FLNA: Flexibly Accelerating Feature Learning Networks for Large-Scale Point Clouds With Efficient Dataflow Decoupling
IEEE TRANSACTIONS ON VERY LARGE SCALE INTEGRATION (VLSI) SYSTEMS(2024)
摘要
Point cloud-based 3-D perception is poised to become a key workload on various applications. It always leverages a feature learning network (FLN) before backbones to obtain uniform representation from the scattered points. Grid-based FLN (GFLN) that partitions point clouds into uniform grids becomes the main category in recent state-of-the-art (SOTA) works. However, it heavily suffers from significant memory and computation inefficiency associated with high point sparsity and critical data dependency. To address these troubles, we propose FLNA, a GFLN accelerator with algorithm-architecture co-optimization for large-scale point clouds. At the algorithm level, the dataflow-decoupling strategy is adopted to alleviate the processing bottlenecks from pipeline dependency, which also reduces 78.3% computation cost by exploiting the redundancy from inherent sparsity and special operators. Based on the algorithm co-optimization, an effective architecture is designed with efficient GFLN mapping and block-wise processing strategies. It manages to improve on-chip memory efficiency tremendously through diverse techniques, including linked-list-based block lookup table (LUT) and transposed feature organization. Extensively evaluated on representative benchmarks, FLNA achieves 69.9-264.4 x speedup with more than 99% energy savings compared to multiple GPUs and CPU. It also demonstrates a substantial performance boost over the SOTA point cloud accelerators while providing superior support of large-scale point clouds.
更多查看译文
关键词
Point cloud compression,Memory management,Feature extraction,Pipelines,Computational efficiency,System-on-chip,Neural networks,Algorithm-architecture co-design,feature learning network (FLN),neural network accelerator,point cloud
AI 理解论文
溯源树
样例
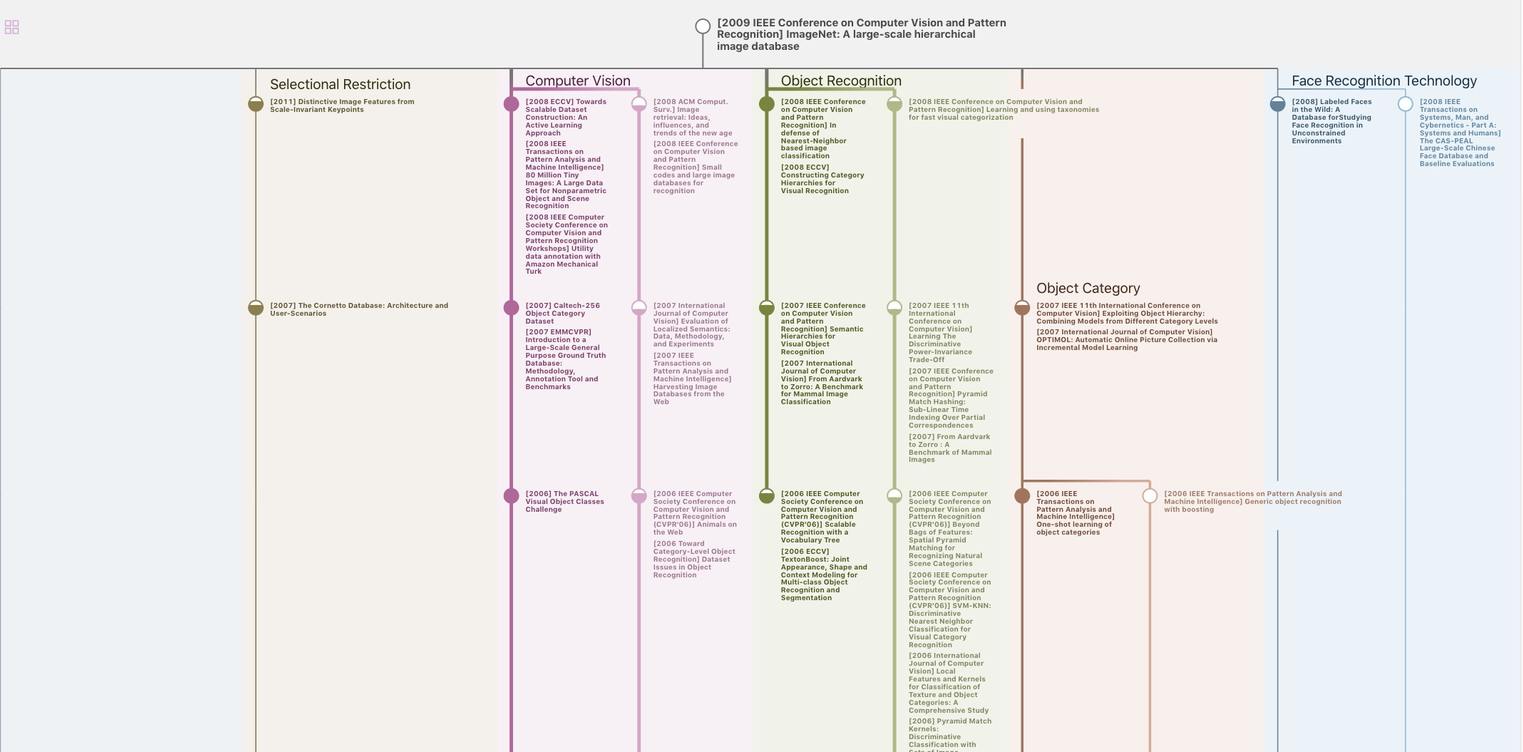
生成溯源树,研究论文发展脉络
Chat Paper
正在生成论文摘要