Ensemble Transfer Strategy Based on Domain Difference for Robot Multiple Peg-in-Hole Assembly
IEEE Transactions on Industrial Electronics(2024)
摘要
Transfer learning and ensemble learning are currently emerging trends in robotics, playing a vital role in the generalization of robot skills for manipulation tasks. In the face of new tasks, previous methods either learn from scratch or require substantial data and experience specific to the target domain, significantly hindering the generalization of robot skills. In this article, an ensemble transfer learning method is proposed based on domain differences. First, a hybrid approach that combines sequential and parallel ensemble learning strategies is designed. The experience of strategies in different domains can be reused in the target domain through ensemble transfer. Second, a strategy weight adjustment method is designed by considering the differences among various domains. This allows the strategies of the source domains that are more similar to the target domain to be assigned greater weights, thus maximizing the utilization of the knowledge of the source domains. The ensemble strategy not only exhibits good generalization for new tasks but also has excellent memory for old tasks. This article combines sequential and parallel ensemble learning methods based on domain difference, which can quickly generalize strategies from multiple source domains to the target domain without learning in the target domain. The dominant base strategies are enhanced through weight adjustment, and the adverse impact of poor base strategies is avoided on the overall strategy. A series of simulation and real multiple peg-in-hole assembly experiments is conducted to verify the superiority of the proposed method.
更多查看译文
关键词
Domain difference,ensemble transfer,robot assembly,weight adjustment
AI 理解论文
溯源树
样例
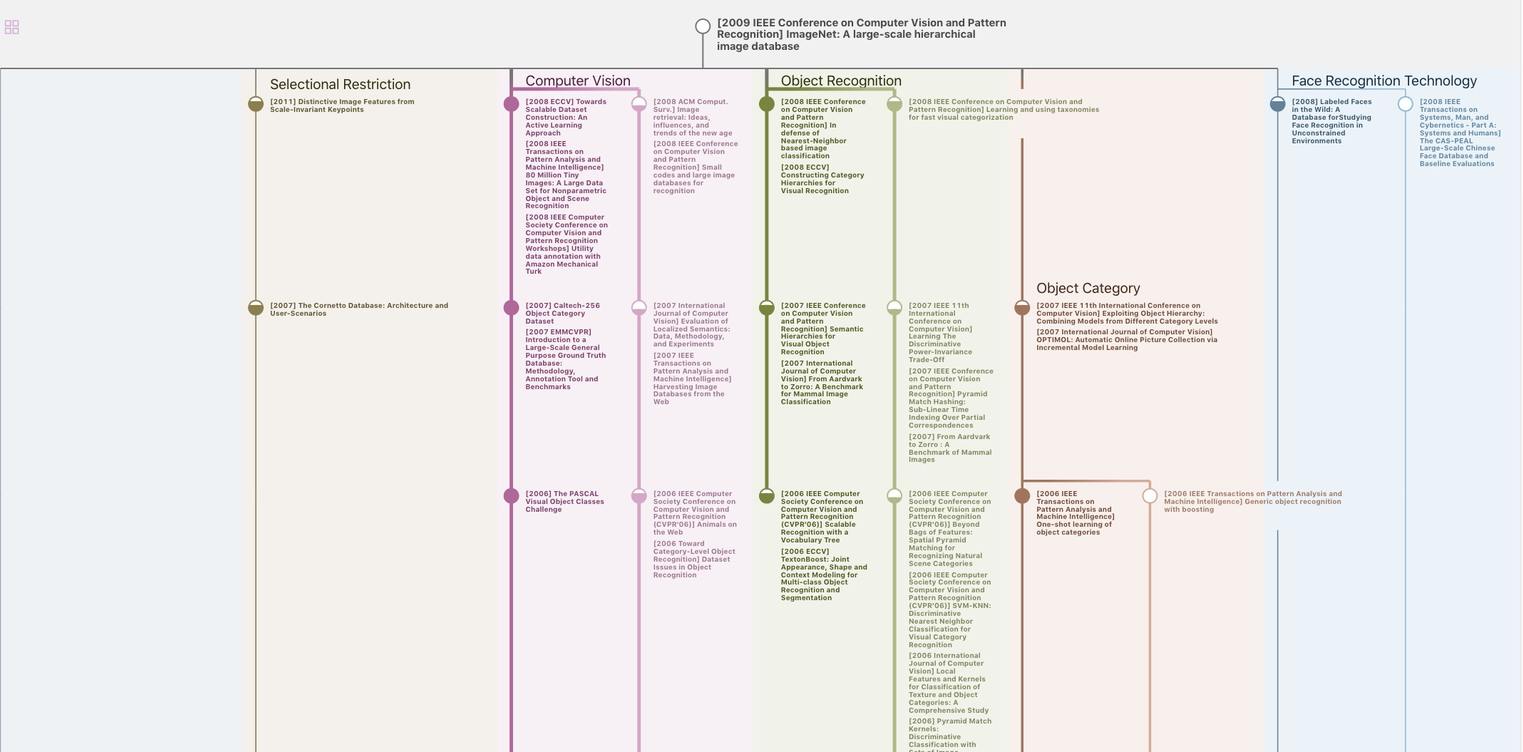
生成溯源树,研究论文发展脉络
Chat Paper
正在生成论文摘要