Spectrum-Energy-Efficient Mode Selection and Resource Allocation for Heterogeneous V2X Networks: A Federated Multi-Agent Deep Reinforcement Learning Approach
IEEE/ACM Transactions on Networking(2024)
摘要
Heterogeneous communication environments and broadcast feature of safety-critical messages bring great challenges to mode selection and resource allocation problem. In this paper, we propose a federated multi-agent deep reinforcement learning (DRL) scheme with action awareness to solve mode selection and resource allocation problem for ensuring quality of service (QoS) in heterogeneous V2X environments. The proposed scheme includes an action-observation-based DRL and a model parameter aggregation algorithm considering local model historical parameters. By observing the actions of adjacent agents and dynamically balancing the historical samples of rewards, the action-observation-based DRL can ensure fast convergence of each agent’ individual model. By randomly sampling historical model parameters and adding them to the foundation model aggregation process, the model parameter aggregation algorithm improves foundation model generalization. The generalized model is only sent to each new agent, so each old agent can retain the personality of its individual model. Simulation results show that the proposed scheme outperforms the comparison algorithms in the key performance indicators.
更多查看译文
关键词
Heterogeneous V2X network,mode selection,resource allocation,spectrum-energy-efficiency,deep reinforcement learning
AI 理解论文
溯源树
样例
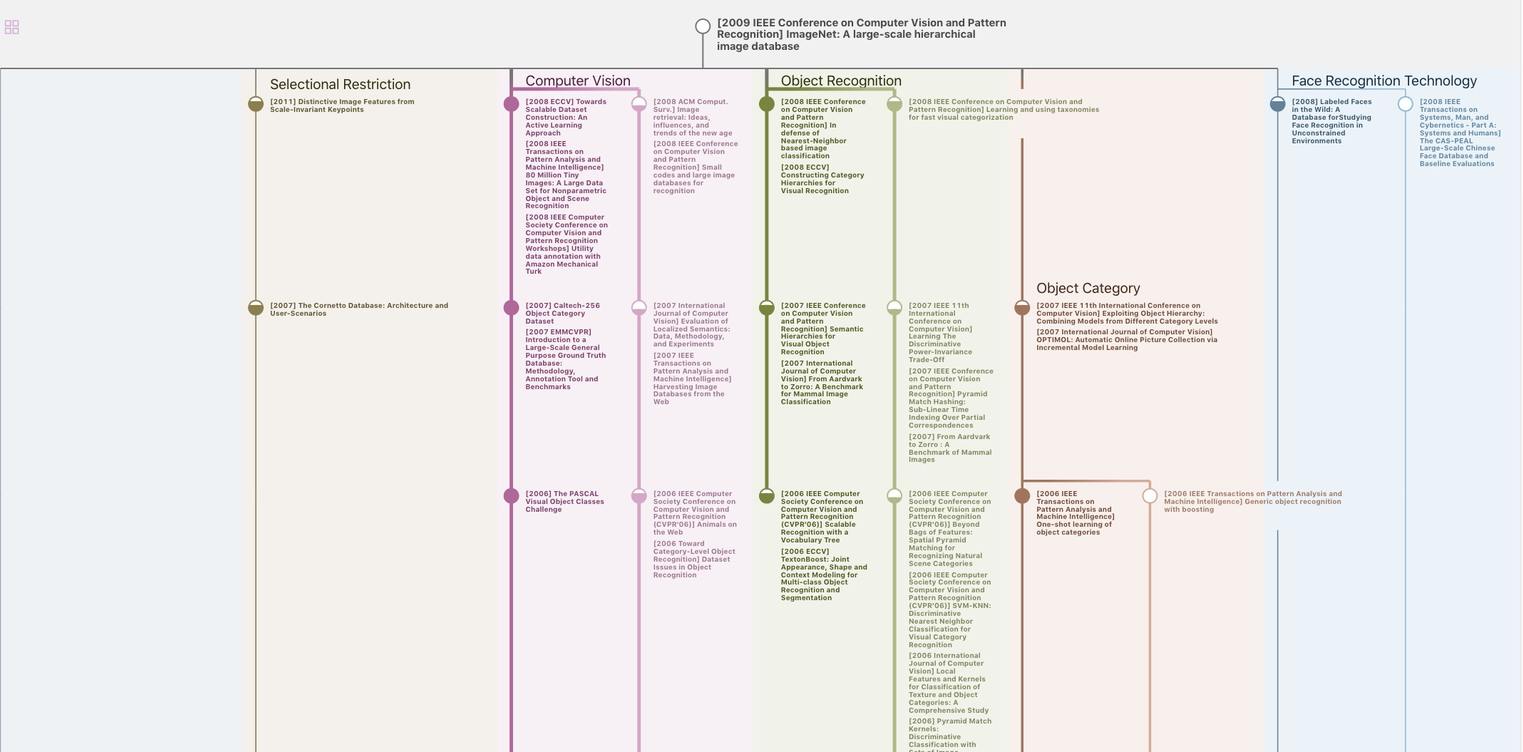
生成溯源树,研究论文发展脉络
Chat Paper
正在生成论文摘要