Distributed Gradient Tracking for Differentially Private Multi-Agent Optimization With a Dynamic Event-Triggered Mechanism
IEEE Transactions on Systems, Man, and Cybernetics: Systems(2024)
摘要
Distributed optimization achieves a minimized objective function through collaboration among distributed agents. Considering limited communication capabilities and privacy concerns, this article proposes a dynamic event-triggered differentially private gradient-tracking algorithm for distributed optimization. The communication requirement is reduced by event triggering, while the
$\epsilon$
-differential privacy is guaranteed by perturbations on states and the tracking of the average gradient. The convergence point is uniquely determined by the noise injected to the tracking. Sufficient conditions for stepsizes are established theoretically to guarantee the convergence in mean and almost surely. Moreover, the theoretical privacy level is rigorously obtained and the positive effect of the event-triggered communication on the privacy is also discussed. Simulations are conducted for the classification of the dataset on the stability of a 4-node star power system to verify the theoretical findings.
更多查看译文
关键词
Differential privacy,distributed optimization,dynamic event-triggered mechanism
AI 理解论文
溯源树
样例
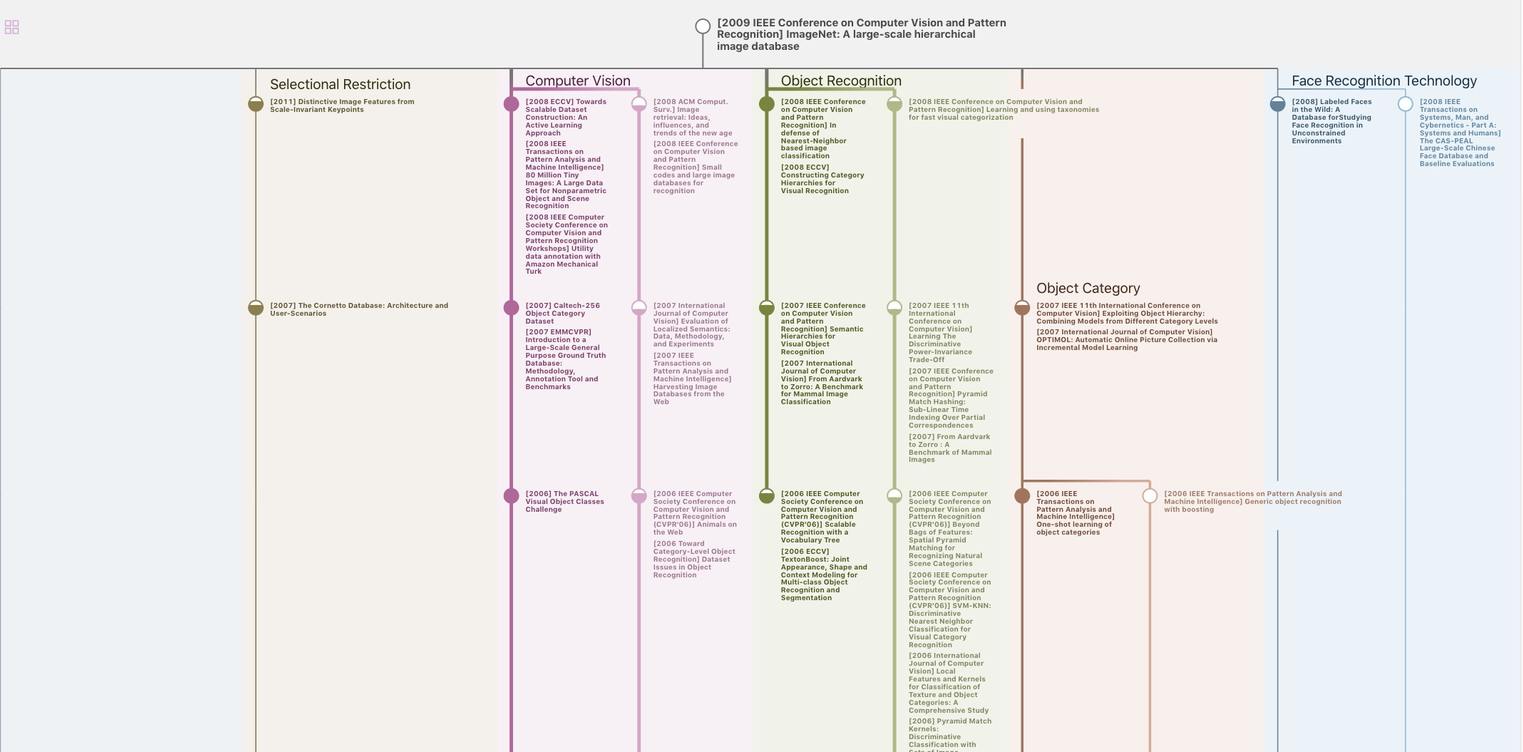
生成溯源树,研究论文发展脉络
Chat Paper
正在生成论文摘要