Social Force Embedded Mixed Graph Convolutional Network for Multi-class Trajectory Prediction
IEEE Transactions on Intelligent Vehicles(2024)
摘要
Accurate prediction of agent motion trajectories is crucial for autonomous driving, contributing to the reduction of collision risks in human-vehicle interactions and ensuring ample response time for other traffic participants. Current research predominantly focuses on traditional deep learning methods, including convolutional neural networks (CNNs) and recurrent neural networks (RNNs). These methods leverage relative distances to forecast the motion trajectories of a single class of agents. However, in complex traffic scenarios, the motion patterns of various types of traffic participants exhibit inherent randomness and uncertainty. Relying solely on relative distances may not adequately capture the nuanced interaction patterns between different classes of road users. In this paper, we propose a novel multi-class trajectory prediction method named the social force embedded mixed graph convolutional network (SFEM-GCN). The primary goal is to extract social interactions among agents more accurately. SFEM-GCN comprises three graph topologies: the semantic graph (SG), position graph (PG), and velocity graph (VG). These graphs encode various of social force relationships among different classes of agents in complex scenes. Specifically, SG utilizes one-hot encoding of agent-class information to guide the construction of graph adjacency matrices based on semantic information. PG and VG create adjacency matrices to capture motion interaction relationships between different classes agents. These graph structures are then integrated into a mixed graph, where learning is conducted using a spatio-temporal graph convolutional neural network (ST-GCNN). To further enhance prediction performance, we adopt temporal convolutional networks (TCNs) to generate the predicted trajectory with fewer parameters. Experimental results on publicly available datasets demonstrate that SFEM-GCN surpasses state-of-the-art methods in terms of accuracy and robustness.
更多查看译文
关键词
Graph convolutional network,trajectory prediction,multi-class,social interactions,autonomous driving
AI 理解论文
溯源树
样例
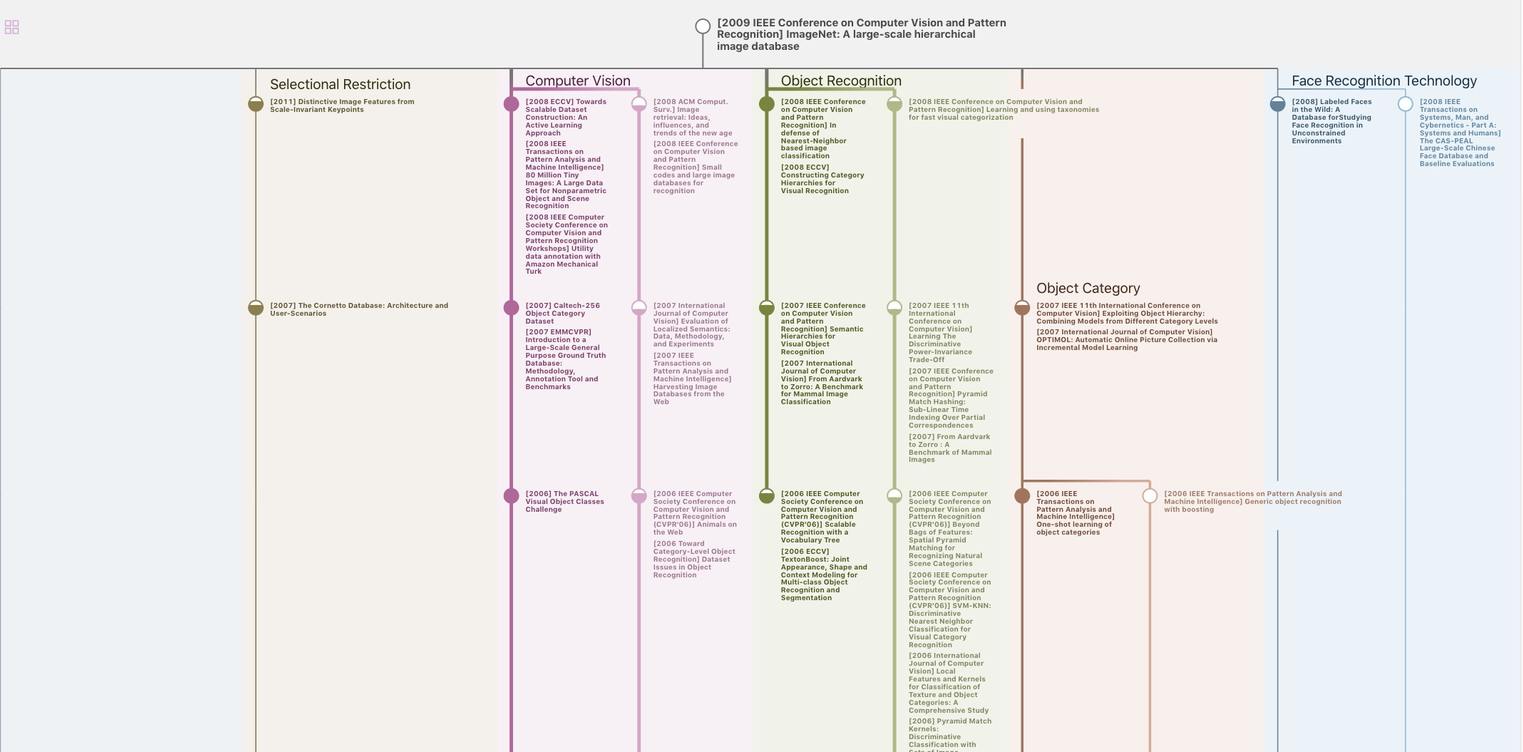
生成溯源树,研究论文发展脉络
Chat Paper
正在生成论文摘要