DCMSTRD: End-to-end Dense Captioning via Multi-Scale Transformer Decoding
IEEE Transactions on Multimedia(2024)
摘要
Dense captioning creates diverse Region of Interests (RoIs) descriptions for complex visual scenes. While promising results have been obtained, several issues persist. In particular: 1) it is hard to find the optimal parameters for artificially designed modules (e.g., non-maximum suppression (NMS)) causing redundancies and fewer interactions to benefit the two sub-tasks of RoI detection and RoI captioning; 2) the absence of a multi-scale decoder in current methods hinders the acquisition of scale-invariant features, thus leading to poor performance. To tackle these limitations, we bypass the artificially designed modules and present an end-to-end dense captioning framework via multi-scale transformer decoding (DCMSTRD). DCMSTRD solves dense captioning by set matching and prediction instead. To further enhance the discriminative quality of the multi-scale representations during caption generation, we introduce a multi-scale module, termed multi-scale language decoder (MSLD). Our proposed method tested on standard datasets achieves a mean Average Precision (mAP) of 16.7% on the challenging VG-COCO dataset, demonstrating its effectiveness against the current methods.
更多查看译文
关键词
Dense Captioning,Artificially Designed Modules,End-to-end Dense Captioning framework via Multi-Scale Transformer Decoding (DCMSTRD),Multi-Scale Language Decoder (MSLD)
AI 理解论文
溯源树
样例
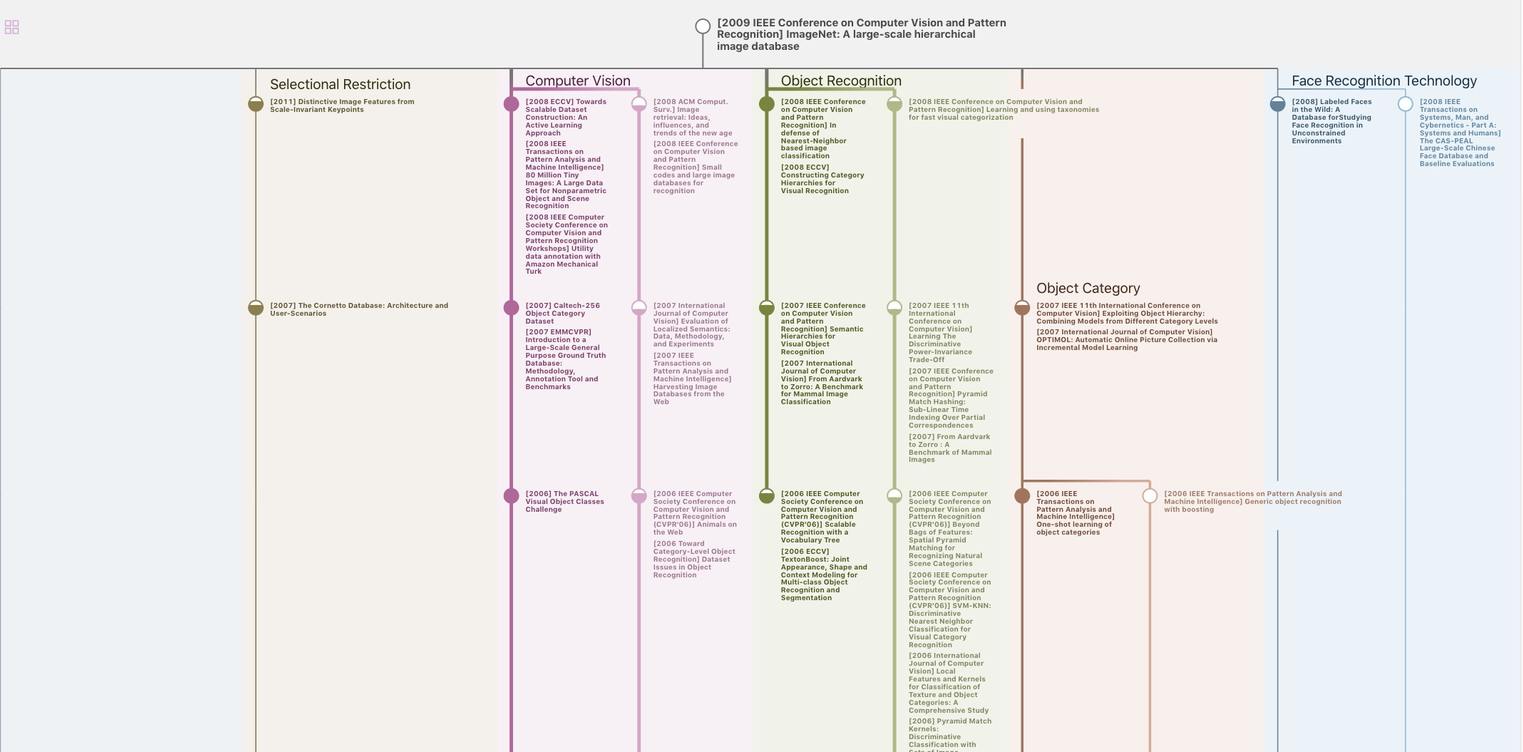
生成溯源树,研究论文发展脉络
Chat Paper
正在生成论文摘要