Towards Secure and Robust Federated Distillation in Distributed Cloud: Challenges and Design Issues
IEEE Network(2024)
摘要
Federated learning (FL) offers a promising solution for effectively leveraging the data scattered across the distributed cloud system. Despite its potential, the huge communication overhead greatly burdens the distributed cloud system. Federated distillation (FD) is a novel distributed learning technique with low communication cost, in which the clients communicate only the model logits rather than the model parameters. However, FD faces challenges related to data heterogeneity and security. Additionally, the conventional aggregation method in FD is vulnerable to malicious uploads. In this article, we discuss the limitations of FL and the challenges of FD in the context of distributed cloud system. To address these issues, we propose a blockchain-based framework to achieve secure and robust FD. Specifically, we develop a pre-training data preparation method to reduce data distribution heterogeneity and an aggregation method to enhance the robustness of the aggregation process. Moreover, a committee/workers selection strategy is devised to optimize the task allocation among clients. Experimental evaluations are conducted to evaluate the effectiveness of the proposed framework.
更多查看译文
AI 理解论文
溯源树
样例
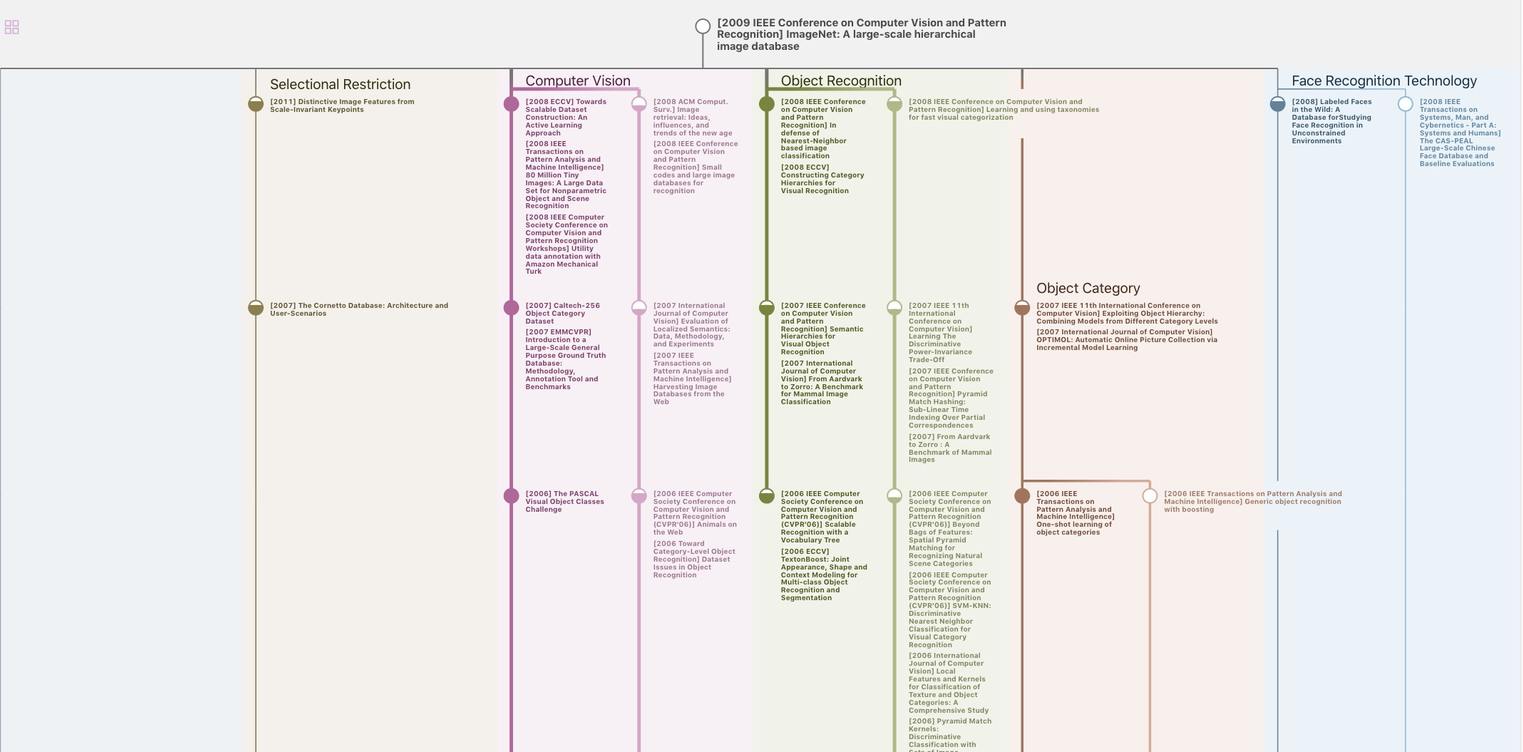
生成溯源树,研究论文发展脉络
Chat Paper
正在生成论文摘要