PTT: Piecewise Transformation Technique for Analyzing Numerical Data under Local Differential Privacy
IEEE Transactions on Mobile Computing(2024)
摘要
Local differential privacy (LDP for short), as an emerging standard privacy-preserving technique that is suitable for privacy preserving data analysis, has been widely deployed into various real-world scenarios to analyze massive data. In this study, we are mainly concerned with piecewise transformation technique (PTT for short) for analyzing numerical data under LDP. We first provide the principled framework for PTT in the context of LDP. Then, we show that (1) many PTTs are asymptotically optimal when used to obtain an unbiased estimator for mean of numerical data, and (2) there is PTT that reaches the theoretical lower bound on variance. Next, we prove that (1) there does not exist strictly better PTT than the well-used Duchi's scheme in terms of the consistency-noisy-variance, also, the latter is not always better than arbitrary PTT, (2) however, one has the ability to find a great number of PTTs that are consistently better than the latter according to the worst-case-noisy-variance. When we are restricted to the high privacy level
$\epsilon \in (0,1]$
, enough PTTs turn out to have smaller variance than the well-known Laplace mechanism. Lastly, we demonstrate that for a family of PTTs, the correspondingly theoretical lower bound of noisy variance follows
$O(\epsilon ^{-2})$
when considering
$\epsilon \in (0,1]$
.
更多查看译文
关键词
Privacy preserving data analysis,Piecewise Transformation Technique,Local differential private mechanism,Optimization,Variance
AI 理解论文
溯源树
样例
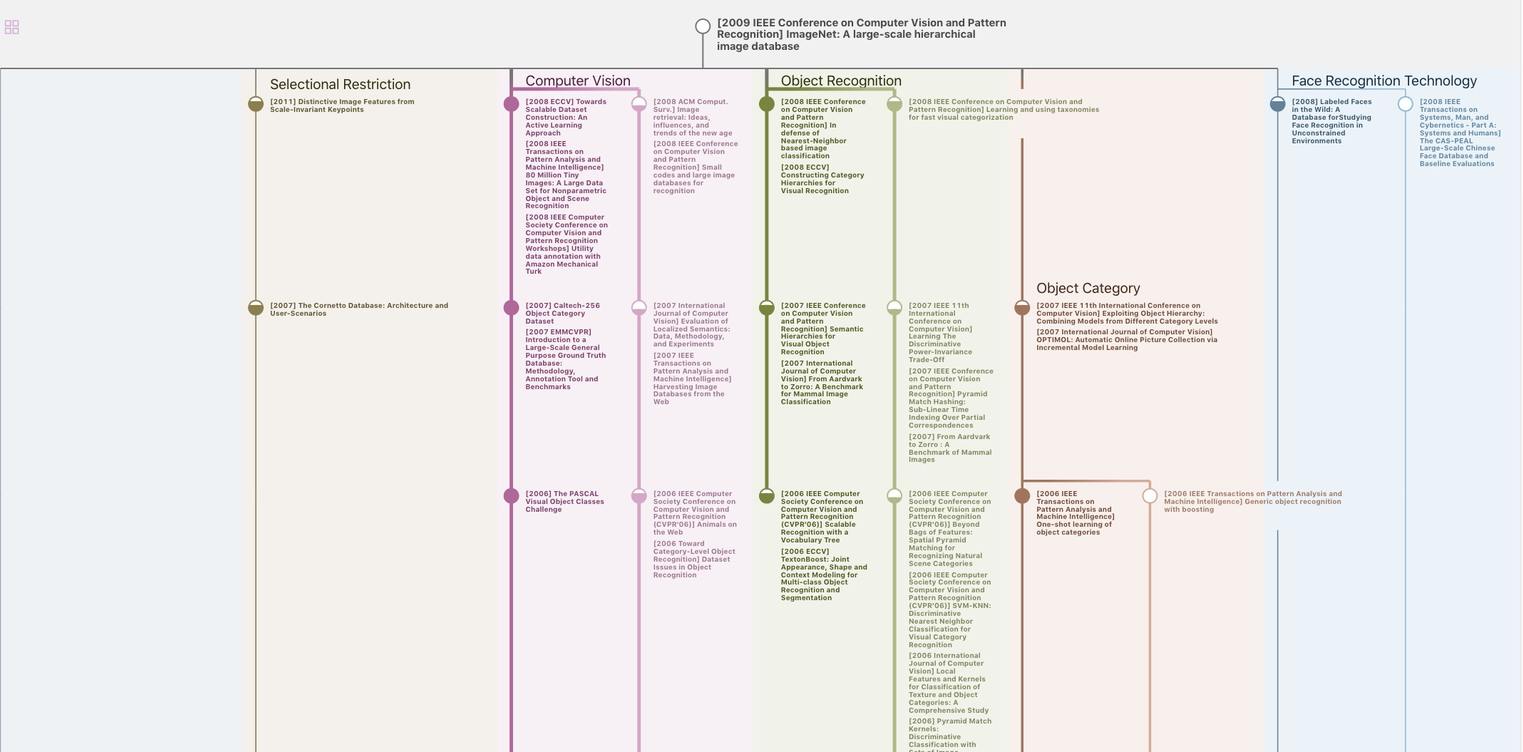
生成溯源树,研究论文发展脉络
Chat Paper
正在生成论文摘要