A 28-nm Floating-Point Computing-in-Memory Processor Using Intensive-CIM Sparse-Digital Architecture
IEEE JOURNAL OF SOLID-STATE CIRCUITS(2024)
摘要
Computing-in-memory (CIM) chips have demonstrated promising high energy efficiency on multiply-accumulate (MAC) operations for artificial intelligence (AI) applications. Though integral (INT) CIM chips are emerging, the floating-point (FP) CIM chip has not been well explored. The high-accuracy demand of larger models and complex tasks requires FP computation. Besides, most of the neural network (NN) training tasks still rely on FP computation. This work presents an energy-efficient FP CIM processor. It is observed that most of the exponent values of FP data are concentrated in a small region. Therefore, the FP computations are divided into intensive and sparse parts and then executed on an intensive-CIM sparse-digital architecture. First, an FP-to-INT CIM workflow for the intensive FP operations is designed to reduce the CIM execution cycles. Second, a flexible sparse-digital core is proposed for the remaining sparse FP operations. Utilizing both the intensive-CIM and sparse-digital cores, this work can achieve both high energy efficiency and identical accuracy to the FP algorithm baseline. Considering the FP CIM execution flow, a CIM-friendly low-bit FP training method is proposed to further reduce the execution cycles. Besides, a low-MAC-value (MACV) CIM macro is designed to utilize the more random sparsity brought by FP alignment. The 28-nm fabricated chip shows 275-1615-TOPS/W@INT4 and 17.2-91.3-TOPS/W@FP16 macro energy efficiency from dense to the average sparsity on the tested models.
更多查看译文
关键词
Computing-in-memory (CIM),floating point (FP),high energy efficiency,neural network (NN) processor,sparsity
AI 理解论文
溯源树
样例
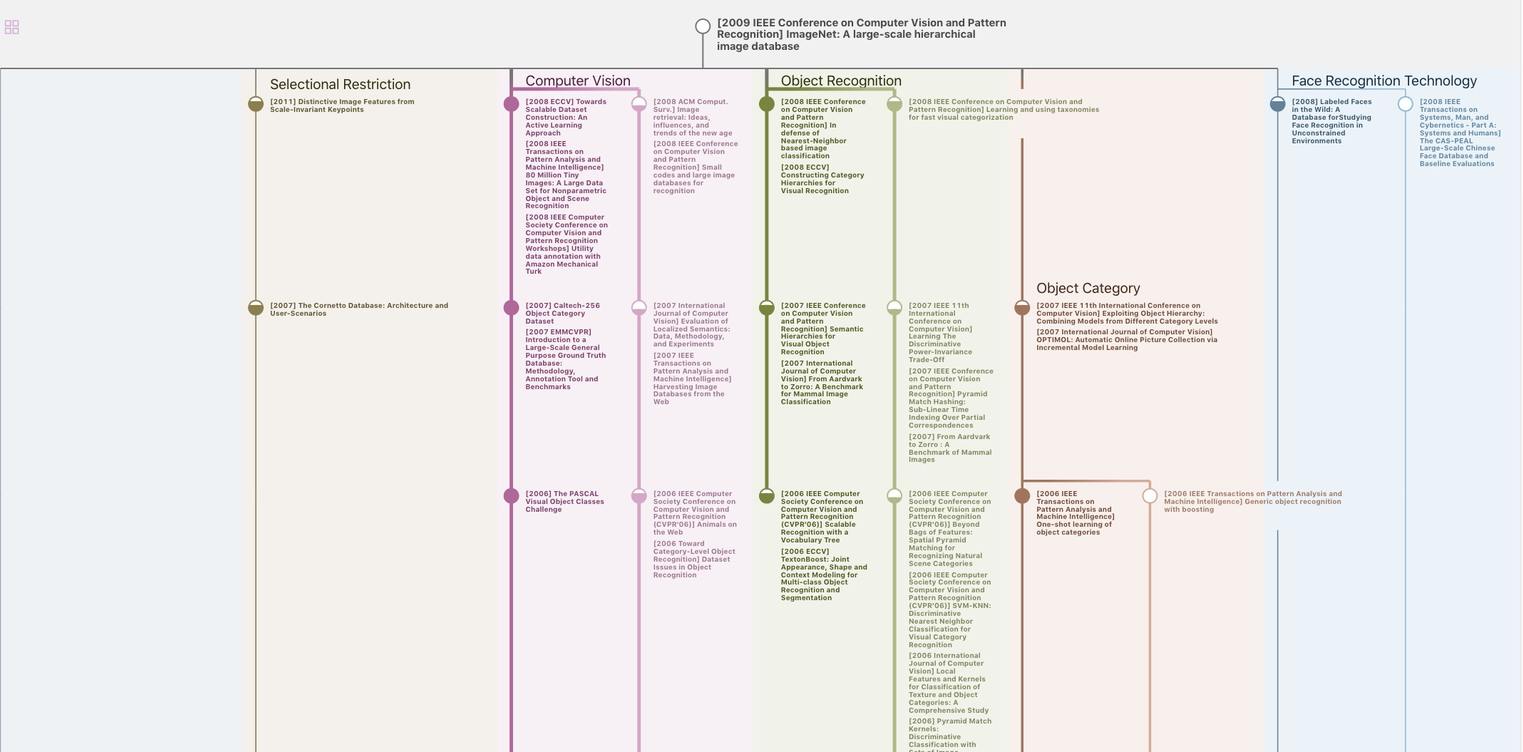
生成溯源树,研究论文发展脉络
Chat Paper
正在生成论文摘要