A Multi-Population Based Evolutionary Algorithm for Many-Objective Recommendations
IEEE TRANSACTIONS ON EMERGING TOPICS IN COMPUTATIONAL INTELLIGENCE(2024)
摘要
Multi-objective evolutionary algorithms (MOEAs) have been proved to be competitive in recommender systems. As the application scenarios of recommender systems become increasingly complex, the number of objectives to be considered in the recommender systems increases. However, most existing multi-objective recommendation algorithms lead to increased environmental selection pressure as the number of objectives increases. To tackle the issue, in this paper, we propose a multi-population based evolutionary algorithm named MP-MORS for many-objective recommendations, where two subpopulations and one major population are used to evolve and interact to find high-quality solutions. Specifically, the objectives are firstly divided into those evaluated on individual users (defined as IndObjectives) and those evaluated on all users (defined as as AllObjectives). Then two subpopulations are suggested to optimize the two types of objectives respectively, with which the potential good solutions can be easily found. In addition, the major population considers the balance of all objectives and refines these potential good solutions. Finally, a set of high-quality solutions can be obtained by the proposed adaptive population interaction strategy. Experiments on the datasets Movielens and Douban show that the proposed MP-MORS outperforms the state-of-the-art algorithms for many-objective recommendations.
更多查看译文
关键词
Recommender systems,many-objective,evolutionary algorithms,multiple populations
AI 理解论文
溯源树
样例
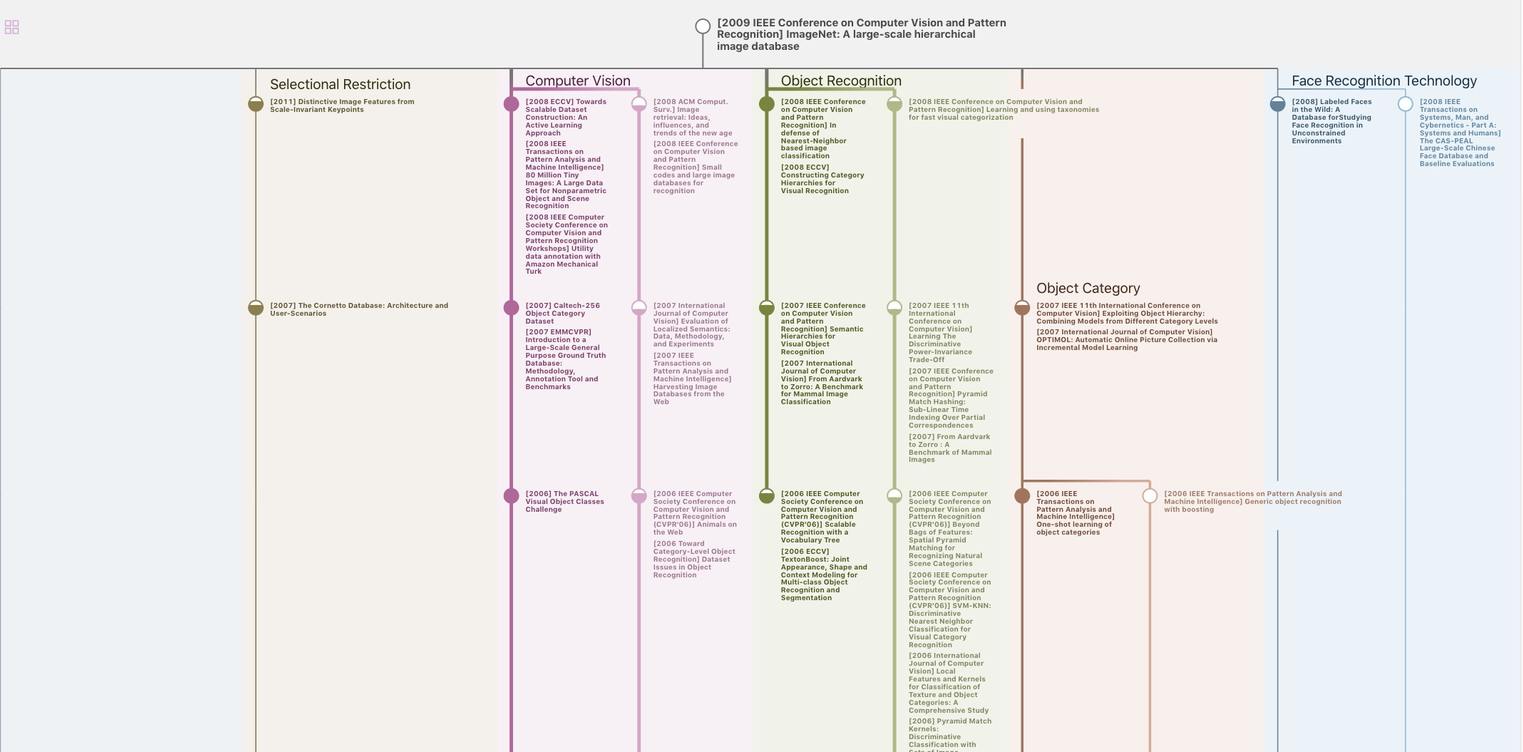
生成溯源树,研究论文发展脉络
Chat Paper
正在生成论文摘要