Graph Temporal Attention Network for Imbalanced Wind Turbine Blade Icing Prediction
IEEE Sensors Journal(2024)
摘要
The conventional approach to mitigating wind turbine blade icing is associated with high costs, and wind farms are susceptible to icing-related challenges. In pursuit of enhanced blade icing prediction, this study introduces a data-driven solution known as the Graph Temporal Attention Network (GTAN) model. This model incorporates a Feature Extractor module aimed at enhancing distinctions among various categories of raw sensor data. Additionally, it integrates a Temporal Attention (TA) mechanism to heighten sensitivity to temporal characteristics. Baseline experiments are conducted using supervisory control and data acquisition (SCADA) data from three wind turbines, revealing that this method surpasses other baseline networks in the realm of multidimensional time series classification. Furthermore, comprehensive ablation and robustness analyses validate the efficacy of the designed model components and underscore its resilience. Notably, the utilization of a specialized loss function tailored for imbalanced wind turbine blade icing data yields a substantial improvement in the prediction accuracy of minority classes. Consequently, this data-driven model exhibits the capability to accurately forecast blade icing occurrences, thereby contributing to reduced maintenance costs within wind farms.
更多查看译文
关键词
Blade icing prediction,Deep learning,Graph Attention Networks,Time series
AI 理解论文
溯源树
样例
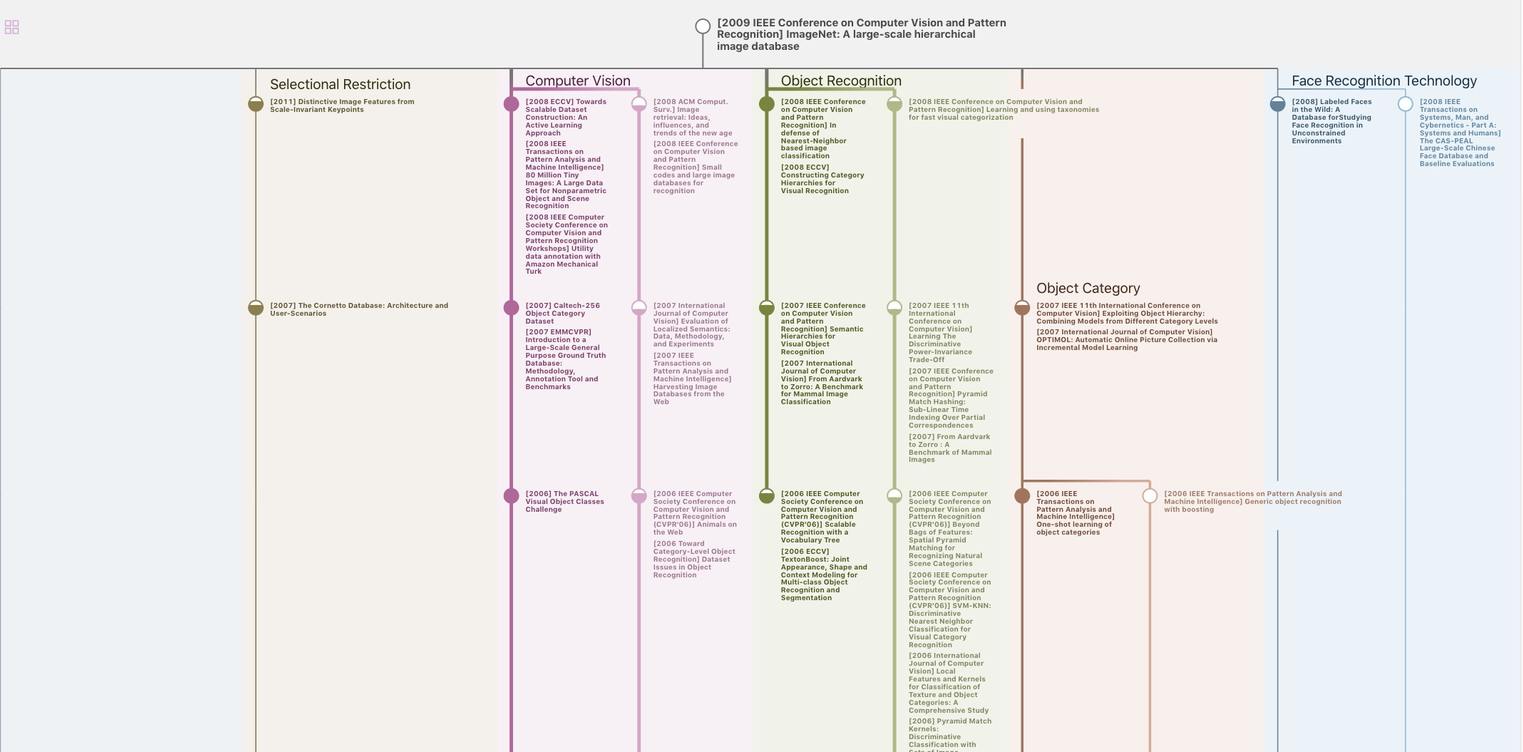
生成溯源树,研究论文发展脉络
Chat Paper
正在生成论文摘要