Relaxed Energy Preserving Hashing for Image Retrieval
IEEE TRANSACTIONS ON INTELLIGENT TRANSPORTATION SYSTEMS(2024)
摘要
Image retrieval is the eye of industrial robots, which determines the performance of machine visual search, street view search, and object grasping. Learning to hash, as a promising technique, has attracted much attention. Existing image hashing methods often directly learn hash codes by a single hash function. Despite their success, they suffer from the following limits: 1) It is difficult to perfectly preserve the intrinsic structure of the data using a single-layer hash function to generate discriminative hash codes; 2) they unconsciously ignore the main energy information of the original data, which lead to severe information loss of low-dimensional hash codes. To alleviate these issues, we propose a concise yet effective Relaxed Energy Preserving Hashing (REPH) method. Specifically, we utilize a two-layer hash function to provide more flexibility, thereby learning discriminant hash codes. The first-layer hash function projects the image data into a transition space, and the second-layer hash function narrows the semantic gap between features and hash codes. Then, we propose an energy preserving strategy to retain the energy of the original data in the transition space, thereby alleviating the energy loss of hash projecting. Moreover, the semantic reconstruction mechanism is proposed to guarantee the semantic information can be well preserved into hash codes. Extensive experiments demonstrate the superior performance of the proposed REPH on five real-world image datasets.
更多查看译文
关键词
Codes,Semantics,Image retrieval,Hash functions,Feature extraction,Visualization,Image reconstruction,Similarity retrieval,energy preserving,learning to hash,transition space
AI 理解论文
溯源树
样例
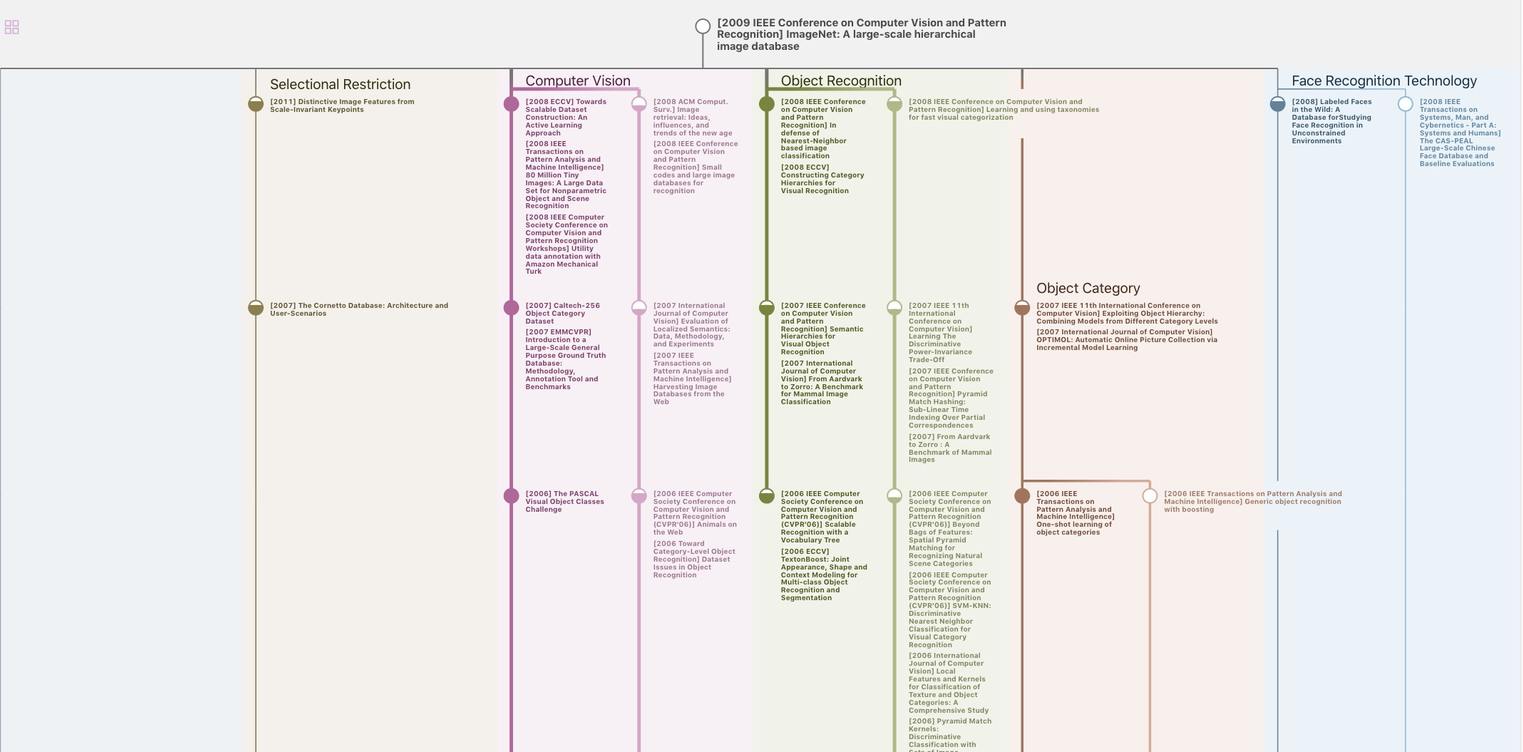
生成溯源树,研究论文发展脉络
Chat Paper
正在生成论文摘要