STO-DARTS: Stochastic Bilevel Optimization for Differentiable Neural Architecture Search
IEEE TRANSACTIONS ON EMERGING TOPICS IN COMPUTATIONAL INTELLIGENCE(2024)
摘要
Differentiable bilevel Neural Architecture Search (NAS) has emerged as a powerful approach in automated machine learning (AutoML) for efficiently searching for neural network architectures. However, the existing differentiable methods encounter challenges, such as the risk of becoming trapped in local optima and the computationally expensive Hessian matrix inverse calculation performed when solving the bilevel NAS optimization model. In this paper, a novel-but-efficient stochastic bilevel optimization approach, called STO-DARTS, is proposed for the bilevel NAS optimization problem. Specifically, we design a hypergradient estimate, which is constructed using stochastic gradient descent from the gradient information contained in the Neumann series. This estimate alleviates the issue of local optima traps, enabling searches for exceptional network architectures. To validate the effectiveness and efficiency of the proposed method, two versions of STO-DARTS with different hypergradient estimators are constructed and experimentally tested on different datasets in NAS-Bench-201 and DARTS search spaces. The experimental results show that the proposed STO-DARTS approach achieves competitive performance with that of other state-of-the-art NAS methods in terms of determining effective network architectures. To support our approach, we also provide theoretical analyses.
更多查看译文
关键词
Neural Architecture Search,Bilevel Optimization,Hypergradient Estimator
AI 理解论文
溯源树
样例
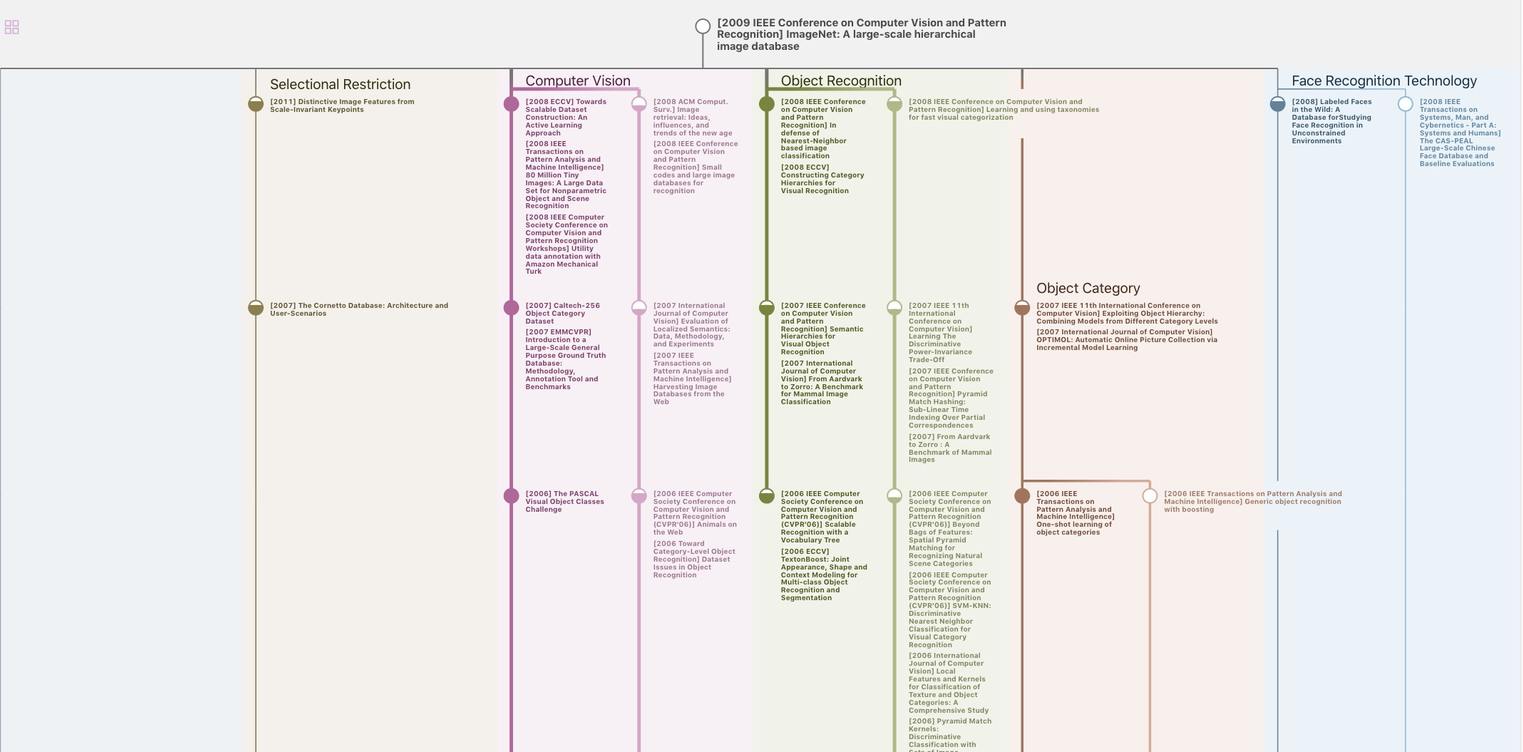
生成溯源树,研究论文发展脉络
Chat Paper
正在生成论文摘要