Information-Imbalance Learning With Hazard-Sensitive Loss for Machine Fault Diagnosis.
IEEE Trans. Instrum. Meas.(2024)
摘要
How to conduct effective machine fault diagnosis so as to avoid catastrophic accidents is an eternal theme in industrial circle. Current research focuses on the data level imbalance in practice and ignore the fact that cognition importance of various fault types is significantly imbalanced. We systematically reveal the instructive significance of cognition prior to dealing with fault classification tasks with different hazard levels. For the first time, an information-imbalance learning framework is constructed to give consideration to both data level and cognition level. Furthermore, we theoretically deduce hazard-sensitive loss to get the Bayes-optimal classifier for information-imbalance learning framework. It can effectively embed the cognition prior of various categories into the model to make it sensitive and adaptive to the degree of hazard. Moreover, it can also adapt to the application scenarios with increased data imbalance characteristics. Performance tests are carried out on the typical scenarios with differentiated hazards of aero-engines and helicopters, the results show that by using this loss function, up to 33% performance improvement of focus categories can be achieved without increasing the time complexity of the model.
更多查看译文
关键词
condition monitoring,class imbalance,cognition imbalance,fault diagnosis
AI 理解论文
溯源树
样例
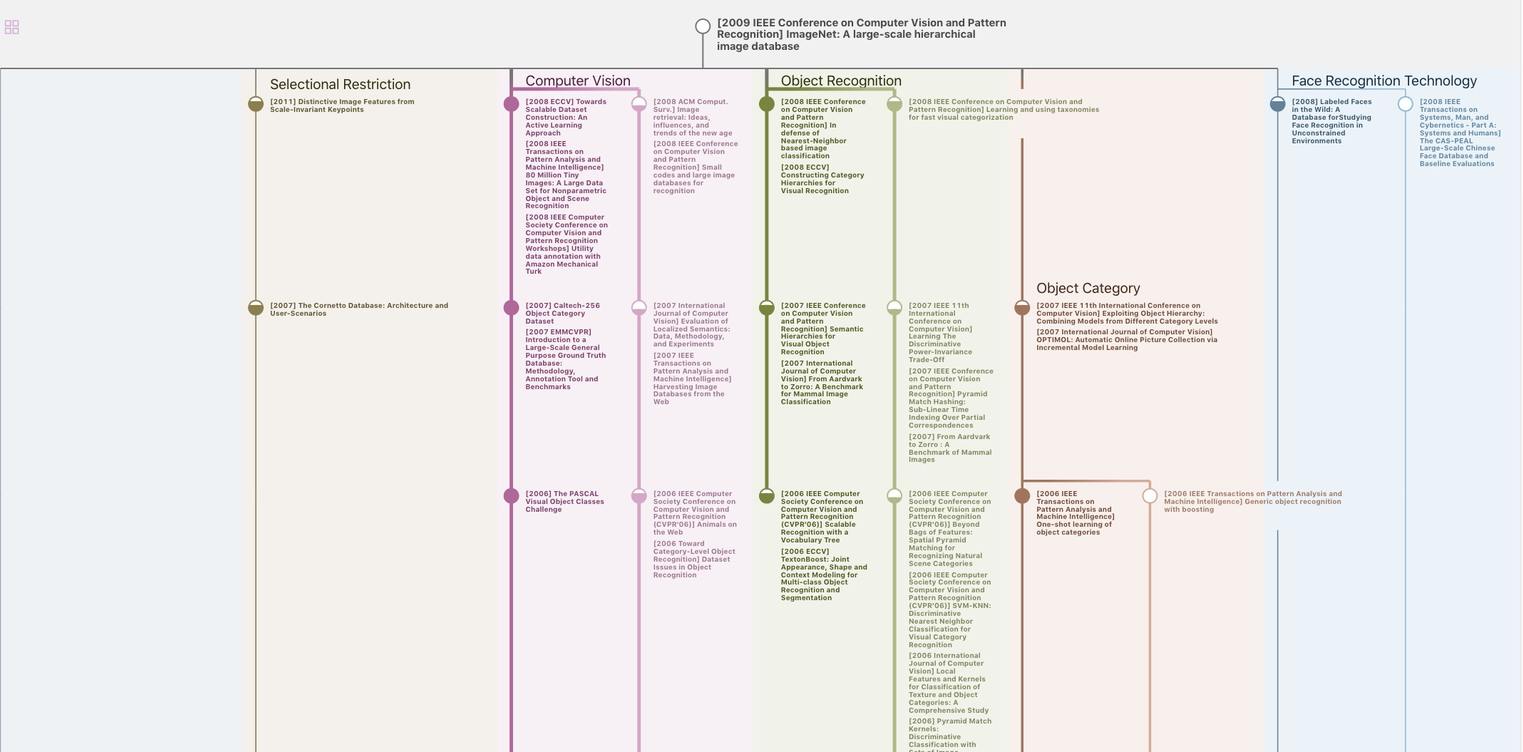
生成溯源树,研究论文发展脉络
Chat Paper
正在生成论文摘要