Multi-view Contrastive Learning for Unsupervised Domain Adaptation in Brain-Computer Interfaces
IEEE Transactions on Instrumentation and Measurement(2024)
摘要
Domain adaptation has gained significant attention to address the nonstationarity problem in electroencephalogram (EEG) data in motor imagery (MI) classification. In MI classification, domain adaptation addresses cross-session variations, enhancing the classifier’s generalization capabilities. However, existing methods have struggled to effectively capture both temporal and spatial features, resulting in limited classification accuracy. To tackle this issue, we propose the multi-view adversarial contrastive network (MACNet). The proposed MACNet simultaneously learns spatial and temporal features in two different views: Euclidean and Riemannian. Furthermore, we introduce a multi-level domain mixup technique to enhance domain aligment at both signal and embedding levels. The proposed MACNet method is evaluated on three public datasets. It achieves an accuracy of 83.79% on the BCI Competition IV dataset, 80.00% on the OpenBMI dataset, and 85.83% on SMR datset that outperforms previous methods in cross-session transfer learning.
更多查看译文
关键词
Electroencephalography,Multi-View Contrastive,Riemannian Neural Network,Unsupervised Domain Adaptation,Neural network,Transfer learning
AI 理解论文
溯源树
样例
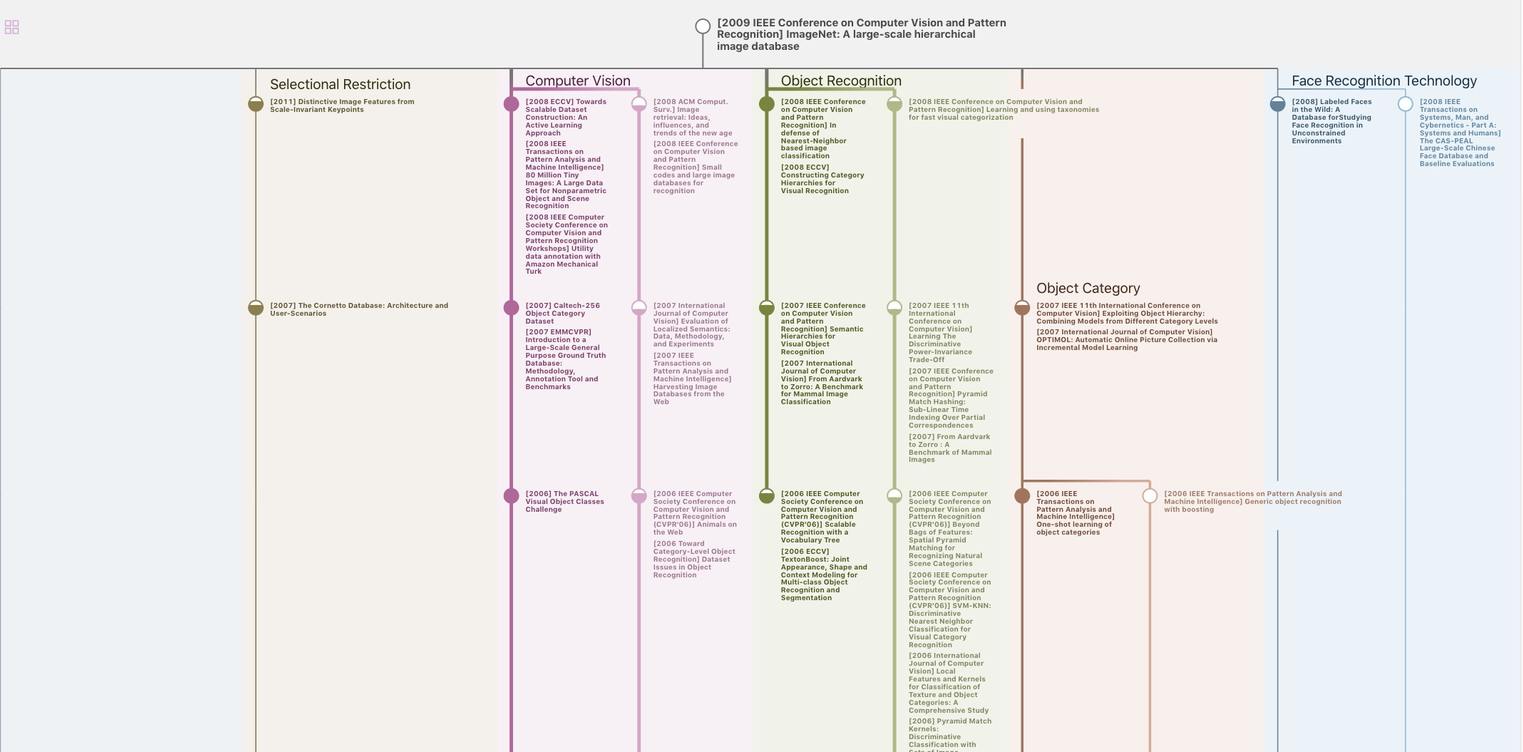
生成溯源树,研究论文发展脉络
Chat Paper
正在生成论文摘要