Self-Mining the Confident Prototypes for Source-Free Unsupervised Domain Adaptation in Image Segmentation
IEEE Transactions on Multimedia(2024)
摘要
This paper studies a practical Source-free unsupervised domain adaptation (SFUDA) problem, which transfers knowledge of source-trained models to the target domain, without accessing the source data. It has received increasing attention in recent years, while the prior arts focus on designing adaptation strategies, ignoring that different target samples exhibit different transfer abilities on the source model. Additionally, we observe pixel-wise class prediction is typically accompanied by ambiguity issue, i.e., prediction errors often occur between several confusing classes. In this study, we propose a dual-branch collaborative learning framework that aims to achieve reliable knowledge transfer from important samples to the rest by fully mining confident prototypes in the target data. Concretely, we first partition the target data into confident samples and uncertain samples via a new class-ranking reliability score and then utilize the latent features from the confident branch as guidance to promote the learning of the uncertain branch. For ambiguity issue, we propose a feature relabelling module, which exploits reliable prototypes in the mini-batch as well as in the target data to refine labels of uncertain features. We further deploy the proposed framework to commonly used CNN and state-of-the-art Transformer architectures and reveal the potential to promote the generalization ability of backbone models. Experimental results on both natural and medical benchmark datasets verify that our proposed approach exceeds state-of-the-art SFUDA methods with large margins, and achieves comparable performance to existing UDA methods.
更多查看译文
关键词
Source-free unsupervised domain adaptation,image segmentation
AI 理解论文
溯源树
样例
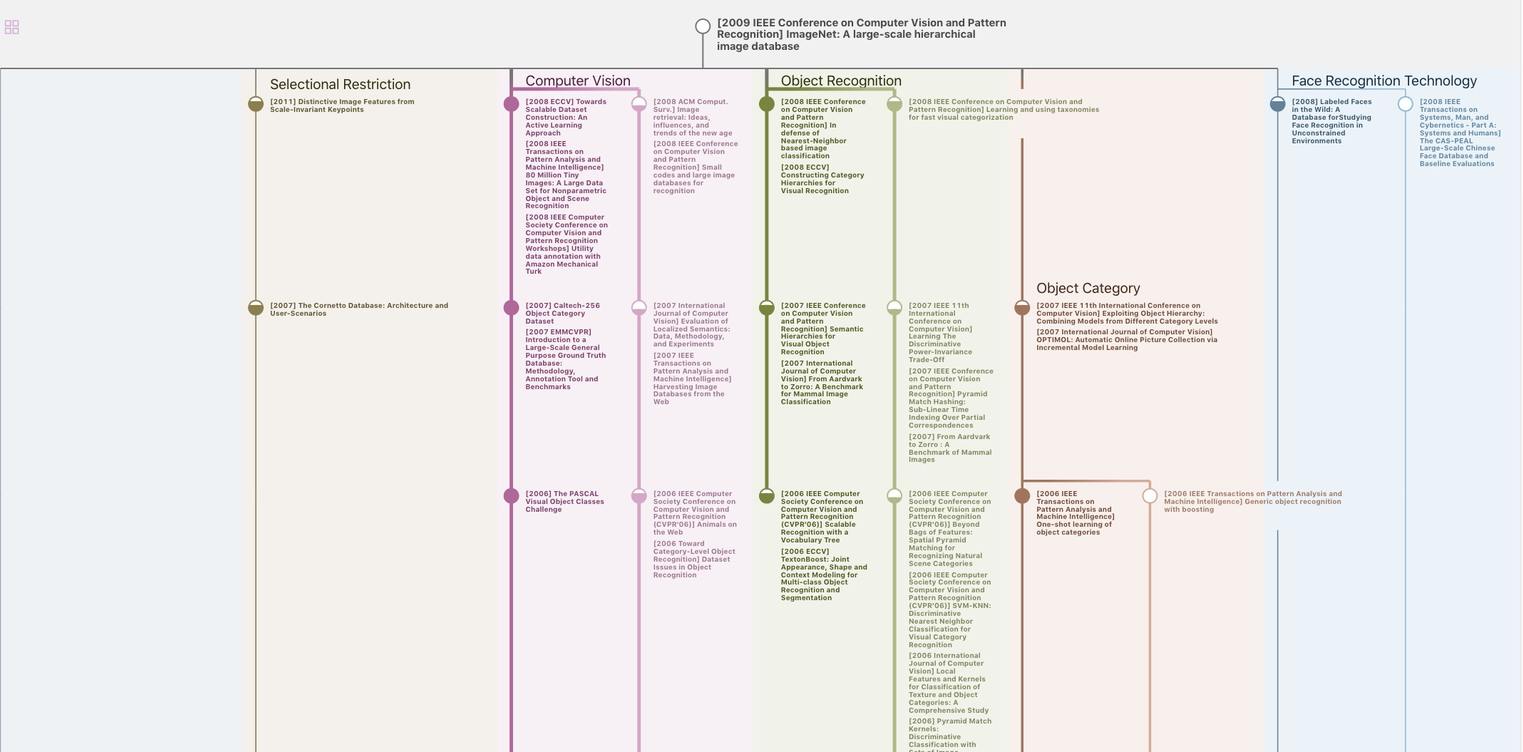
生成溯源树,研究论文发展脉络
Chat Paper
正在生成论文摘要