Self-Triggered Approximate Optimal Neuro-Control for Nonlinear Systems Through Adaptive Dynamic Programming
IEEE TRANSACTIONS ON NEURAL NETWORKS AND LEARNING SYSTEMS(2024)
摘要
In this article, a novel self-triggered approximate optimal neuro-control scheme is presented for nonlinear systems by utilizing adaptive dynamic programming (ADP). According to the Bellman principle of optimality, the cost function of the general nonlinear system is approximated by building a critic neural network with a nested updating weight vector. Thus, the Hamilton-Jacobi-Bellman equation is solved to indirectly obtain the approximate optimal neuro-control input. In order to reduce the computation, the communication bandwidth, and the energy consumption, an appropriate self-triggering condition is designed as an alternative way to predict the updating time instants of the approximate optimal neuro-control policy. On the basis of Lyapunov's direct method, the stability of the closed-loop nonlinear system is analyzed and guaranteed to be uniformly ultimately bounded. Simulation results of two practical systems illustrate the present ADP-based self-triggered approximate optimal neuro-control scheme to be reasonable and effective.
更多查看译文
关键词
Adaptive dynamic programming (ADP),neural networks (NNs),optimal control,reinforcement learning,self-triggered control
AI 理解论文
溯源树
样例
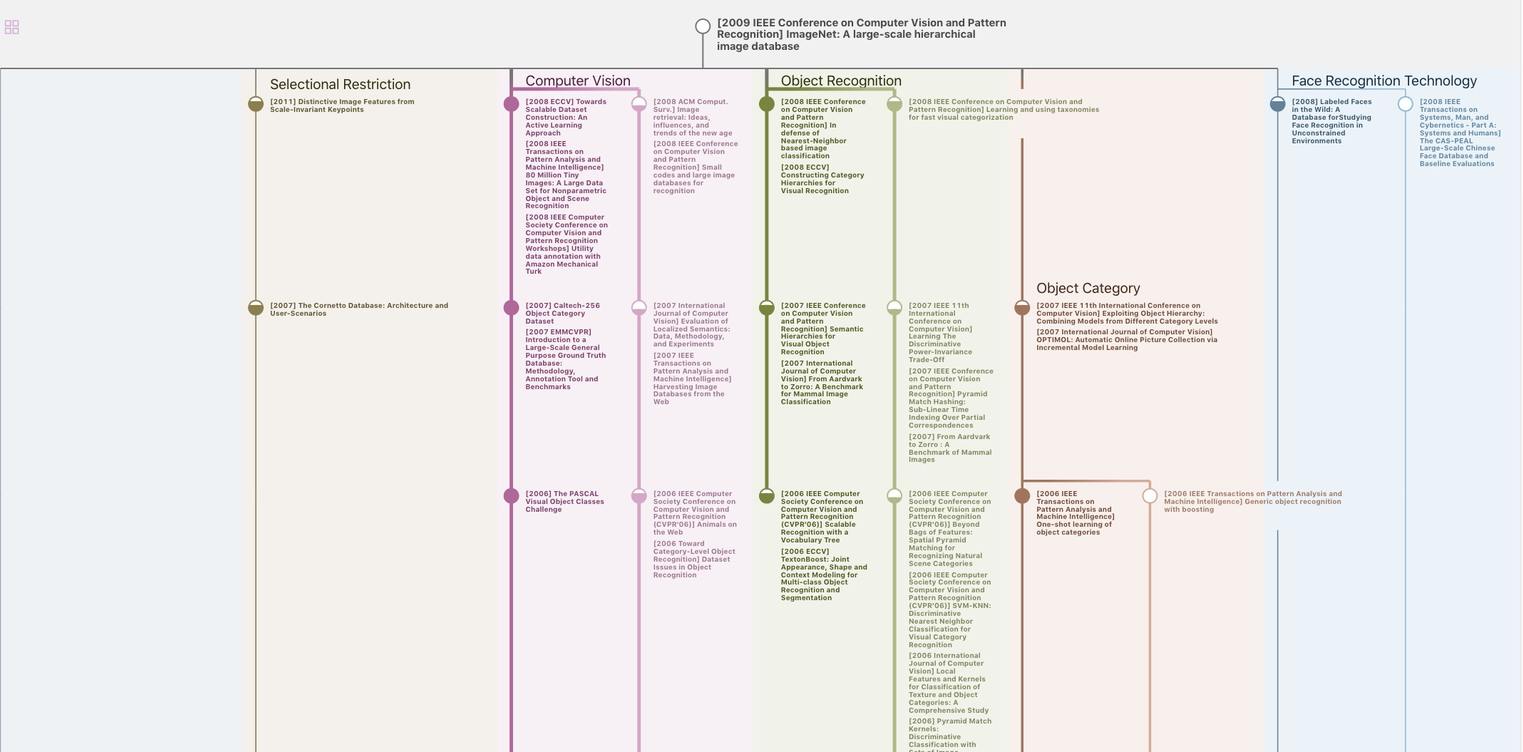
生成溯源树,研究论文发展脉络
Chat Paper
正在生成论文摘要