Large-Scale Multiobjective Optimization for Watershed Planning and Assessment
IEEE TRANSACTIONS ON SYSTEMS MAN CYBERNETICS-SYSTEMS(2024)
摘要
Selecting the appropriate best management practices (BMPs) is crucial for reducing pollution levels and improving the watershed's water quality. However, identifying cost-effective BMP combinations for various locations is challenging, especially when using computationally expensive evaluation procedures like the Chesapeake Assessment Scenario Tool (CAST). This study presents a customized and hybrid evolutionary multiobjective optimization (EMO) algorithm aimed at enhancing the water quality in the Chesapeake Bay Watershed for two conflicting objectives: 1) cost of BMP implementation and 2) the amount of resulting nitrogen loading to streams. First, we present a surrogate model-based optimization approach and evaluate its accuracy and execution time against the CAST evaluation system. Then, we present a hybrid two-stage EMO procedure, which is initialized with solutions obtained from a point-based $\epsilon$ -constraint procedure and works with a repair operator to satisfy equality constraints. The hybrid EMO procedure yields a set of nondominated tradeoff solutions for problems with as few as 1012 variables (West Virginia's Tucker County) to as large as 153 818 variables (the whole state of West Virginia). Alternate tradeoff solutions provide a knowledge of different possible options and also importantly provide a flexible method of arriving at a single preferred solution for deployment. The EMO procedure is then integrated with CAST using recent RESTful API approaches, and interesting accuracy versus computational tradeoffs are discussed. Finally, a number of interesting insights of the scale-up optimization study reveal promising strategies to scale the application to multiple counties and the watershed level.
更多查看译文
关键词
Optimization,Watersheds,Nitrogen,Costs,Computational modeling,Water quality,Complexity theory,Best management practices (BMPs),Chesapeake Bay Watershed (CBW),evolutionary multiobjective optimization (EMO),hybrid approach,large-scale optimization,watershed optimization
AI 理解论文
溯源树
样例
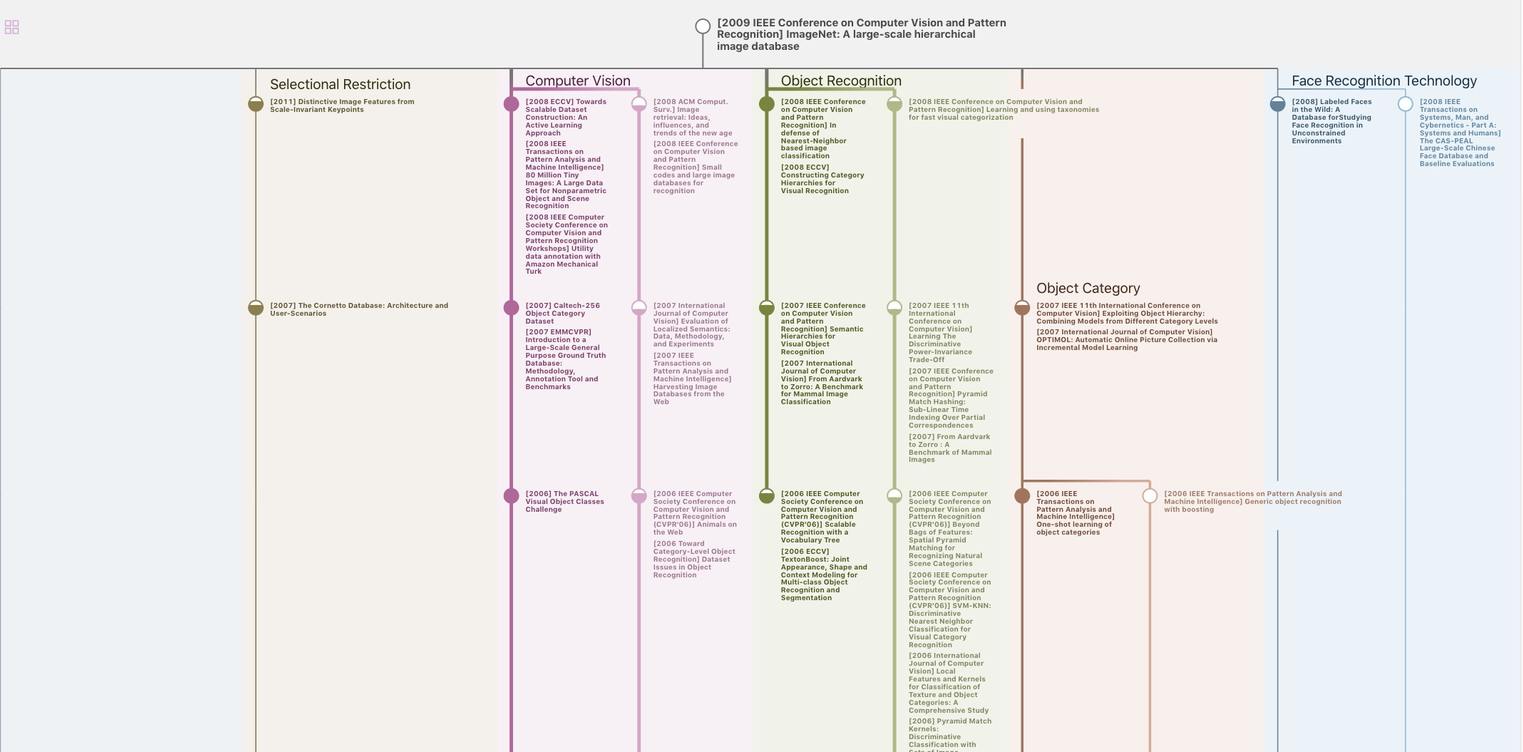
生成溯源树,研究论文发展脉络
Chat Paper
正在生成论文摘要