FairTL : A Transfer Learning Approach for Bias Mitigation in Deep Generative Models
IEEE Journal of Selected Topics in Signal Processing(2024)
摘要
This work studies fair generative models. We reveal and quantify the biases in state-of-the-art (SOTA) GANs
w.r.t.
different sensitive attributes. To address the biases, our main contribution is to propose novel methods to learn fair generative models via transfer learning. Specifically, first, we propose
FairTL
where we pre-train the generative model with a large biased dataset, then adapt the model using a small fair reference dataset. Second, to further improve sample diversity, we propose
FairTL++
, containing two additional innovations: i)
aligned feature adaptation
, which preserves learned general knowledge while improving fairness by adapting only sensitive attribute-specific parameters, ii)
multiple feedback discrimination
, which introduces a frozen discriminator for quality feedback and another evolving discriminator for fairness feedback. Taking one step further, we consider an alternative challenging and practical setup. Here, only a pre-trained model is available but the dataset used to pre-train the model is inaccessible. We remark that previous work requires access to large, biased datasets and cannot handle this setup. Extensive experimental results show that
FairTL
and
FairTL++
achieve state-of-the-art performance in quality, diversity and fairness in both setups. Code is available at:
https://github.com/sutd-visual-computing-group/FairTL
.
更多查看译文
关键词
Fairness,Generative Models,Transfer Learning,Generative Adversarial Networks
AI 理解论文
溯源树
样例
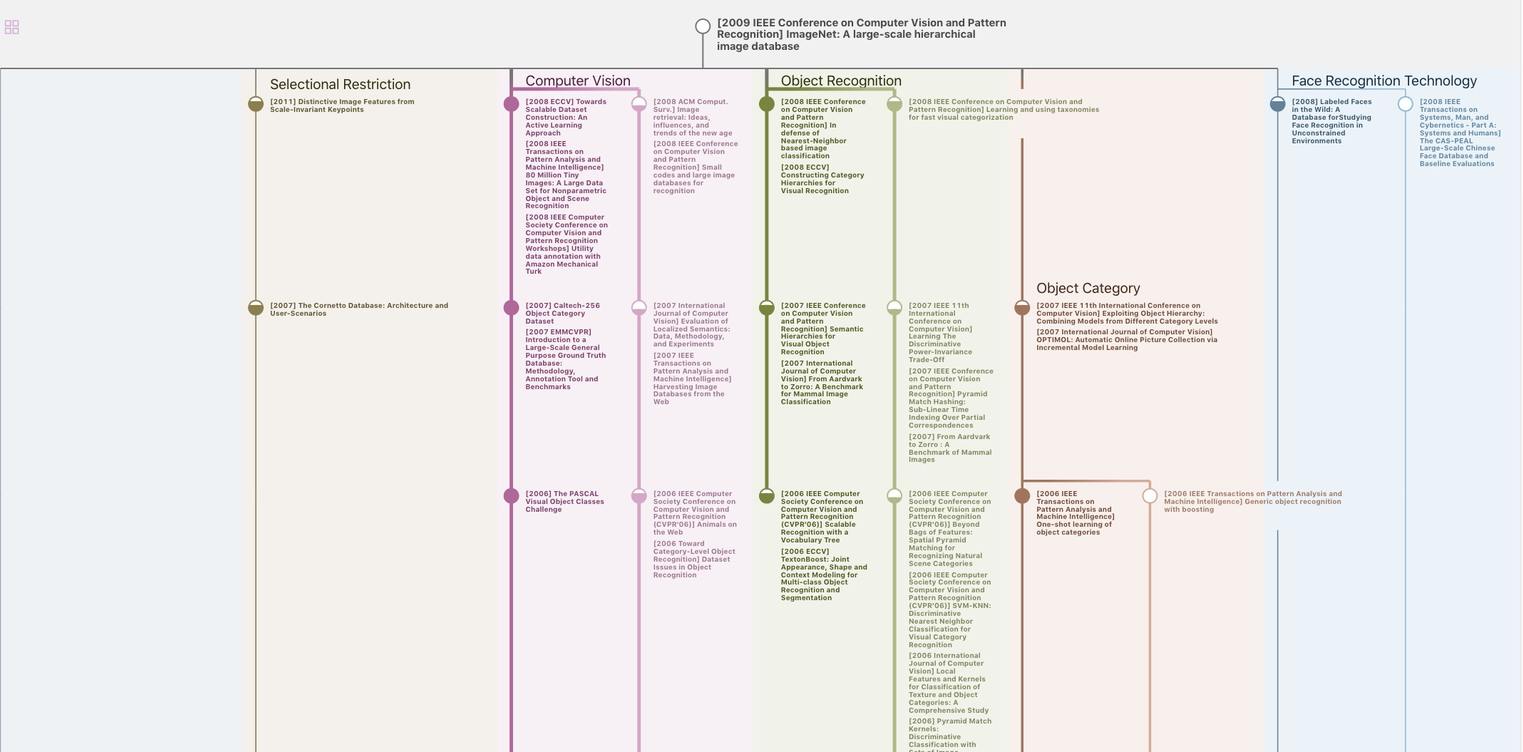
生成溯源树,研究论文发展脉络
Chat Paper
正在生成论文摘要