SACNet: A Scattered Attention-Based Network With Feature Compensator for Visual Localization.
IEEE Robotics Autom. Lett.(2024)
摘要
Visual localization, an integral component of a vast array of computer applications, has been effectively resolved by scene coordinate regression (SCoRe) methods. However, due to the limited receptive field of convolutional neural networks (CNNs), current SCoRe methods have difficulty in distinguishing comparable image patches in sparse texture scenes, thus impairing localization performance. Recently, Transformer exhibits remarkable capability in modelling long-range dependencies, which provides a remedy to the aforementioned problem. Whereas the Transformer alleviates the deficiencies of CNNs, the quadratically computational cost of Transformer leaves it incapable of handling intensive regression tasks, such as scene coordinates prediction. Towards this end, we introduce SACNet, a sparse attention-based network for efficient and accurate visual localization. We overhaul the core designs of vanilla Transformer and further propose a multiple scattered Transformer (MST) with linear complexity. MST consists of a multiple scattered attention (MSA) layer and a filtered feed-forward network (F-FFN). The MSA layer calculates the attention matrix along the channel dimension and adaptively retains the most profitable attention values for feature consolidation such that the consolidated features can better foster scene coordinate regression. F-FFN utilizes a gate mechanism that suppresses less pertinent features, where multi-scale depth-wise convolutions are further used to promote the information flow. After MST, SACNet develops a feature compensator (FC) that combines local geometry features with global context information to predict element-wise soft attention mask, thus enabling the network to adaptively reconcile the importance of local and global-aware local features. Extensive experimental results demonstrate that SACNet noticeably surpasses the cutting-edge methods on several datasets.
更多查看译文
关键词
Visual localization,deep learning,Transformer
AI 理解论文
溯源树
样例
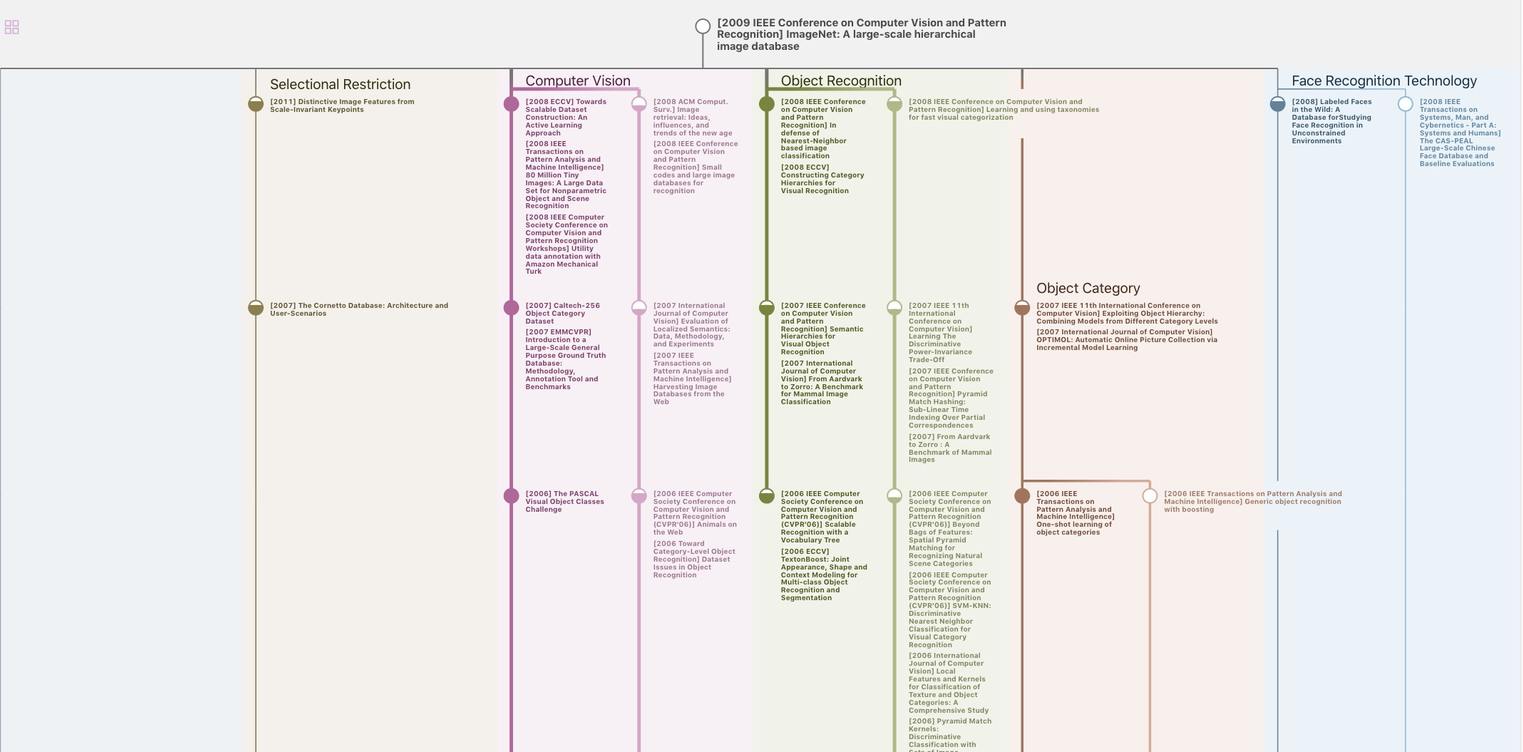
生成溯源树,研究论文发展脉络
Chat Paper
正在生成论文摘要