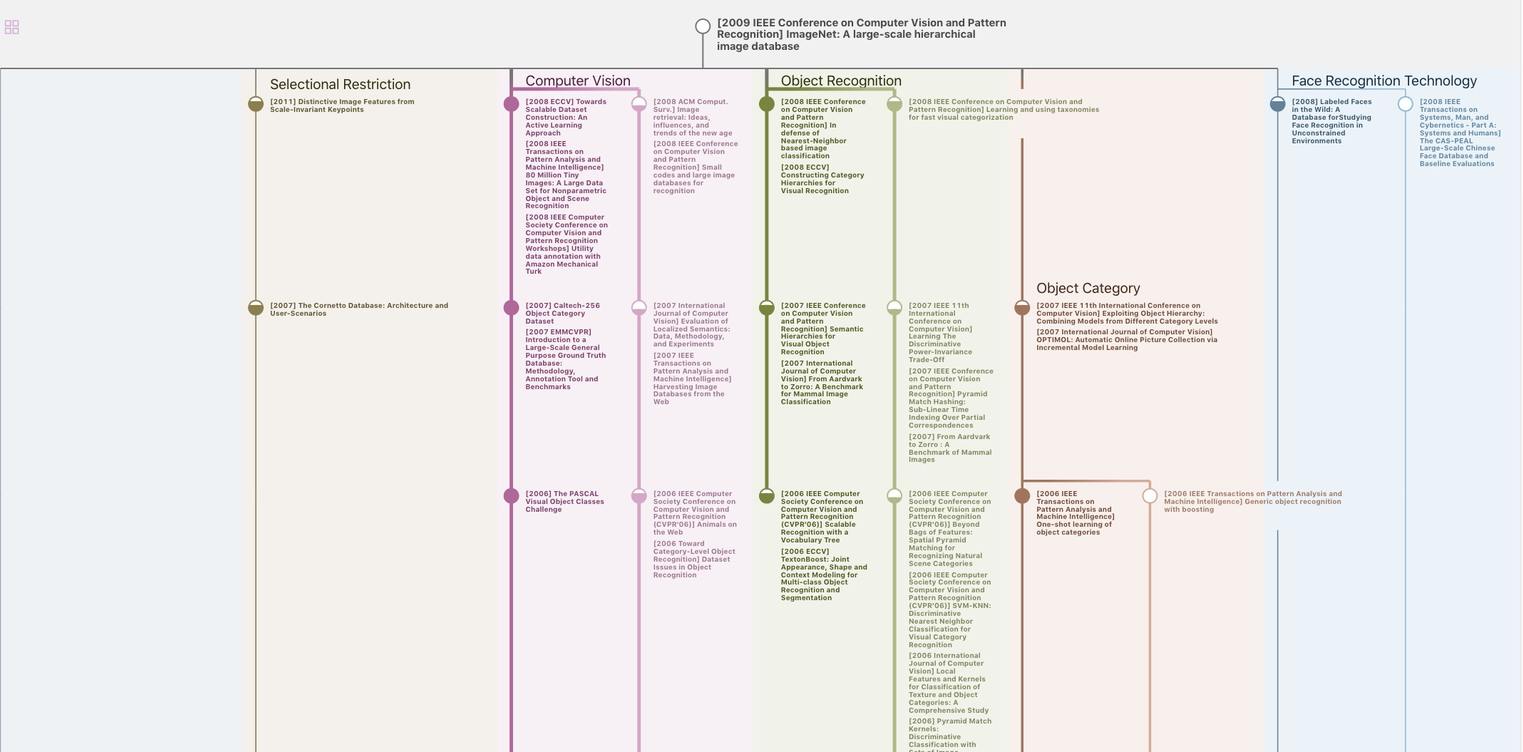
生成溯源树,研究论文发展脉络
Chat Paper
正在生成论文摘要