Text2Reaction : Enabling Reactive Task Planning Using Large Language Models
IEEE Robotics and Automation Letters(2024)
摘要
To complete tasks in dynamic environments, robots need to timely update their plans to react to environment changes. Traditional stripe-like or learning-based planners struggle to achieve this due to their high reliance on meticulously predefined planning rules or labeled data. Fortunately, recent works find that Large Language Models (LLMs) can be effectively prompted to solve planning problems. Thus, we investigate the strategies for LLMs to master reactive planning problems without complex definitions and extra training. We propose Text2Reaction, an LLM-based framework enabling robots to continuously reason and update plans according to the latest environment changes. Inspired from human's step-by-step re-planning process, we present the Re-planning Prompt, which informs LLMs the basic principles of re-planning and fosters the gradual development of a current plan to a new one in a three-hop reasoning manner - cause analysis, consequence inference, and plan adjustment. Additionally, Text2Reaction is designed to first generate an initial plan based on the task description before execution, allowing for subsequent iterative updates of this plan. We demonstrate the superior performance of Text2Reaction over prior works in reacting to various environment changes and completing varied tasks. Additionally, we validate the reliability of our re-planning prompt through ablation experiments and its capability when deployed in real-world robots, enabling continuous reasoning in the face of diverse changes until the user instructions are successfully completed.
更多查看译文
关键词
Planning under Uncertainty,AI-Based Methods,Learning from Demonstration
AI 理解论文
溯源树
样例
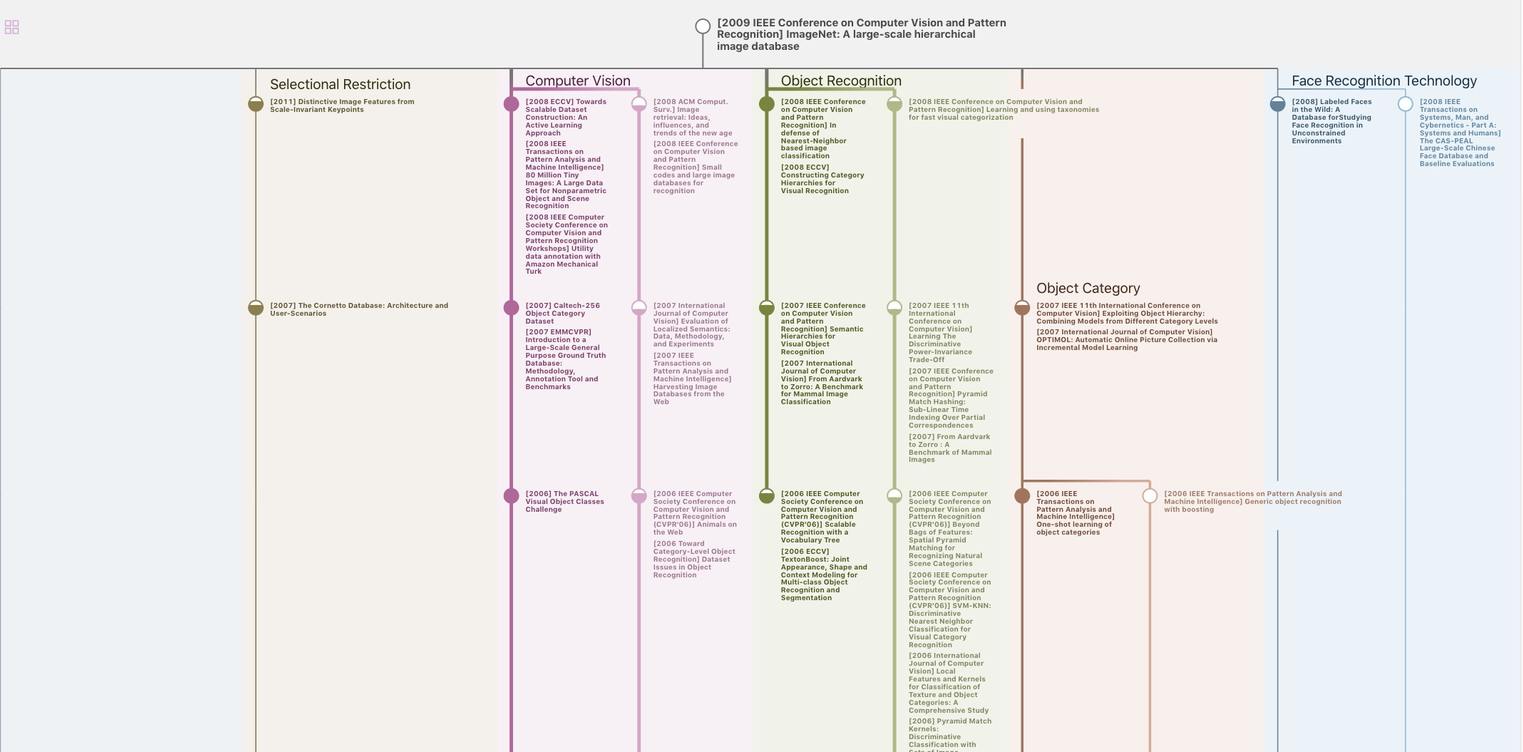
生成溯源树,研究论文发展脉络
Chat Paper
正在生成论文摘要