TPV-IGKD: Image-Guided Knowledge Distillation for 3D Semantic Segmentation With Tri-Plane-View
IEEE TRANSACTIONS ON INTELLIGENT TRANSPORTATION SYSTEMS(2024)
摘要
As 3D LiDAR point cloud and 2D images capture complementary information for autonomous driving, great efforts are made on 3D semantic segmentation using both modalities data. However, they suffer from different problems. 3D-to-2D fusion methods is difficult to determine accurate mapping relations, and the moving objects cannot be carefully considered. 2D-to-3D fusion methods need to process strictly paired data simultaneously, which is time-consuming and impractical in real-time scenarios. In this paper, a novel image-guided knowledge distillation framework based on tri-plane-view is proposed for 3D semantic segmentation. Our method has two main contributions. First, the image features are represented in an efficient 3D tri-plane-view space, which facilitates features alignment and fusion. Second, the object movements can be predicted in such a unified 3D space to fully utilize the time information. The fusion data knowledge is transferred to pure 3D network using knowledge distillation, so only the point cloud branch is needed during inference and thus achieving real-time deployment. Our method is evaluated on SemanticKITTI and nuScenes dataset as well as outdoor environments. As a result, models based on point cloud inputs are significantly improved after applying our method.
更多查看译文
关键词
Three-dimensional displays,Point cloud compression,Semantics,Laser radar,Semantic segmentation,Image sensors,History,Autonomous driving,LiDAR semantic segmentation,knowledge distillation,image guide
AI 理解论文
溯源树
样例
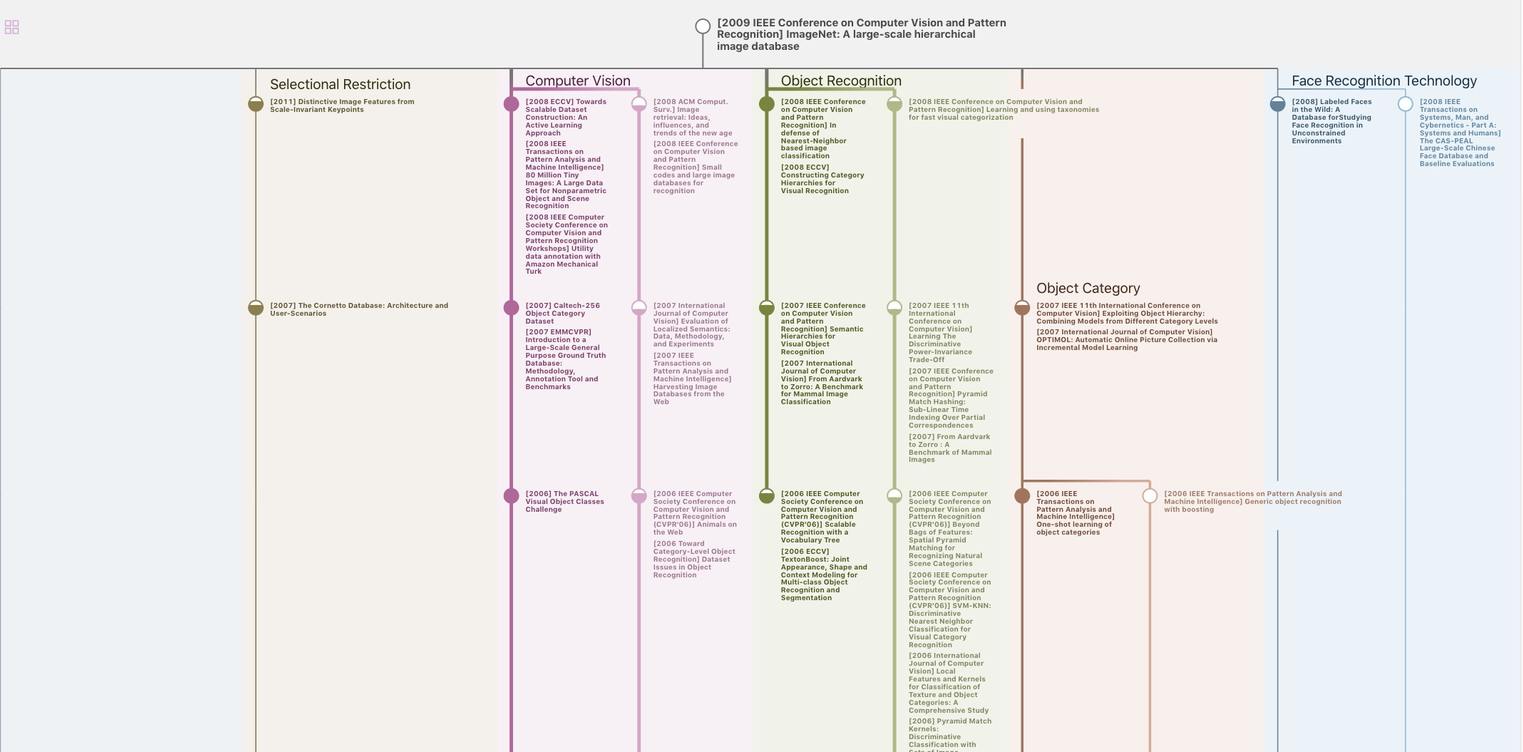
生成溯源树,研究论文发展脉络
Chat Paper
正在生成论文摘要