Engravings, Secrets, and Interpretability of Neural Networks
IEEE Transactions on Emerging Topics in Computing(2024)
摘要
This work proposes a definition and examines the problem of undetectably engraving special input/output information into a Neural Network (NN). Investigation of this problem is significant given the ubiquity of neural networks and society's reliance on their proper training and use. We systematically study this question and provide (1) definitions of security for secret engravings, (2) machine learning methods for the construction of an engraved network, (3) a threat model that is instantiated with state-of-the-art interpretability methods to devise distinguishers/attackers. In this work, there are two kinds of algorithms. First, the constructions of engravings through machine learning training methods. Second, the distinguishers associated with the threat model. The weakest of our engraved NN constructions are insecure and can be broken by our distinguishers, whereas other, more systematic engravings are resilient to each of our distinguishing attacks on three prototypical image classification datasets. Our threat model is of independent interest, as it provides a concrete quantification/benchmark for the “goodness” of interpretability methods.
更多查看译文
关键词
Backdoor attack,data poisoning,engraving,interpretability,machine learning,neural net,security
AI 理解论文
溯源树
样例
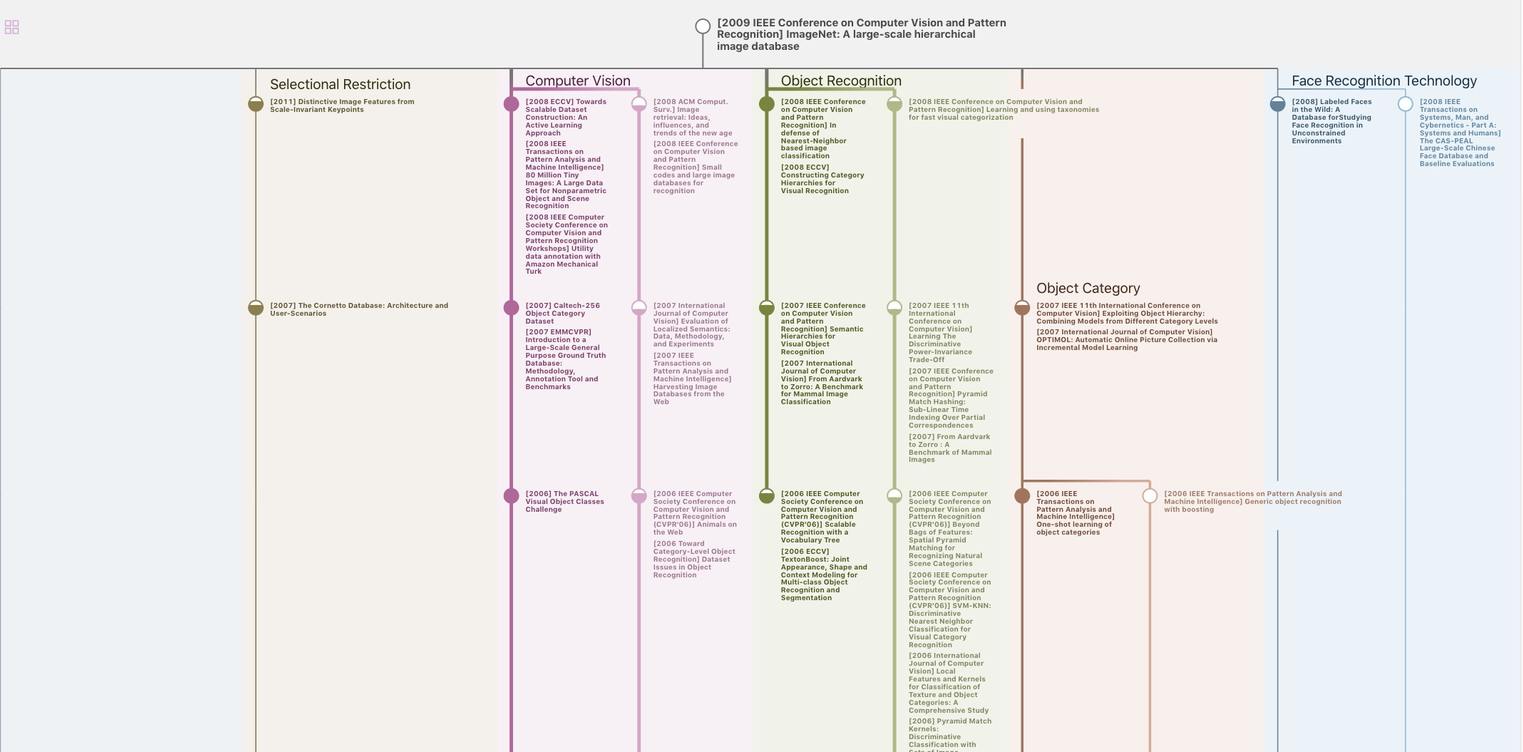
生成溯源树,研究论文发展脉络
Chat Paper
正在生成论文摘要