Reinforcement Learning Based Adaptive BitRate Caching at MEC Server
IEEE Transactions on Network and Service Management(2024)
摘要
Mobile Edge Computing (MEC) has become an important concept in modern video communication and broadcasting scenarios to address varied user expectations in an ever-evolving network environment. It has been observed that by preventing redundant access to the Origin Server through backhaul links, caching popular video content in MEC servers minimizes network congestion. Designing an efficient caching mechanism in the MEC server is challenging. To maintain a decent QoE (Quality of Experience) for end-users, we must consider diverse parameters like content popularity, network conditions, etc. In this work, we have proposed a QoE-aware Adaptive BitRate (ABR) caching mechanism at the MEC server using Reinforcement Learning (RL). The proposed model predicts the content popularity of the video and the most preferred video quality for the end-users of a Base Station. In this work, an efficient caching mechanism is devised in the MEC server to provide a decent QoE among the end-users. The primary goal of our RL-based framework is to increase the cache hit rate and reduce the backhaul load while maintaining a satisfactory QoE. Experimental results demonstrate that the proposed model, which emphasizes on video quality, video quality switching, and cache hit rate, outperforms state-of-the-art caching algorithms in terms of the overall QoE reward.
更多查看译文
关键词
Mobile Edge Computing,Adaptive Bitrate Streaming,Reinforcement Learning,Content Caching,Content Popularity
AI 理解论文
溯源树
样例
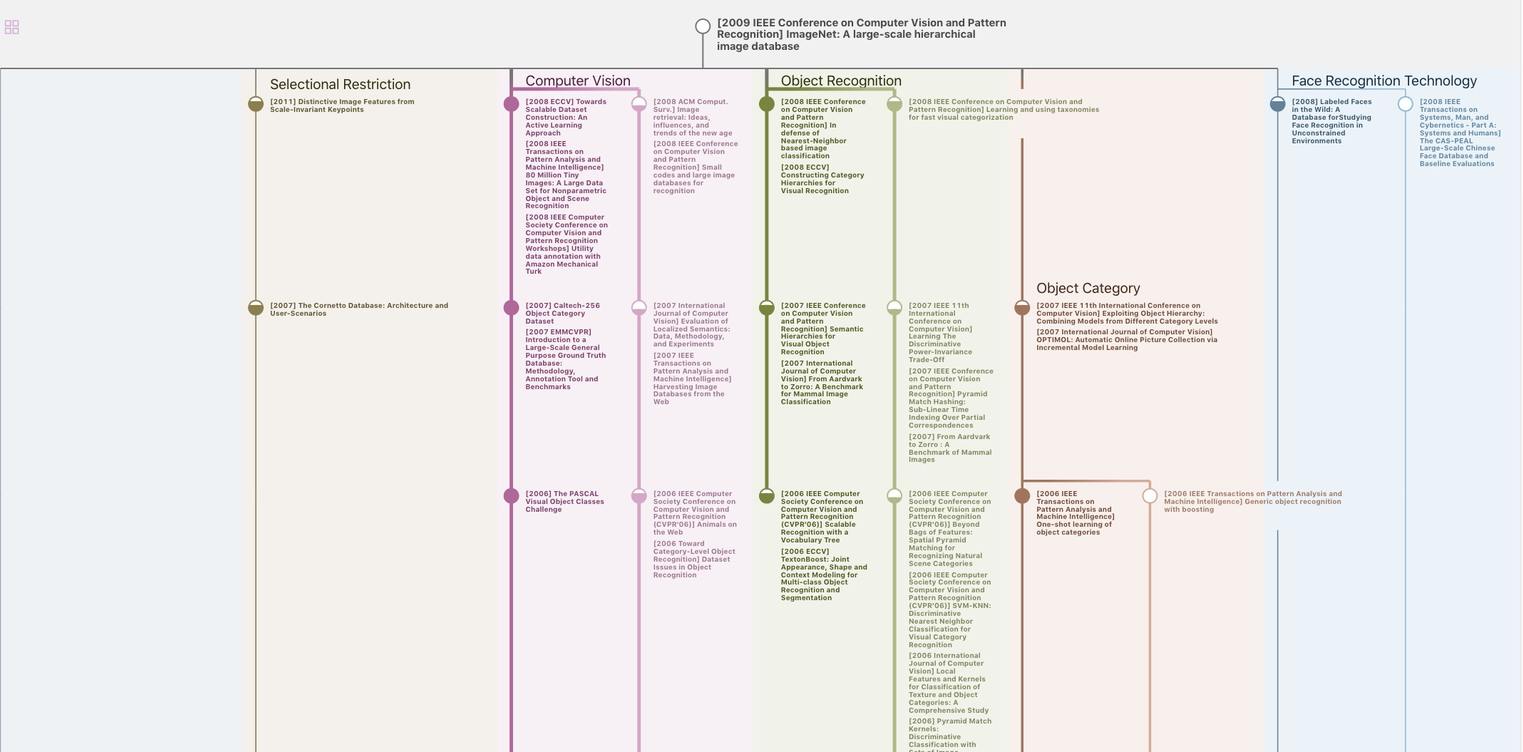
生成溯源树,研究论文发展脉络
Chat Paper
正在生成论文摘要