Variable Weight Combination Model for Lane-changing Prediction of Human-Driven Vehicle
IEEE Transactions on Intelligent Vehicles(2024)
摘要
Accurate lane-changing prediction is important to Advanced Driver Assistance Systems (ADAS). However, the prediction accuracy declines when the lateral motion is ambiguous during the early stage of lane change or when vehicles abruptly cease the process for safety concerns. This study proposes a variable weight combination (VWC) model to predict human-driven vehicles' (HDV) lane-changing. Speed gain and comfort gain are selected to describe lane change demand. An improved safety potential field model that considers different vehicle types is established to represent lane change conditions. Two Random Forest (RF) models are initially trained using vehicle and lane change demand, vehicle motion, and lane change condition as input features to extract the probabilities of different driving intentions. The dynamic weights of the two RF models are obtained based on the logistic function to achieve the final prediction. The results show that the accuracy of the proposed model reaches 89.14% on average within 3s duration before lane change point on NGSIM(I-80), the accuracy of the VWC model has increased by about 5% when compared to independent RF models and maintains an 83.69% accuracy even under challenging N(0, 0.2) noise condition. Notably, at a 3s prediction time, the VWC model outperforms Long Short-Term Memory and XGBoost by approximately 10%. Furthermore, the accuracy of the VWC model on NGSIM (US101) and HighD datasets sustains 86.81% and 88.02%, respectively, demonstrating its generalization ability. The research findings contribute to the advancement of ADAS by enabling the prediction of neighboring HDV driving intention, thereby enhancing driving safety and efficiency.
更多查看译文
关键词
lane change prediction,variable weight combination model,safety potential field,lane change demand,lane change condition
AI 理解论文
溯源树
样例
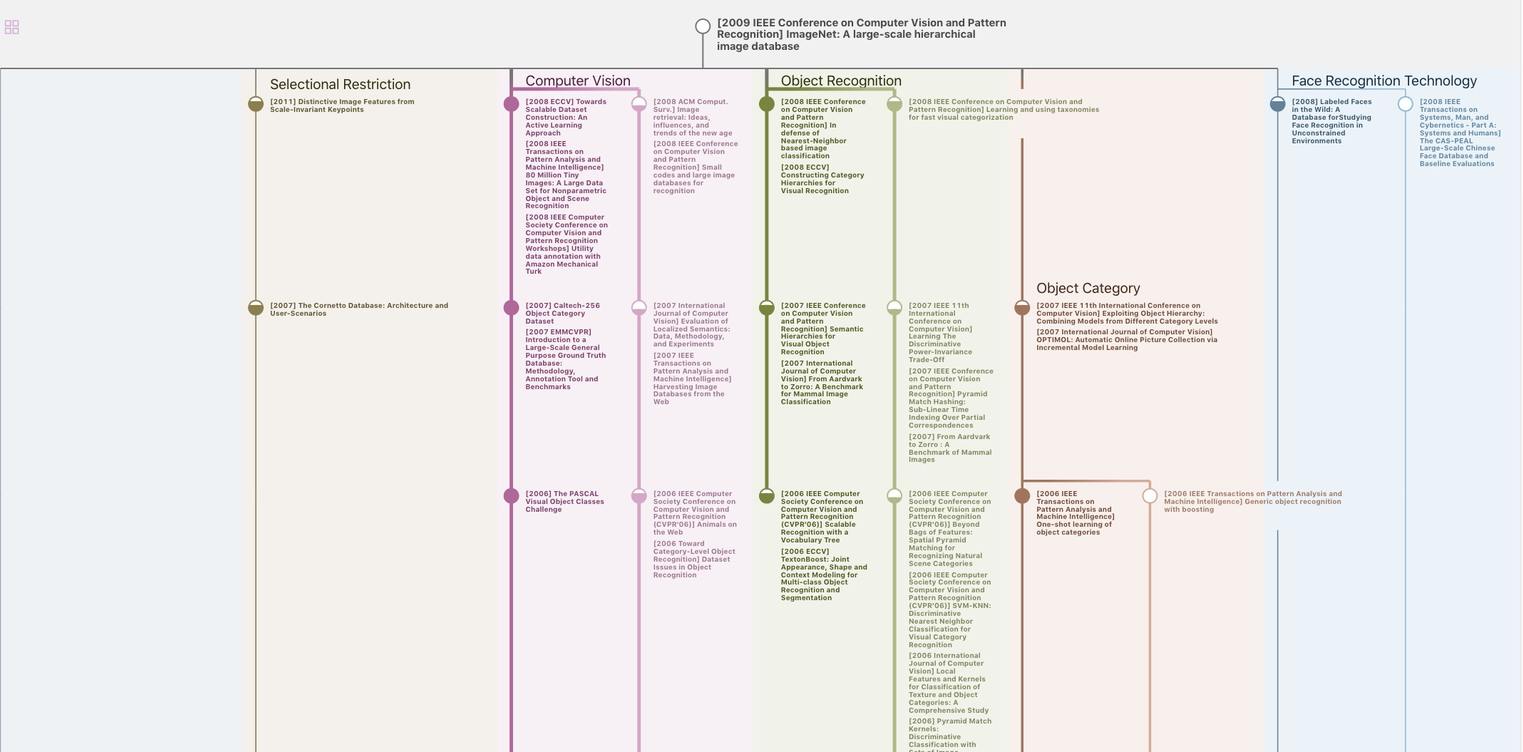
生成溯源树,研究论文发展脉络
Chat Paper
正在生成论文摘要