iMCU: A 28-nm Digital In-Memory Computing-Based Microcontroller Unit for TinyML
IEEE JOURNAL OF SOLID-STATE CIRCUITS(2024)
摘要
Tiny machine learning (TinyML) envisions executing a deep neural network (DNN)-based inference on an edge device for improving battery life, latency, security, and privacy. Toward this vision, recent microcontroller units (MCUs) integrate in-memory computing (IMC) hardware to leverage its high energy efficiency and throughput in vector-matrix multiplication (VMM). However, those existing works require large IMC hardware, severely increasing the area overhead. In addition, most existing works use analog-mixed-signal (AMS) IMC hardware, exhibiting limited robustness over process, voltage, and temperature (PVT) variations. Finally, none can support a practical software development framework such as TensorFlow Lite for Microcontrollers (TFLite-micro). Due to these limitations, those MCUs did not present the performance for the standard benchmark MLPerf-Tiny, which makes it difficult to evaluate them against the state-of-the-art neural (not necessarily IMC-based) MCUs. In this article, we design a new IMC-based MCU, titled iMCU, for TinyML to address those challenges. In the design process, we: 1) define the optimal set of acceleration targets and 2) devise an area-efficient computation flow that requires the least amount of IMC hardware yet still provides a significant acceleration. In addition, we develop: 1) state-of-the-art digital IMC macros and 2) create the accelerator based on the macros, which can support the proposed computation flow in a fully pipelined manner. Combining those innovations, we prototyped the iMCU in a 28-nm CMOS. The measurement results show that the iMCU significantly outperforms the prior IMC-based MCUs in compute density, energy efficiency, and SRAM density (total SRAM size/total SRAM area). It also achieves a compact footprint of 2.73 mm(2).
更多查看译文
关键词
Deep learning,hardware/software co-design,in-memory computing (IMC),microcontroller units (MCUs),neural network accelerators,tiny machine learning (TinyML)
AI 理解论文
溯源树
样例
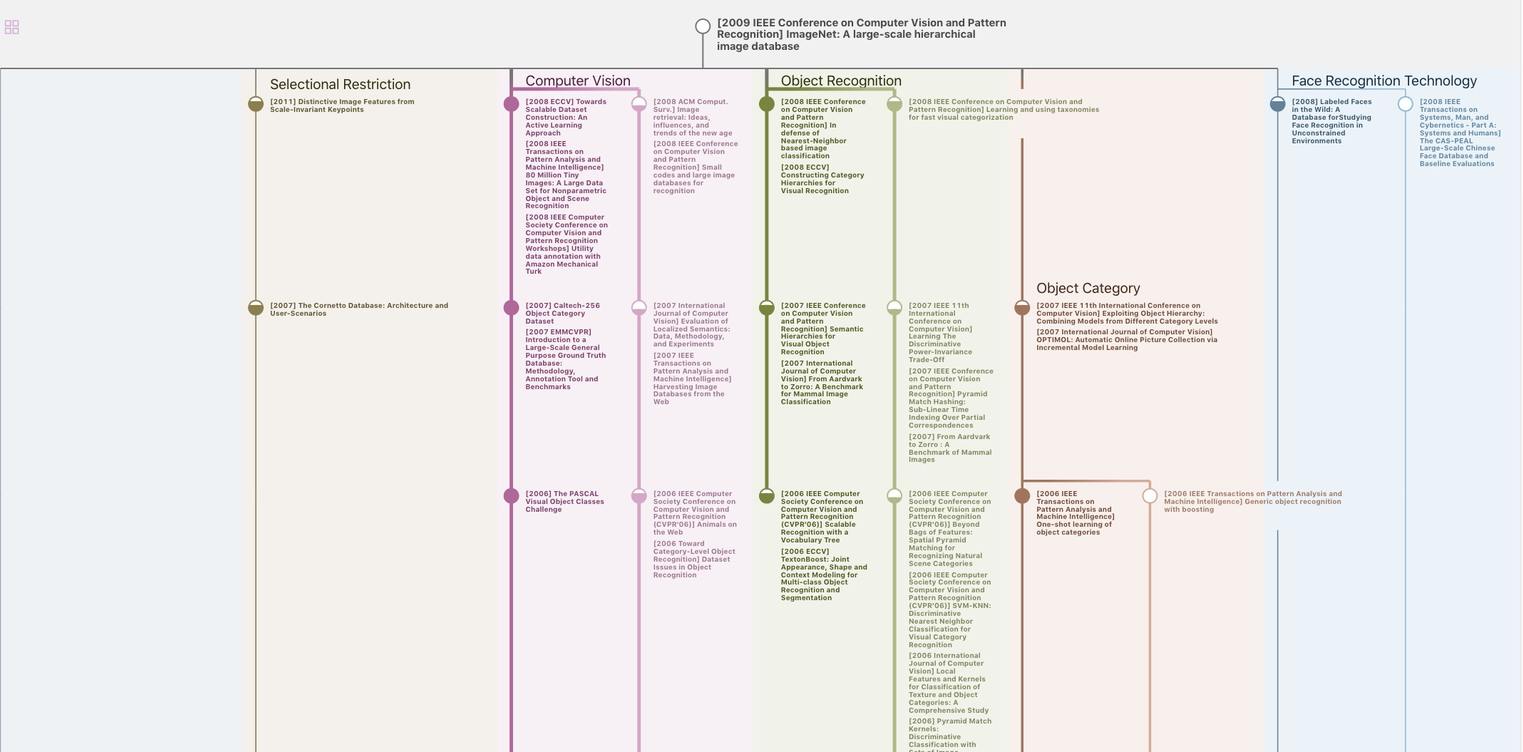
生成溯源树,研究论文发展脉络
Chat Paper
正在生成论文摘要