Depriving the Survival Space of Adversaries Against Poisoned Gradients in Federated Learning
IEEE Transactions on Information Forensics and Security(2024)
摘要
Federated learning (FL) allows clients at the edge to learn a shared global model without disclosing their private data. However, FL is susceptible to poisoning attacks, wherein an adversary injects tainted local models that ultimately corrupt the global model. Despite various defensive mechanisms having been developed to combat poisoning attacks, they all fall short of securing practical FL scenarios with heterogeneous and unbalanced data distribution. Moreover, the cutting-edge defenses currently at our disposal demand access to a proprietary dataset that closely mirrors the distribution of clients’ data, which runs counter to the fundamental principle of privacy protection in FL. It is still challenging to devise an effective defense approach that applies to practical FL. In this work, we strive to narrow the divide between FL defense and its practical use. We first present a general framework to comprehend the effect of poisoning attacks in FL when the training data is not independent and identically distributed (non-IID). We then HeteroFL, a novel FL scheme that incorporates four complementary defensive strategies. These tactics are implemented in succession to refine the aggregated model toward approaching the global optimum. Ultimately, we devise an adaptive attack specifically for HeteroFL, aimed at offering a more thorough evaluation of its robustness. Our extensive experiments over heterogeneous datasets and models show that HeteroFL surpasses all state-of-the-art defenses in thwarting various poisoning attacks, i.e., HeteroFL achieves global model accuracies comparable to the baseline, whereas other defenses suffer a significant accuracy reduction ranging from 34% to 79%.
更多查看译文
关键词
Federated learning,poisoning attacks,defenses
AI 理解论文
溯源树
样例
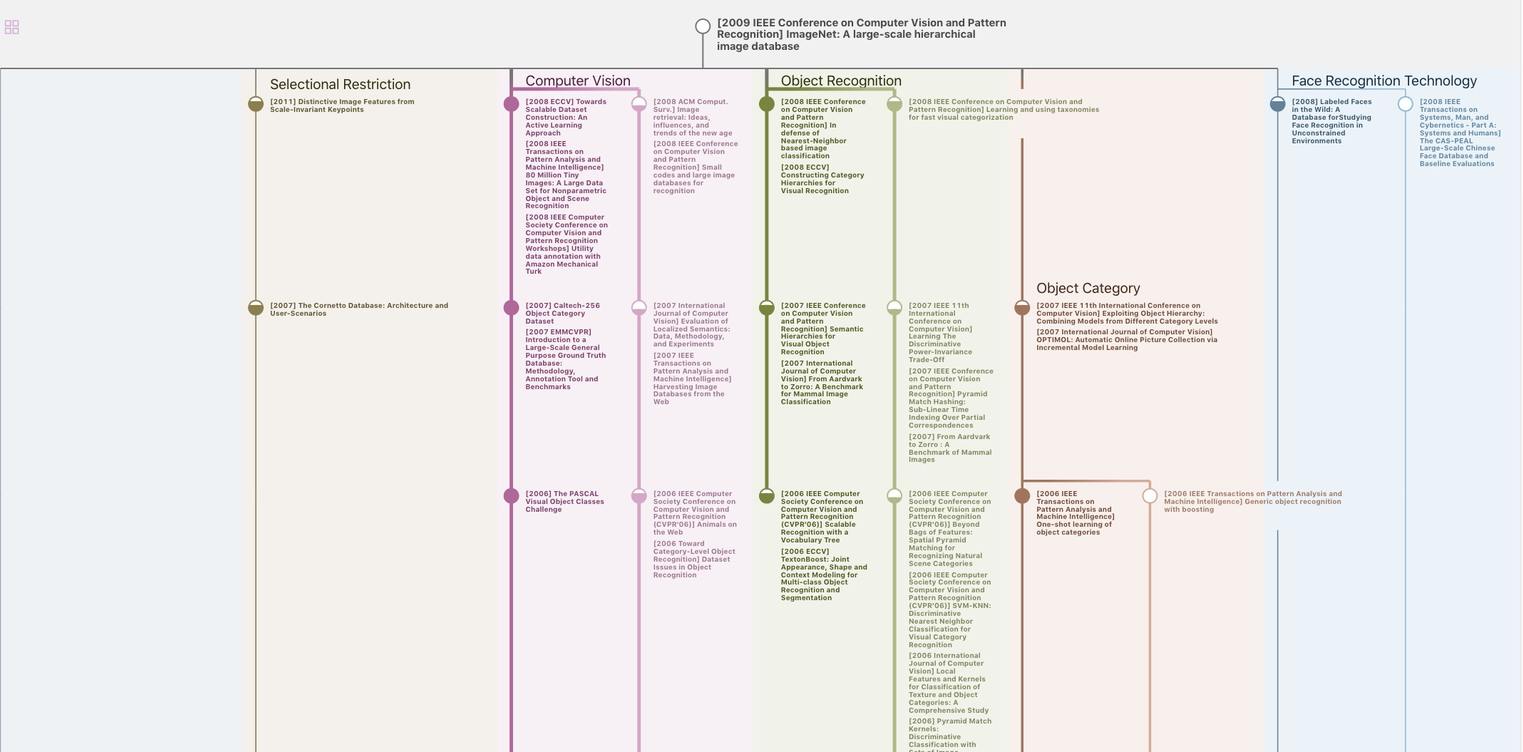
生成溯源树,研究论文发展脉络
Chat Paper
正在生成论文摘要