Multi-Score Prediction for Lung Infection Severity in Chest X-Ray Images
IEEE TRANSACTIONS ON EMERGING TOPICS IN COMPUTATIONAL INTELLIGENCE(2024)
摘要
Lung diseases, including COVID-19, can be considered a serious health problem. In order for patients to be identified to make better decisions and receive the appropriate treatment that can save their lives, rapid and accurate diagnosis of pulmonary infections is critical. In this study, we propose a multi-task deep learning algorithm to quickly and effectively determine the severity of pulmonary infection in patients with COVID-19 or similar pulmonary diseases. The main contributions are as follows. First, we propose a multi-task network based on a dual transformer encoder followed by a feature fusion module feeding two MLP regression heads. Second, we introduce an online combination of region and score for image augmentation. The resulting network quantifies CXR images with two scores that characterize two types of infection. One score is based on the spread of infection in the lung, while the other measures how opaque the infected sites are. The proposed approach is evaluated using chest radiographs (CXR) from the public RALO dataset. Compared to prediction models in the literature, our multi-task technique outperforms them. At the time of inference, the proposed model has the highest Pearson correlation coefficient and the lowest mean absolute error. Our results show that accurate diagnosis of lung severity can be achieved by generating two assessment scores in a multi-tasking model.
更多查看译文
关键词
Lung,Multitasking,COVID-19,Predictive models,Training,Germanium,Imaging,quantification of infection severity,chest X-ray image analysis,computer vision,deep learning,multi-task Learning
AI 理解论文
溯源树
样例
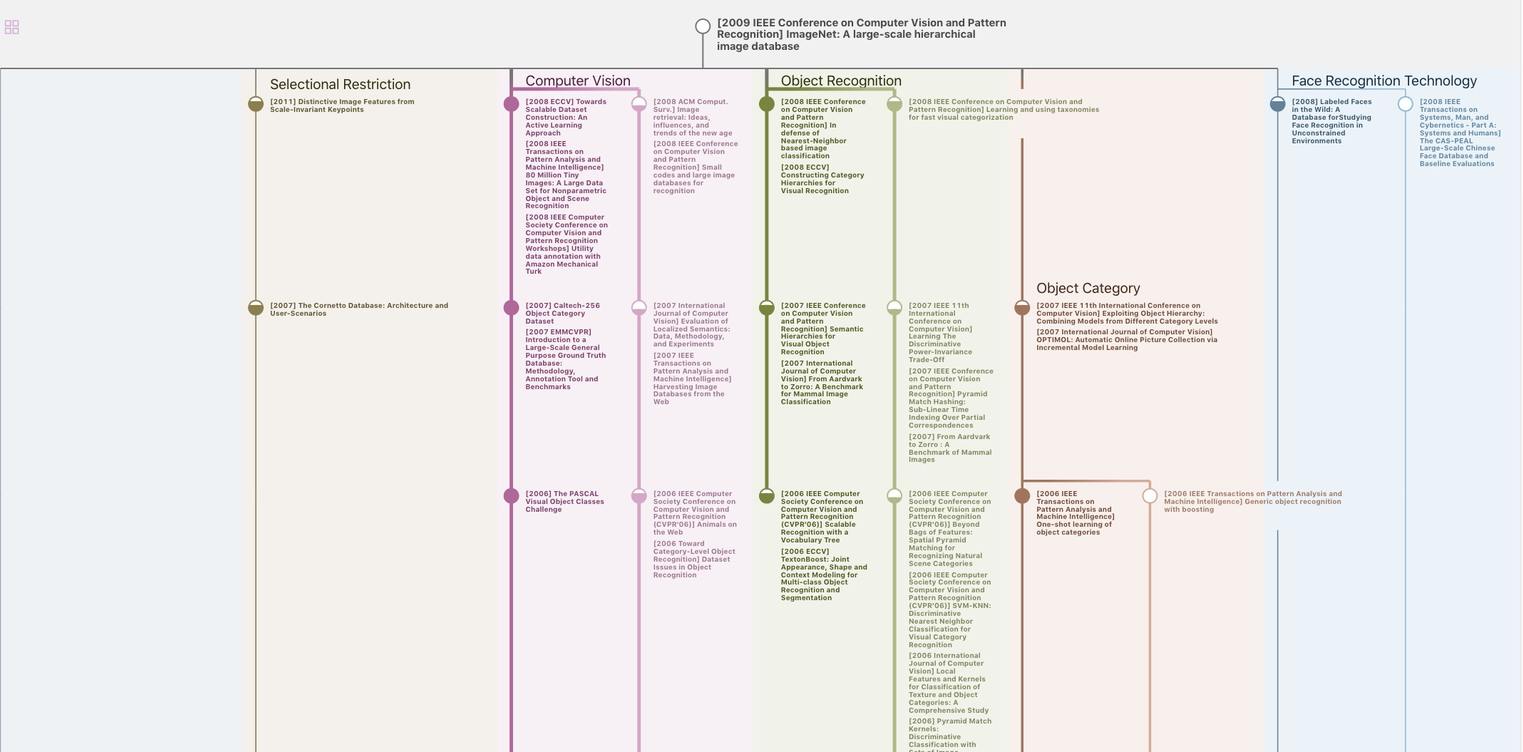
生成溯源树,研究论文发展脉络
Chat Paper
正在生成论文摘要