Spatial-Temporal Data-Driven Model for Load Altering Attack Detection in Smart Power Distribution Networks
IEEE TRANSACTIONS ON INDUSTRIAL INFORMATICS(2024)
摘要
The widespread deployment of information and communication technologies in smart power distribution networks (SPDNs) exposes them to cyber threats. Among different types of cyber-attacks in such ICT-based SPDNs, load-altering attacks (LAAs) against high-wattage devices have received significant attention in recent years. In this context, this article proposes a data-driven detection model tailored for identifying and localizing LAAs in SPDNs. In this pursuit, first, the graph structure of an SPDN, which is obtained from the grid topology, and node features, i.e., measurements of the load's power, are fed to a graph attention network (GAT), and the spatial correlations among the nodes are captured. Alongside, the temporal correlations are captured using a long short-term memory model trained based on the graph representation obtained from the GAT. These spatial and temporal correlations are used by prediction and reconstruction models, i.e., a fully connected neural network and an auto-encoder. Finally, based on the error of the prediction and reconstruction blocks, an attack score for each load is calculated, and the compromised loads are detected and localized. To evaluate the performance of the proposed model, a co-simulation framework, which simulates the power system and emulates the communication network using real industrial protocols, i.e., IEC 60870-5-104, has been developed. The robustness of the model's performance against noisy data and non-attack outliers is confirmed with respect to different noise levels and data outliers. Also, the developed model's superior performance over existing models is demonstrated through various LAA scenarios applied to the IEEE 33- and the 123-Bus benchmarks.
更多查看译文
关键词
Load modeling,Correlation,Predictive models,Power systems,Data models,Power measurement,Computational modeling,Cosimulation framework,detection and localization,load-altering attack (LAA),prediction and reconstruction models,smart power distribution network (SPDN),spatial and temporal correlation
AI 理解论文
溯源树
样例
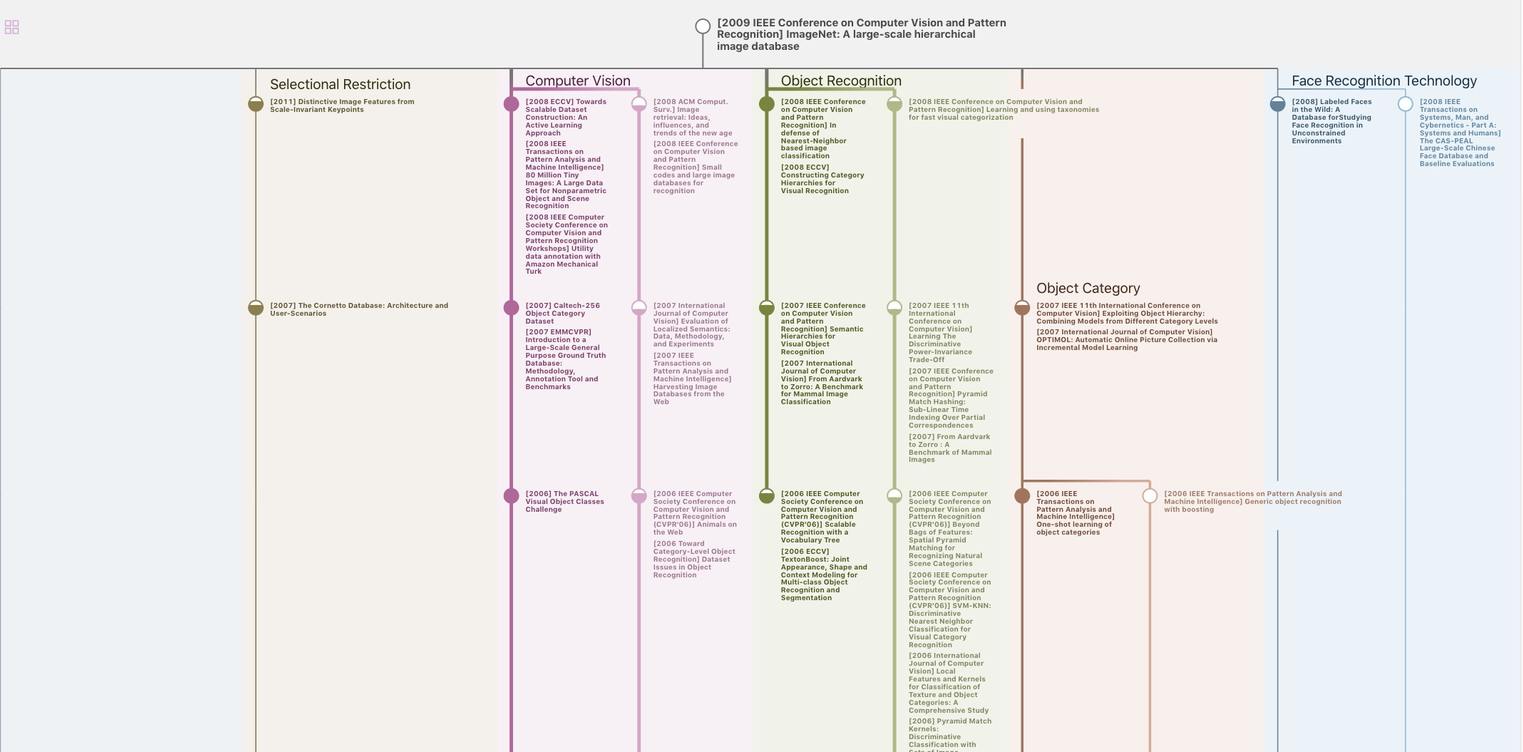
生成溯源树,研究论文发展脉络
Chat Paper
正在生成论文摘要