Adaptive Denoising-Enhanced LiDAR Odometry for Degeneration Resilience in Diverse Terrains.
IEEE Trans. Instrum. Meas.(2024)
摘要
The flexibility of Simultaneous Localization and Mapping (SLAM) algorithms in various environments has consistently been a significant challenge. To address the issue of LiDAR odometry drift in high-noise settings, integrating clustering methods to filter out unstable features has become an effective module of SLAM frameworks. However, reducing the amount of point cloud data can lead to potential loss of information and possible degeneration. As a result, this research proposes a LiDAR odometry that can dynamically assess the point cloud’s reliability. The algorithm aims to improve adaptability in diverse settings by selecting important feature points with sensitivity to the level of environmental degeneration. Firstly, a fast adaptive Euclidean clustering algorithm based on range image is proposed, which, combined with depth clustering, extracts the primary structural points of the environment defined as ambient skeleton points. Then, the environmental degeneration level is computed through the dense normal features of the skeleton points, and the point cloud cleaning is dynamically adjusted accordingly. The algorithm is validated on the KITTI benchmark and real environments, demonstrating higher accuracy and robustness in different environments.
更多查看译文
关键词
3D LiDAR odometry,point cloud segmentation,ambient skeleton,degeneration scene detection,diverse terrains
AI 理解论文
溯源树
样例
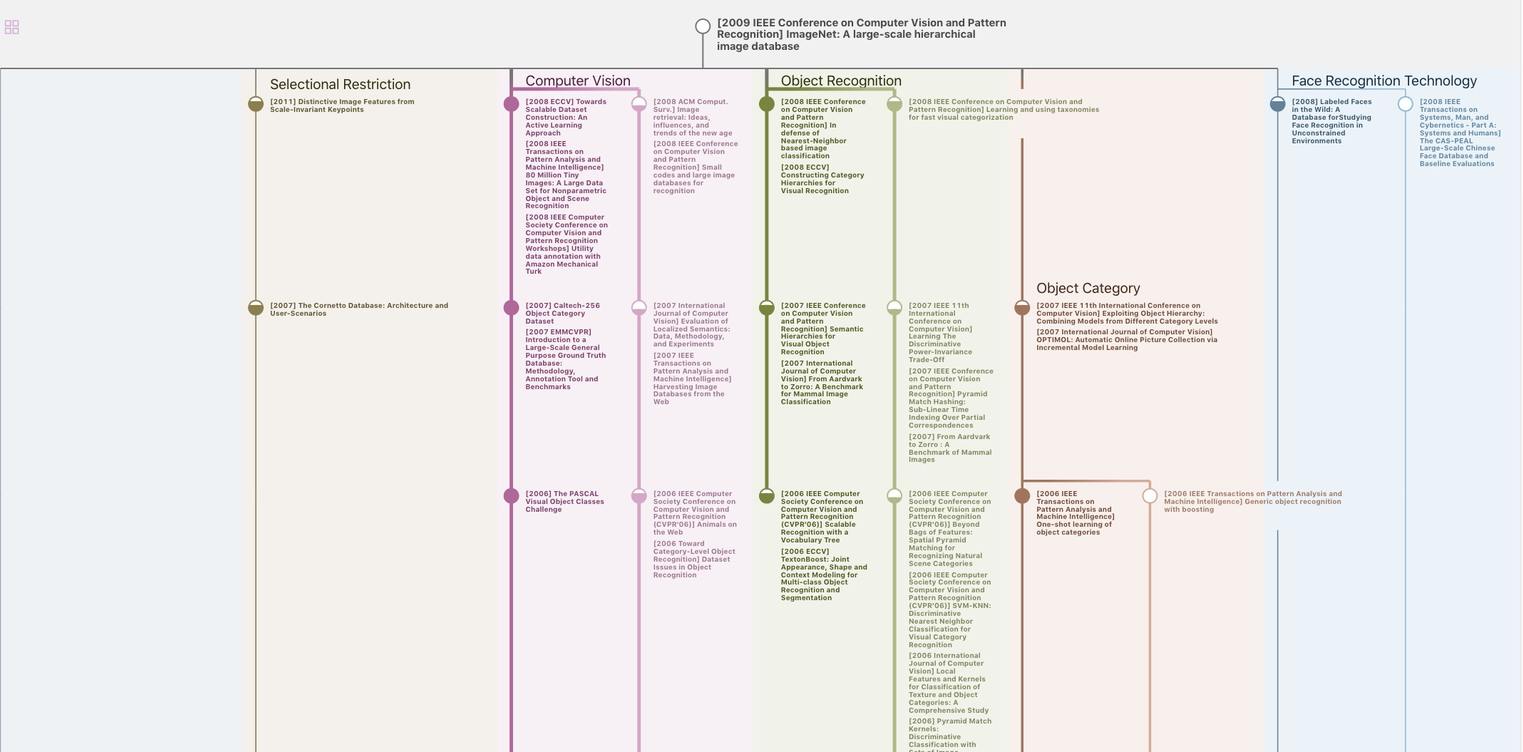
生成溯源树,研究论文发展脉络
Chat Paper
正在生成论文摘要