Short-Term Metro Origin-Destination Passenger Flow Prediction via Spatio-Temporal Dynamic Attentive Multi-Hypergraph Network
IEEE TRANSACTIONS ON INTELLIGENT TRANSPORTATION SYSTEMS(2024)
摘要
Metro bears a large number of passenger flows in urban transportation systems. Short-term metro origin-destination (OD) passenger flow prediction is an essential component of intelligent transportation systems (ITS), which allows operators to better monitor the metro system and improve the level of service for passengers. In this paper, we exploit a novel data structure, hypergraph, to represent the complex correlation between OD pairs, and propose an elaborately designed Spatio-Temporal Dynamic Attentive Multi-HyperGraph Network (ST-DAMHGN) to tackle the short-term OD passenger flow prediction problem. In the proposed framework, we construct multiple hypergraphs to model the relationship between OD pairs and adopt the perceptual field to realize efficient and effective vertex feature extraction. Then, we utilize the attention mechanism to adaptively and dynamically synthesize information from multiple hypergraphs and make a trade-off between exploration and exploitation. A case study is conducted on the metro system of Hangzhou, China. The results of extensive experiments show that ST-DAMHGN outperforms baseline models. The efficiency is validated for the multi-hypergraph model, perceptual field, spatial feature extraction, and attention mechanism. The hypergraph structure used in our model is verified suitable for modeling traffic data without physical road networks to map directly, e.g., OD passenger flow. ST-DAMHGN can be widely implemented by defining proper correlations for model relationships.
更多查看译文
关键词
Metro OD passenger flow prediction,multi-graph structure,dynamic attentive neural network,hypergraph convolutional network
AI 理解论文
溯源树
样例
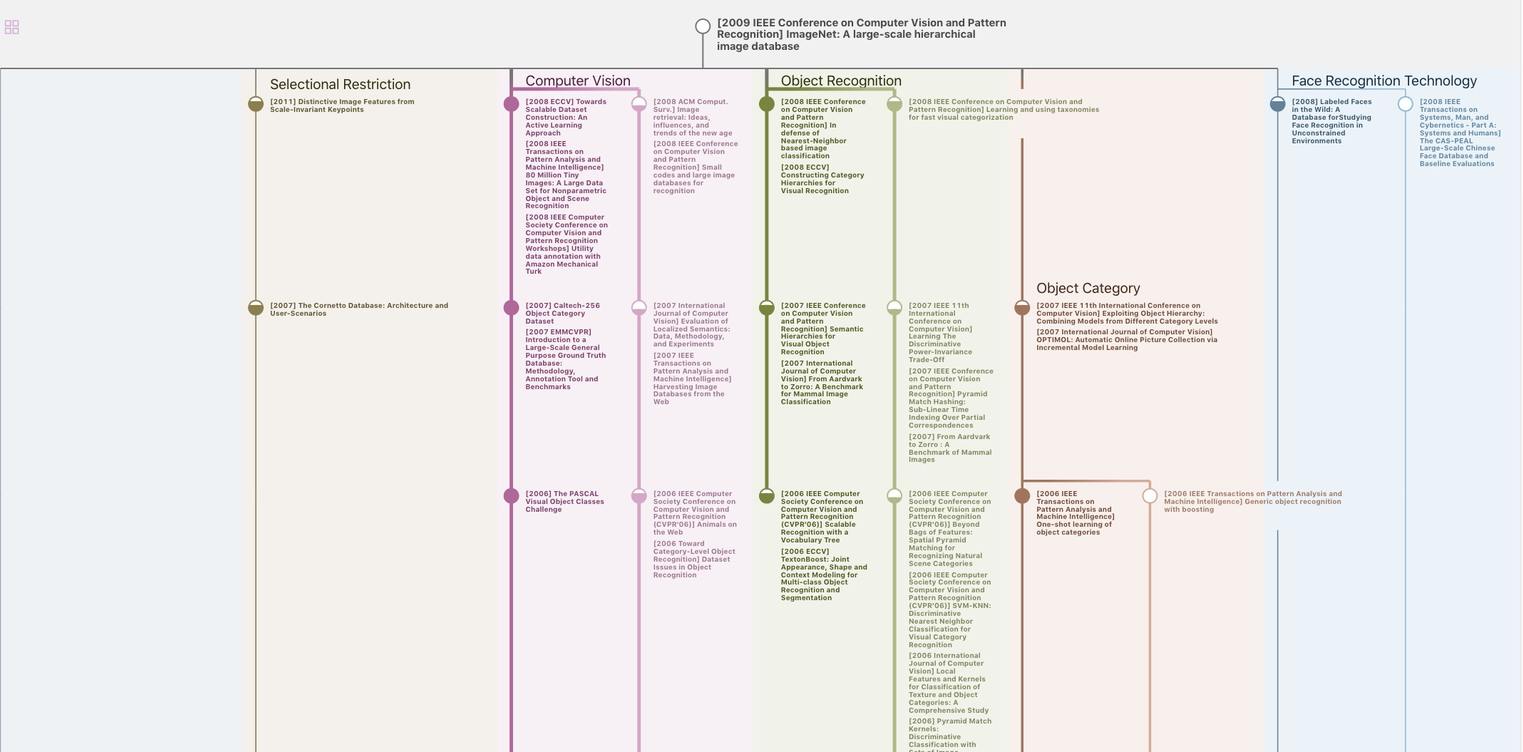
生成溯源树,研究论文发展脉络
Chat Paper
正在生成论文摘要