Feedback Spatial-Temporal Infrared Small Target Detection Based on Orthogonal Subspace Projection
IEEE TRANSACTIONS ON GEOSCIENCE AND REMOTE SENSING(2024)
摘要
Infrared (IR) small target detection plays an essential role in many civilian and military fields. Low-rank and sparse decomposition-based detection techniques have gradually shown superiority. However, there are two crucial issues. The first is accurate low-rank background estimation; the second is effective target enhancement and background suppression. To address the abovementioned issues, we propose a method named feedback spatial-temporal IR small target detection with framelet- and log-based improved tensor nuclear norm (FST-FLNN). Specifically, we transform the current frame to be detected and its adjacent frames into a spatial-temporal tensor D , followed by projecting D onto the principal component analysis (PCA)-driven orthogonal complement subspace to achieve (DVmPCA)-V-perpendicular to for preliminarily eliminating principal background components. To further enhance the low-rank property of residual background components and estimate it more accurately, we fully utilize the spatial-temporal information of the background and the corresponding core to improve tensor nuclear norm using log operation in the framelet domain, which is called framelet- and log-based improved tensor nuclear norm (FL-ITNN). In addition, we introduce a posterior knowledge extraction method based on extended 3-D morphology as feedback mechanisms toward the model. According to the different feedback objects of posteriors, we provide two different feedback mechanisms and their corresponding low-rank and sparse decomposition (LRSD)-based target detection model, FST-FLNN. Finally, two efficient ADMM-based solving frameworks are designed. Compared with nine state-of-the-art competitive methods, comprehensive qualitative and quantitative experiments and analysis on five real complex IR sequences illustrate the satisfactory target detectability (TD), background suppressibility (BS), and overall performance of the two versions of FST-FLNN.
更多查看译文
关键词
Tensors,Object detection,Estimation,Matrix decomposition,Detectors,Target tracking,Principal component analysis,3-D morphology,feedback mechanism,framelet,infrared (IR) small target detection,orthogonal complement subspace
AI 理解论文
溯源树
样例
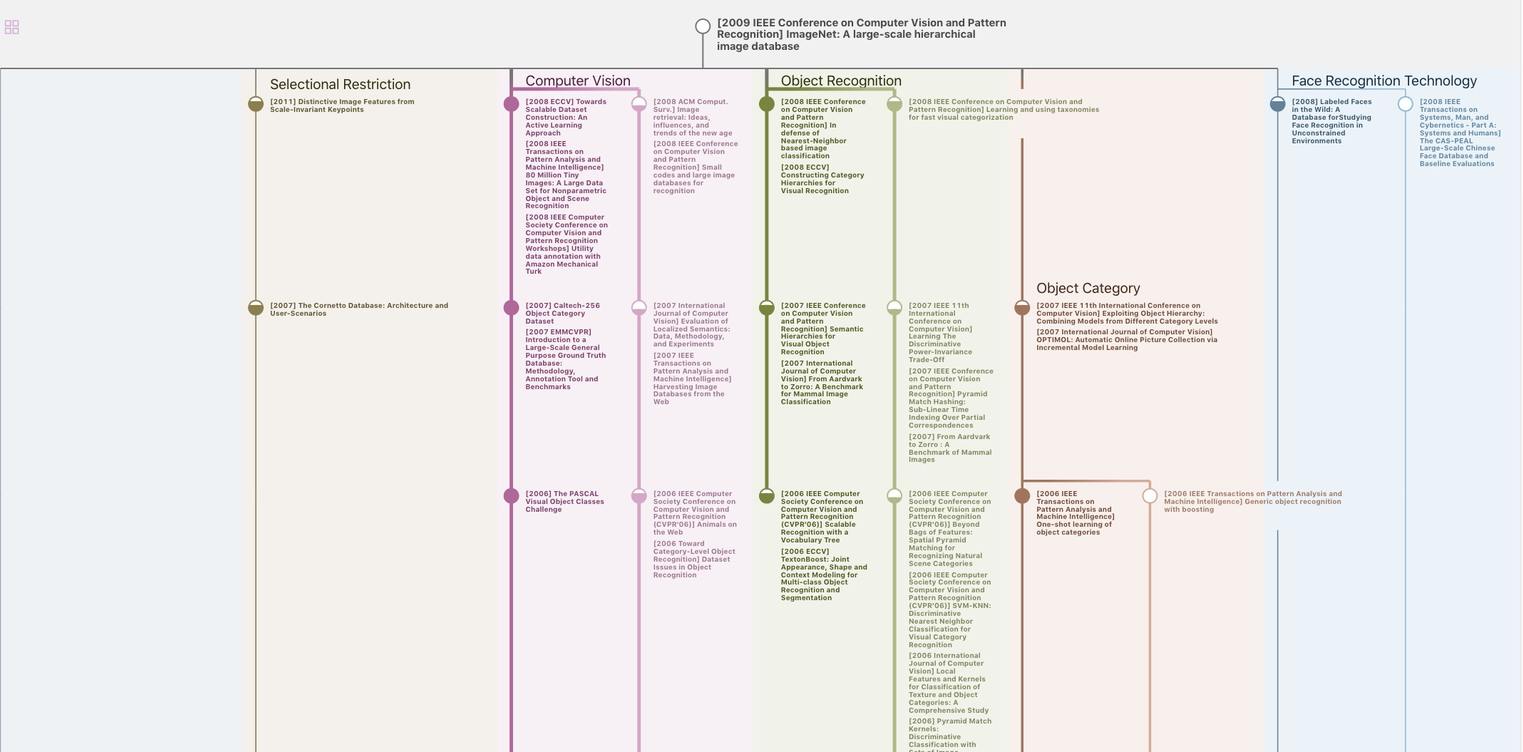
生成溯源树,研究论文发展脉络
Chat Paper
正在生成论文摘要