Data-Driven Robust Multimodal Multiobjective Particle Swarm Optimization
IEEE Transactions on Systems, Man, and Cybernetics: Systems(2024)
摘要
For the data-driven multimodal multiobjective optimization problems (MMOPs), the inevitable uncertainties will lead to distortion of multiple peak landscapes, thus causing slow convergence in complex landscapes. To solve this problem, a robust multimodal multiobjective particle swarm optimization (RMMPSO) is designed to alleviate slow convergence. There are three novelties in RMMPSO. First, a perturbation observer is proposed to detect perturbation in the fixed point of variance to evaluate the influences of disturbed recording position on convergence. Second, an adaptive adjustment mechanism, based on the perturbation observer, is designed to obtain reasonable search ranges and suppress the abnormal changes in convergence, so as to improve convergence performance. Third, a Lipschitz-based exploitation strategy is designed to search for reliable solutions, which reduces the optimal offset caused by uncertainties. Finally, the effectiveness of RMMPSO is demonstrated in terms of multiobjective multimodal benchmark problems with uncertain components and wastewater treatment simulation platform. The results of experiments demonstrate the superiority of RMMPSO in solving data-driven MMOPs compared to state-of-the-art multimodal multiobjective algorithms.
更多查看译文
关键词
Data-driven,multimodal multiobjective optimization algorithm,particle swarm optimization (PSO),robust
AI 理解论文
溯源树
样例
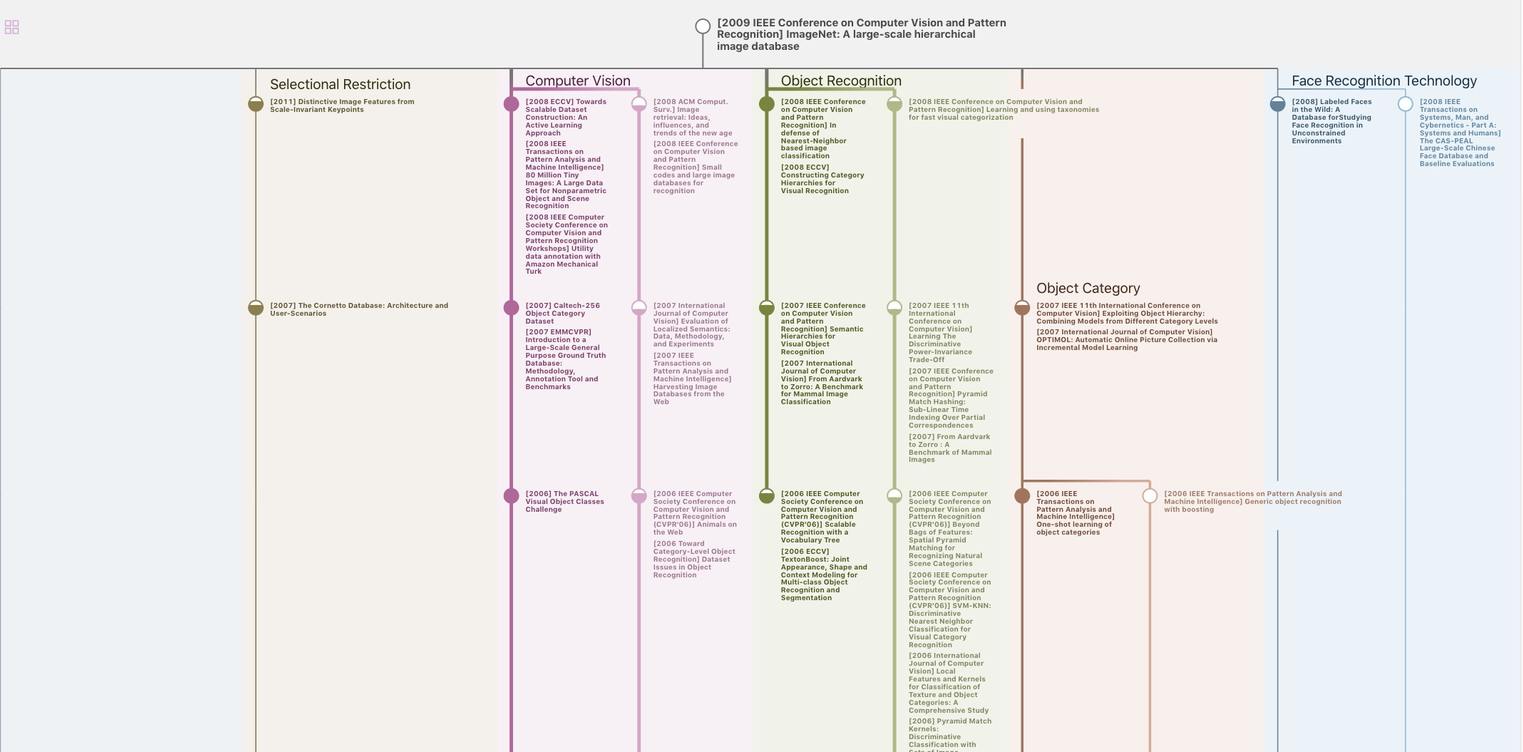
生成溯源树,研究论文发展脉络
Chat Paper
正在生成论文摘要