Machine Learning-Based Electrode-Level State of Health Estimation for NMC/Graphite Battery Cells
IEEE Transactions on Transportation Electrification(2024)
摘要
Accurate health diagnostics of lithium-ion batteries are critical for ensuring safe, reliable, and prolonged battery operation. This study presents a data-driven approach to estimating electrode-level state-of-health (eSOH) using a Deep Neural Network (DNN), enabling the assessment of the loss of active material (LAM) in both electrodes and the loss of lithium inventory (LLI). To construct the DNN models, essential features are extracted from the differential voltage and incremental capacity analyses of the open-circuit voltage (OCV), derived from a mechanistic model for an NMC/Graphite battery. The DNNs are trained and tested using various hyperparameters, leveraging the OCV data. Specifically, accuracy and robustness are considered in the model selection and evaluation process to balance precision and generalization ability. Additionally, feature reduction is performed considering three aspects: the number of features, robustness to noise, and the SOC window for data acquisition. Based on the analysis, three DNN models with reduced futures are meticulously chosen and extensively evaluated against the baseline all(12)-feature model, demonstrating their performance. Lastly, a correction method utilizing the entropy change of the electrodes is proposed to improve the estimation accuracy in the presence of temperature variations from the training phase.
更多查看译文
关键词
Lithium-ion battery,electrode-level state-of-health,feature reduction,correlation analysis,data-driven estimation,entropy change
AI 理解论文
溯源树
样例
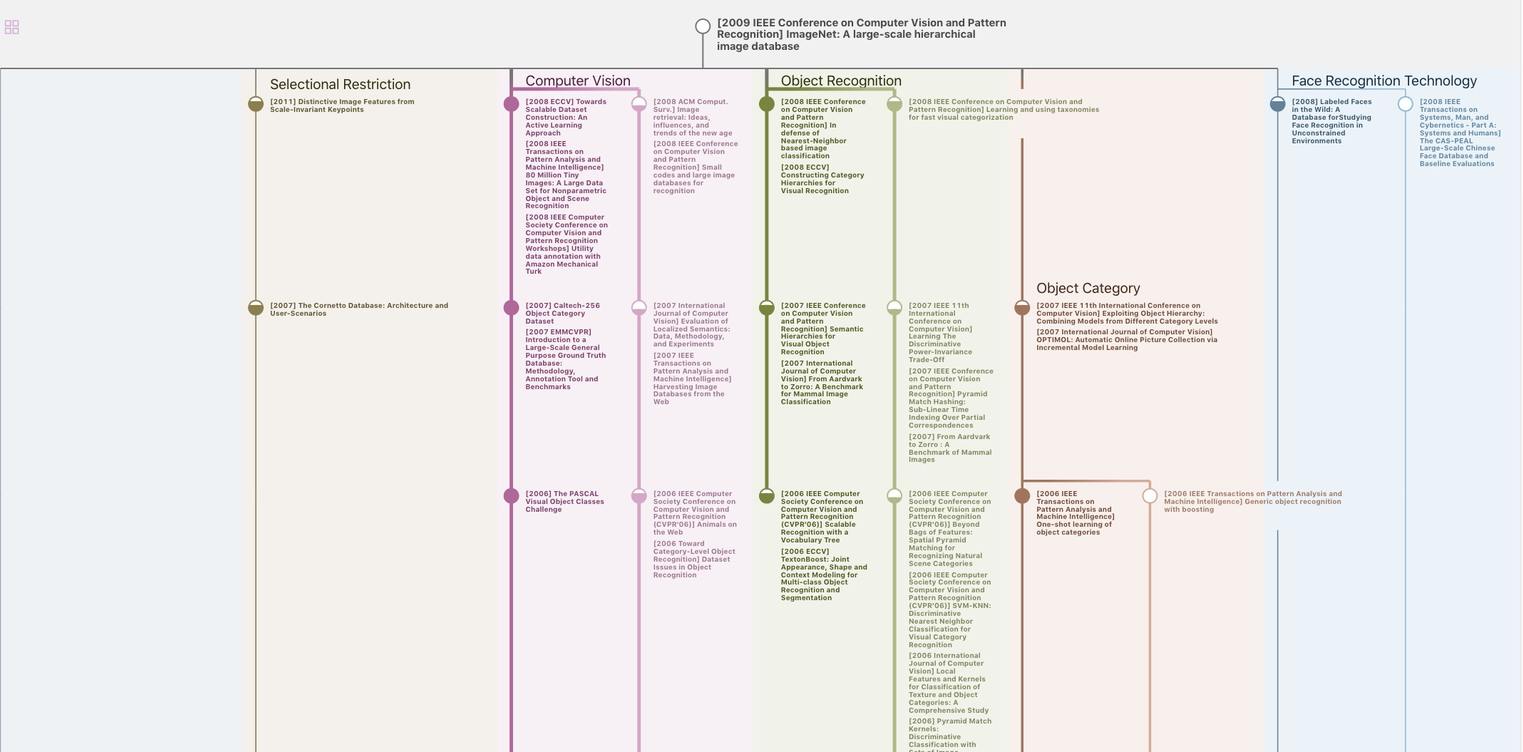
生成溯源树,研究论文发展脉络
Chat Paper
正在生成论文摘要