Testing Conditional Independence Between Latent Variables by Independence Residuals
IEEE TRANSACTIONS ON NEURAL NETWORKS AND LEARNING SYSTEMS(2024)
摘要
Conditional independence (CI) testing is an important problem, especially in causal discovery. Most testing methods assume that all variables are fully observable and then test the CI among the observed data. Such an assumption is often untenable beyond applications dealing with, e.g., psychological analysis about the mental health status and medical diagnosing (researchers need to consider the existence of latent variables in these scenarios); and typically adopted latent CI test schemes mainly suffer from robust or efficient issues. Accordingly, this article investigates the problem of testing CI between latent variables. To this end, we offer an auxiliary regression-based CI (AReCI) test by taking the measured variable as the surrogate variable of the latent variables to conduct the regression over the latent variables under the linear causal models, in which each latent variable has some certain measured variables. Specifically, given a pair of latent variables LX and LY, and a corresponding latent variable set L-O, L-X (sic) L-Y vertical bar L-O holds if and only if A{(LX)}-omega(inverted perpendicular)(1) A({LO})' and A({LY })-omega(inverted perpendicular)(2) A({LO})'' are statistically independent, where A' and A '' are the two disjoint subset of the measured variable for the corresponding latent variables, A({LO})' boolean AND A({LO})'' = empty set, and omega(1) is a parameter vector characterized from the cross covariance between A({L X}) and A({LO})', and omega(2) is a parameter vector characterized from the cross covariance between A({LY)} and A({LO})''. We theoretically show that the AReCI test is capable of addressing both Gaussian and non -Gaussian data. In addition, we find that the well-known partial correlation test can be seen as a special case of the AReCI test. Finally, we devise a causal discovery method by using the AReCI test as the CI test. The experimental results on synthetic and real-world data illustrate the effectiveness of our method.
更多查看译文
关键词
Causal discovery,conditional independence (CI) test,latent variable,latent variable model
AI 理解论文
溯源树
样例
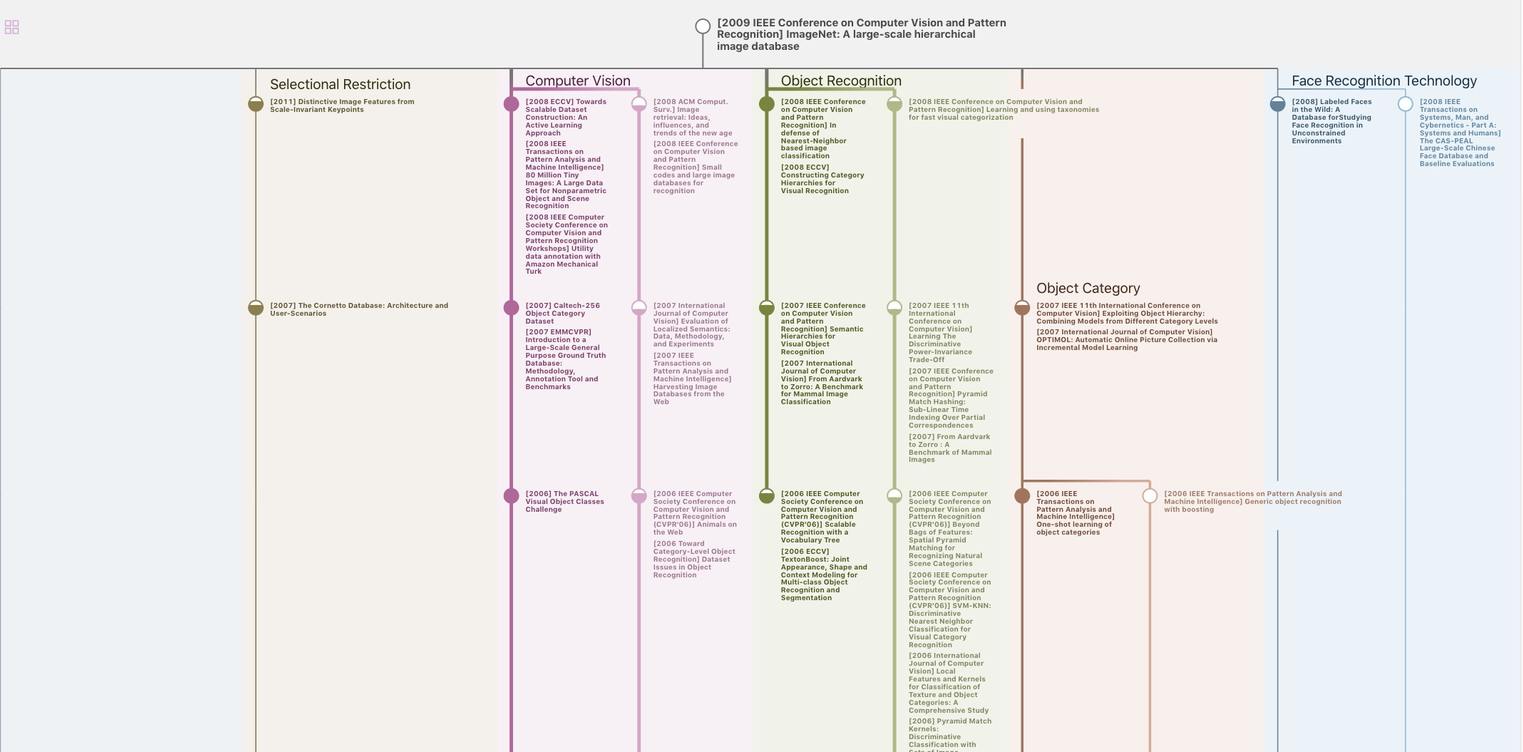
生成溯源树,研究论文发展脉络
Chat Paper
正在生成论文摘要