Tactile Object Property Recognition Using Geometrical Graph Edge Features and Multi-Thread Graph Convolutional Network.
IEEE Robotics Autom. Lett.(2024)
摘要
Performing dexterous tasks with multi-fingered hand is still a challenge. Tactile sensors can provide touch states and the object features during in-hand manipulation. However, even with such rich data of touch states, achieving dexterous multi-fingered tasks is further complicated because of the underlying complexities owing to irregularities in shapes, sizes, textures, deformabilities and mass of miscellaneous objects involved, which makes the task-conditions too diverse. Even deep learning methods struggle to handle and learn such non-linear data. In spite of the stated challenges, humans achieve these difficult tasks owing to their ability to produce a sensory-motor representation of their body. This combined representation of tactile and proprioceptive sense enables humans to be accommodative towards the diversity of objects involved in dexterous tasks. By referring to this idea, this paper presents a method for object property recognition based on Graph Convolutional Networks (GCNs). The method utilizes robot hand proprioception and morphology, with spatial embeddings derived from geometrical graph edge features acquired from real tactile sensor alignments on an Allegro Hand. We also introduce a Multi-Thread GCN (MT-GCN) architecture to process these edge features and basically multi-modal data in a graph. Distributed across the fingertips, finger phalanges, and palm of an Allegro Hand, the tri-axial tactile sensors produced a total of 1152 tactile measurements. Training data was acquired using a data-glove to directly transfer human dexterous manipulation to the robot hand. The MT-GCN with tactile and edge features achieved high recognition rate, 86.08% for 6 classes of object property combinations from 8 objects. The effect of adjacency on the network was also investigated and the trend of accuracy with respect to edge connections and edge density was observed. The proposed network showed clusters following the robot hand configuration with t-SNE analysis. Furthermore, analysis of learned parameters of edge-feature encoder showed that it can learn positions of joints on the hand, thus acquiring proprioceptive features of the hand. From these results, we could confirm that the graph edge features acquired by real robotic configuration and the MT-GCN architecture were effective for multi-fingered dexterous tasks.
更多查看译文
AI 理解论文
溯源树
样例
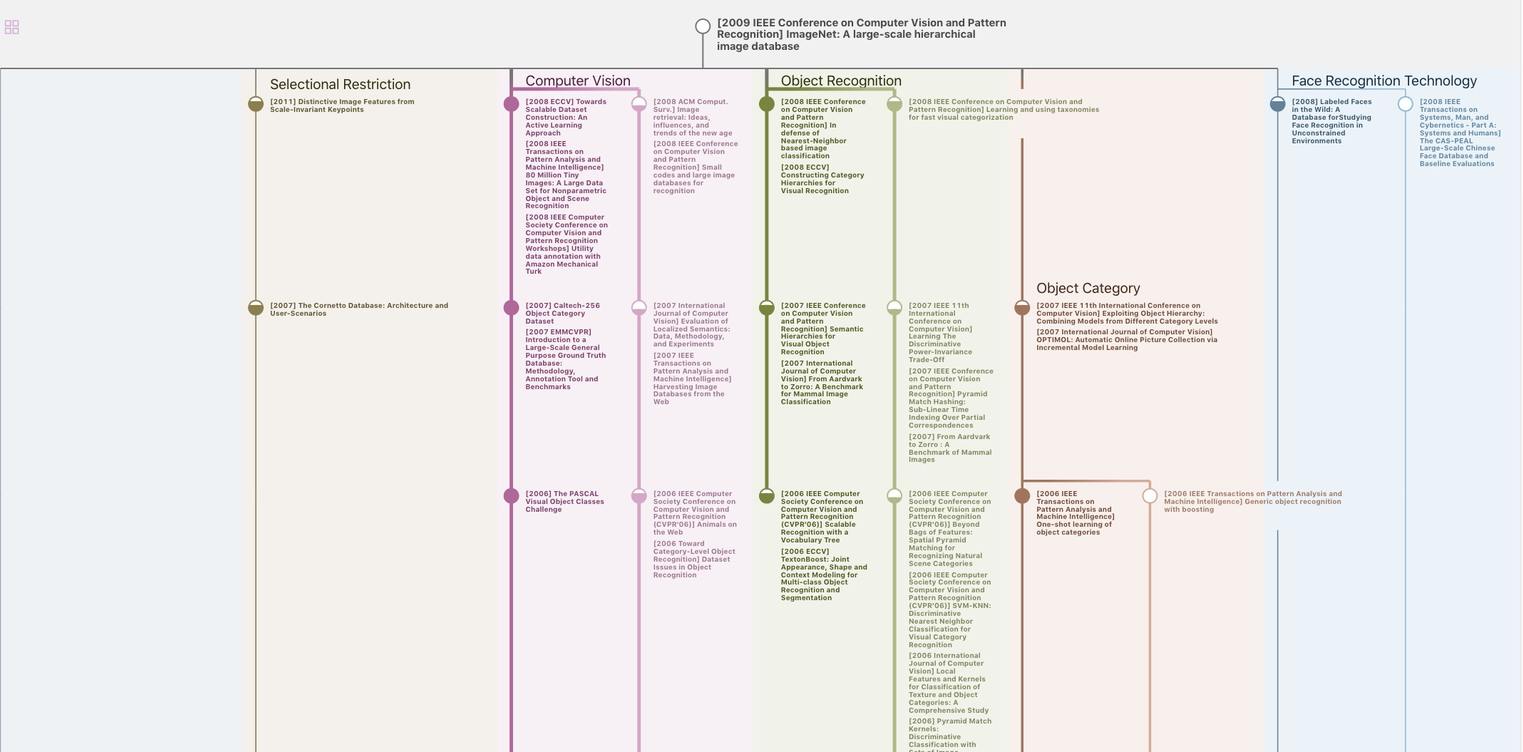
生成溯源树,研究论文发展脉络
Chat Paper
正在生成论文摘要