Data Protection Method Against Cross-Domain Inference Threat in Cyber-Physical Power Grid
IEEE Transactions on Smart Grid(2024)
摘要
Recent advancement of data-driven methods has enabled the mining of associations among various data sets, particularly those generated from cyber-physical power grids (CPPG) where different measurements may be inherently coupled by an underlying system. As such, attackers may infer sensitive parameters jointly from several publicly shared electricity data across different security domains based on their revealed associations. Traditional data protection methods, mainly focusing on reducing the risks of direct data probing, may not be applicable to properly guard sensitive information in such scenarios. In this paper, we elaborate on such a data security issue, namely cross-domain inference (CDI), and then propose the decoder-ensemble variational autoencoder (DE-VAE) which is used for noise-adding to protect against such attack. The DE-VAE is designed with a mutual information maximization scheme to preserve the original usability of the noise-added data. Meanwhile, a multi-objective optimization is solved to achieve a trade-off between privacy protection and data usability. Our protection method is evaluated on experiments involving four real-world scenarios in the modern sensor-rich smart power grid. The experimental results show that our method can provide effective protection ability against CDI threats without sacrificing much usability, and achieves a performance gain of more than 45% compared to several non-trivial baselines.
更多查看译文
关键词
data security,cross-domain inference,data protection,generative learning
AI 理解论文
溯源树
样例
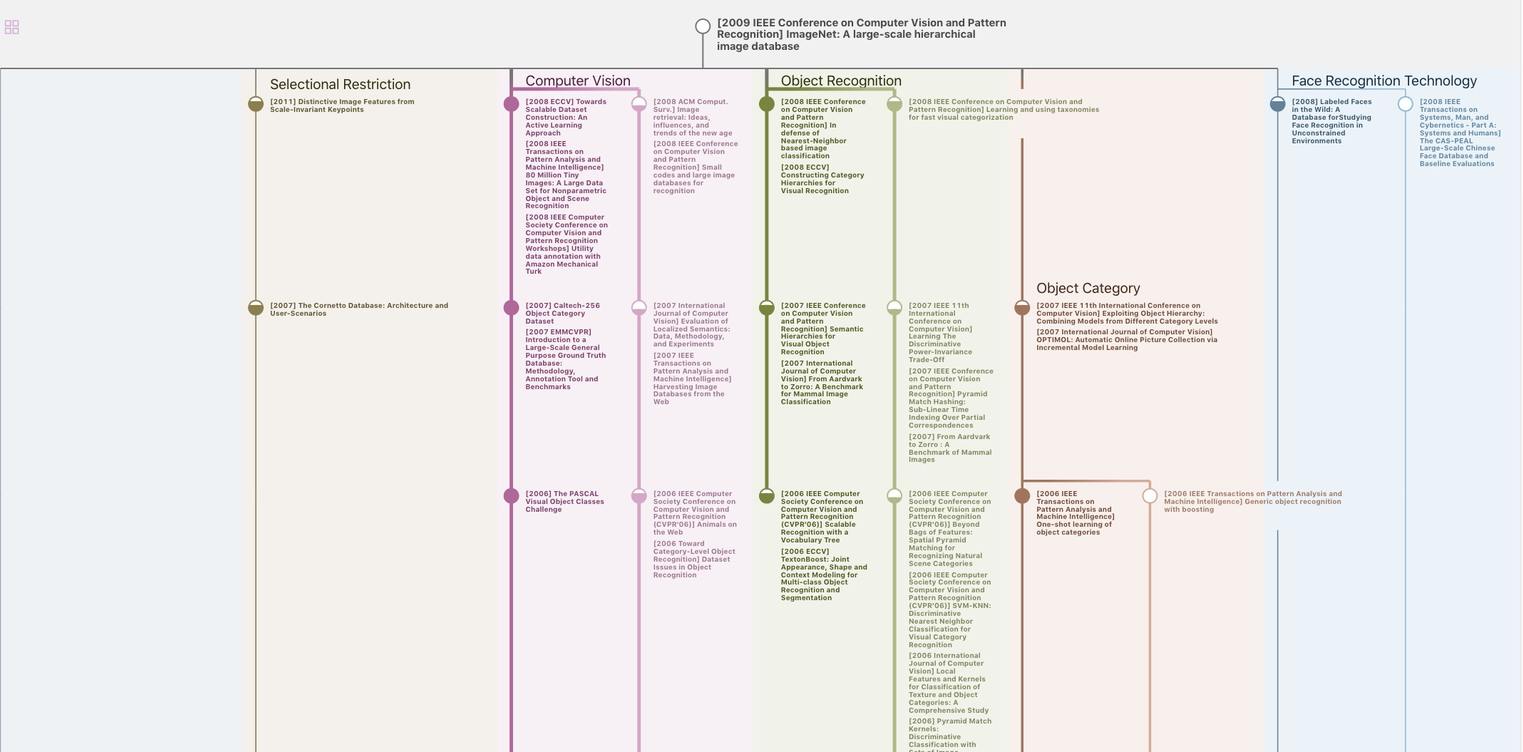
生成溯源树,研究论文发展脉络
Chat Paper
正在生成论文摘要