DMF-Net: A Dual Remote Sensing Image Fusion Network Based on Multi-Scale Convolutional Dense Connectivity with Performance Measure
IEEE Transactions on Instrumentation and Measurement(2024)
摘要
Pan-sharpening is a remote sensing image processing technique whose main objective is to generate images with high spatial and spectral resolution by combining high spatial resolution panoramic (PAN) images and low spatial resolution multispectral (MS) images, and this processing improves the detail resolution of remotely sensed images while preserving the rich spectral information. Inspired by other deep learning methods on panchromatic sharpening, we propose a dual-stream remote sensing image fusion network (DMF-Net) based on multi-scale convolution and dense connectivity, aiming to improve the spatial and spectral quality of the fused images. DMF-Net uses a novel attentional mechanism (MSKA) as well as multi-scale convolution and dense connectivity to extract the key features in the images with different resolutions, and fuses the features using a fusion module (FB), and finally generates high-resolution multispectral(HRMS) images. In our experiments, we tested the proposed method on three datasets, QuickBird, WorldView-2, and Maryland, using a variety of evaluation metrics, and achieved PSNR of 36.4065, 38.7740, and 31.1169, respectively, while our method also ranked high in terms of other metrics. The experimental results show that DMF-Net has significant advantages over existing fusion techniques in quantitative and qualitative evaluations, and can effectively reduce spectral distortion. Furthermore, by comparing with existing fusion schemes, we demonstrate the robustness and generalizability of the method.
更多查看译文
关键词
Pan-sharpening,attention mechanism,image fusion,remote sensing,deep learning,convolutional neural networks,measure
AI 理解论文
溯源树
样例
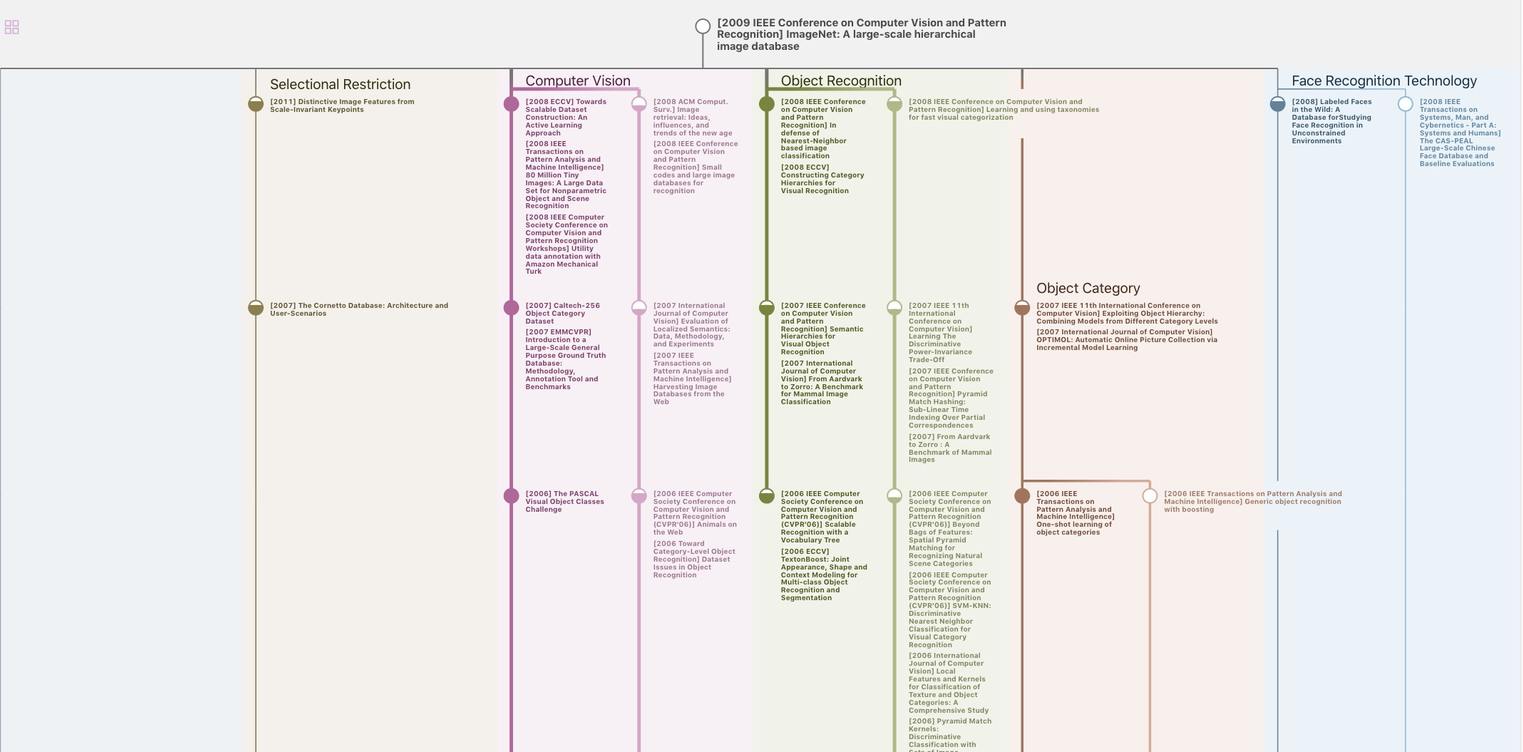
生成溯源树,研究论文发展脉络
Chat Paper
正在生成论文摘要