Deep Rank-N Decomposition Network for Image Fusion
IEEE Transactions on Multimedia(2024)
摘要
Existing deep neural network (DNN)-based image fusion methods seldom consider low-rank priors for the decomposition of source images, which cannot efficiently model base and detail components in images. To exploit the low-rank priors better, we propose a deep rank-
N
decomposition network (DRDec-Net) according to the rank-
N
decomposition of source images. Specifically, a rank-
N
decomposition model is first established by imposing low-rank priors on the base component of source images. Then, based on the decomposition model, we construct DRDec-Net, which is composed of low-rank decomposition (LRD) modules, a detail fusion (DetailF) module, and a low-rank fusion (LRF) module. In DRDec-Net, it is assumed that source images share the same base component, which is expressed as the sum of rank-1 components. We employ
N
cascaded LRD modules to extract these rank-1 components from source images. Meanwhile, detail components are obtained by subtracting the base component from source images. Next, the extracted rank-1 components and detail components are integrated by LRF and DetailF modules to produce the base component and detail component of the fused image. Finally, the sum of the two obtained components is regarded as the fused image. Compared to some state-of-the-art methods, experimental results demonstrate that the proposed DRDec-Net can produce a better performance on three image fusion tasks, including infrared and visible images, multi-exposure images, and multi-focus images.
更多查看译文
关键词
Image fusion,low-rank priors,rank-N decomposition,rank-1 component,decomposition network
AI 理解论文
溯源树
样例
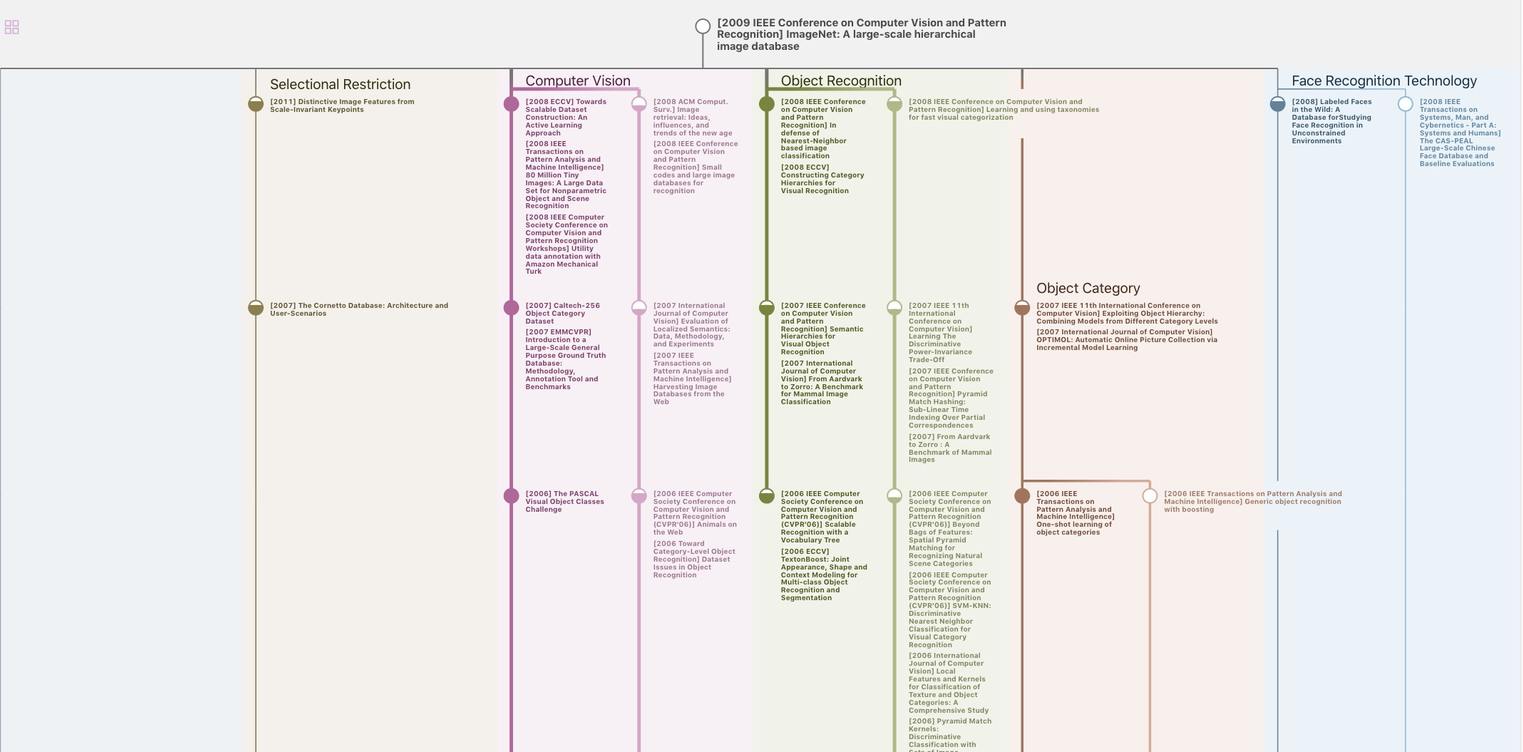
生成溯源树,研究论文发展脉络
Chat Paper
正在生成论文摘要