A Novel Network Forensic Framework for Advanced Persistent Threat Attack Attribution Through Deep Learning
IEEE TRANSACTIONS ON INTELLIGENT TRANSPORTATION SYSTEMS(2024)
摘要
The Internet now plays a pivotal role in the social and economic landspace, providing individuals and businesses with access to essential daily services and tasks. However, it has also become a breeding ground for conflicts. Advanced Persistent Threats (APTs) pose a formidable chanllenge when directed at organizations and governments, exposing the entire network to substantial security risks. Employing network fornesics for attributing cyber-attacks and acquiring timely, credible forensic results is a fundamental challenge in maintaining cyber security. This paper introduces a Deep Learning-based network forensics framework for digitally identifying and tracking network attacks, providing a comprehensive overview of the network forensics process. Specifically, we extract network traffic and employ encryption to ensure the integrity and security of data. Subsequently, we apply feature filtering techniques to retain essential traceability information, and Deep Learning model parameters are automatically optimized using hyperparameter optimization techniques. Lastly, we develop a Multi-Layer Perceptual Deep Neural Network (MLP DNN) model with perceptual capabilities for detecting anomalous events within the network. We evaluated the framework's effectiveness using the UNSW-NB15 dataset. The experiments demonstrate that the proposed framework is applicable to APT attack forensics scenarios. In comparison to other AI methods, our framework excels in discovering and tracking network attack events with high performance.
更多查看译文
关键词
Network forensic,advanced persistent threat,deep learning,Pearson correlation,multi-layer perceptual deep neural network
AI 理解论文
溯源树
样例
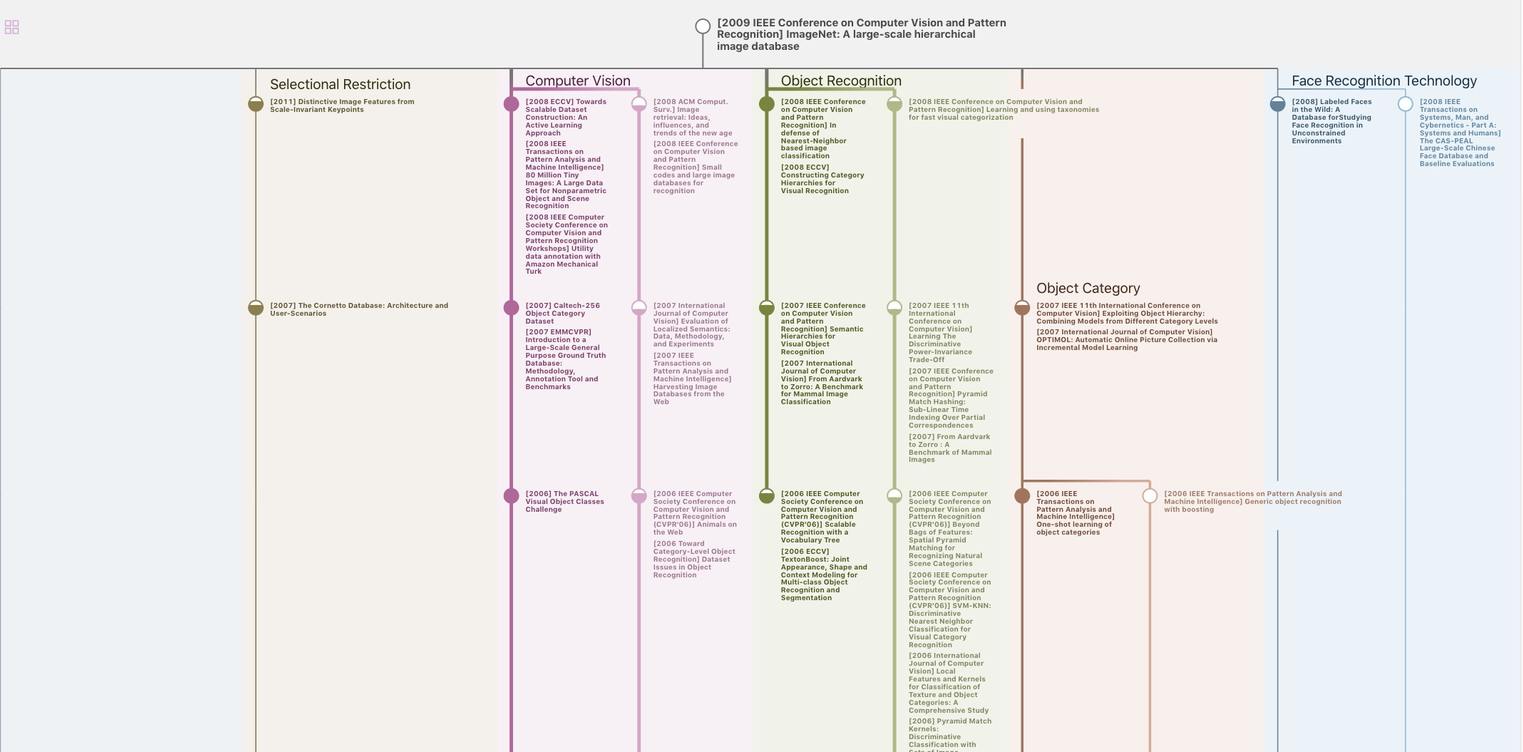
生成溯源树,研究论文发展脉络
Chat Paper
正在生成论文摘要