DeepFPD: Browser Fingerprinting Detection via Deep Learning With Multimodal Learning and Attention
IEEE Transactions on Reliability(2024)
摘要
Browser fingerprinting is a stateless tracking technique that poses a significant security threat to users' privacy. However, the distinction between fingerprinting and nonfingerprinting scripts is far from well-defined, making the detection of fingerprinting scripts very challenging. Existing methods for detecting browser fingerprinting are based on heuristics or machine learning, and thus either require strictly defined rules or are not able to learn the features of fingerprinting scripts comprehensively, failing to detect a significant fraction of fingerprinting scripts. To detect browser fingerprinting more effectively, we propose a deep learning-based detection method,
DeepFPD
, in which multiple script modalities including tokens, abstract syntax trees, and control flow graphs are learned by using different specific neural networks to obtain lexical, syntax, and control flow information of the script code. Moreover, the attention mechanism is introduced to enhance the effectiveness of
DeepFPD
. The experimental results on the training dataset and test dataset constructed based on real-world scripts show that
DeepFPD
outperforms the state-of-the-art work with an F1-measure improvement of 8.3% and 18.7%, respectively.
更多查看译文
关键词
Attention mechanism,browser fingerprinting detection,multimodal learning,tracking,web privacy
AI 理解论文
溯源树
样例
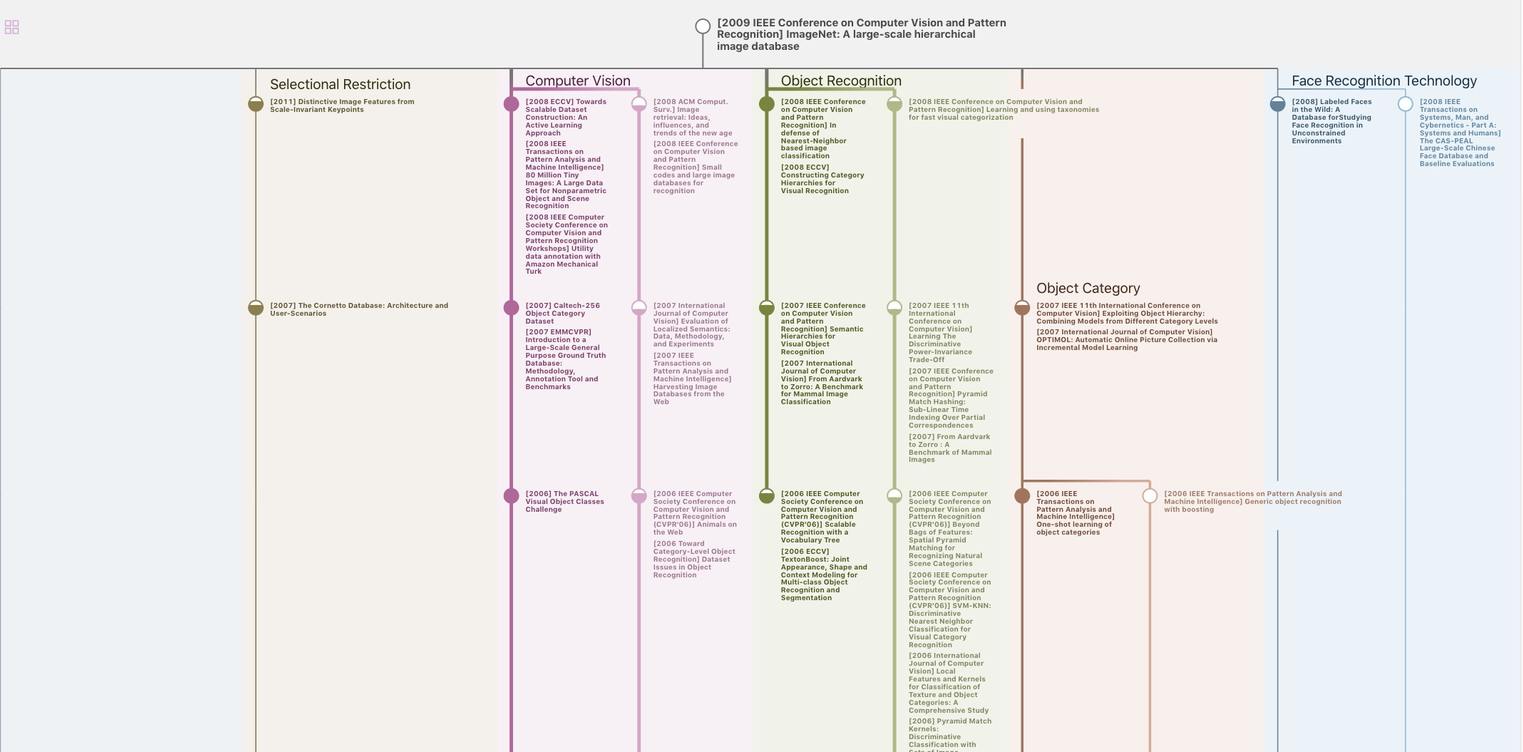
生成溯源树,研究论文发展脉络
Chat Paper
正在生成论文摘要