A 1-8b Reconfigurable Digital SRAM Compute-in-Memory Macro for Processing Neural Networks
IEEE TRANSACTIONS ON CIRCUITS AND SYSTEMS I-REGULAR PAPERS(2024)
摘要
This work presents a 1-8b reconfigurable digital SRAM compute-in-memory (CIM) macro, which significantly improves array utilization and energy efficiency under different input and weight configurations compared to previous works. To ensure the array utilization under different configurations, a row-based bitwise-summation-first digital CIM architecture is proposed. In addition, to realize flexible switching between signed and unsigned operations, a complete 2's complement encoding method is adopted, which makes the computation of the sign bits consistent with that of the magnitude bits when performing signed operations, thus ensuring that each row of the CIM array can store the sign of the weight. Due to the support of reconfigurable bit width, the proposed CIM macro can be widely used in various neural networks for optimal efficiency. In order to better apply the CIM macro to binarized neural networks, a configurable bitwise multiplier is presented, which supports both AND and XNOR operations. Moreover, since the power consumption of the adder tree occupies a major part of the digital CIM macro, a 4-2 compressor based adder tree is presented to further improve the energy efficiency. Measurement results based on 55nm CMOS process show that the proposed CIM macro achieves an energy efficiency of up to 2238TOPS/W at 1b/1b and 44.82TOPS/W at 4b/4b MAC operations.
更多查看译文
关键词
SRAM,compute-in-memory,reconfigurable,neural networks,array utilization,energy efficiency
AI 理解论文
溯源树
样例
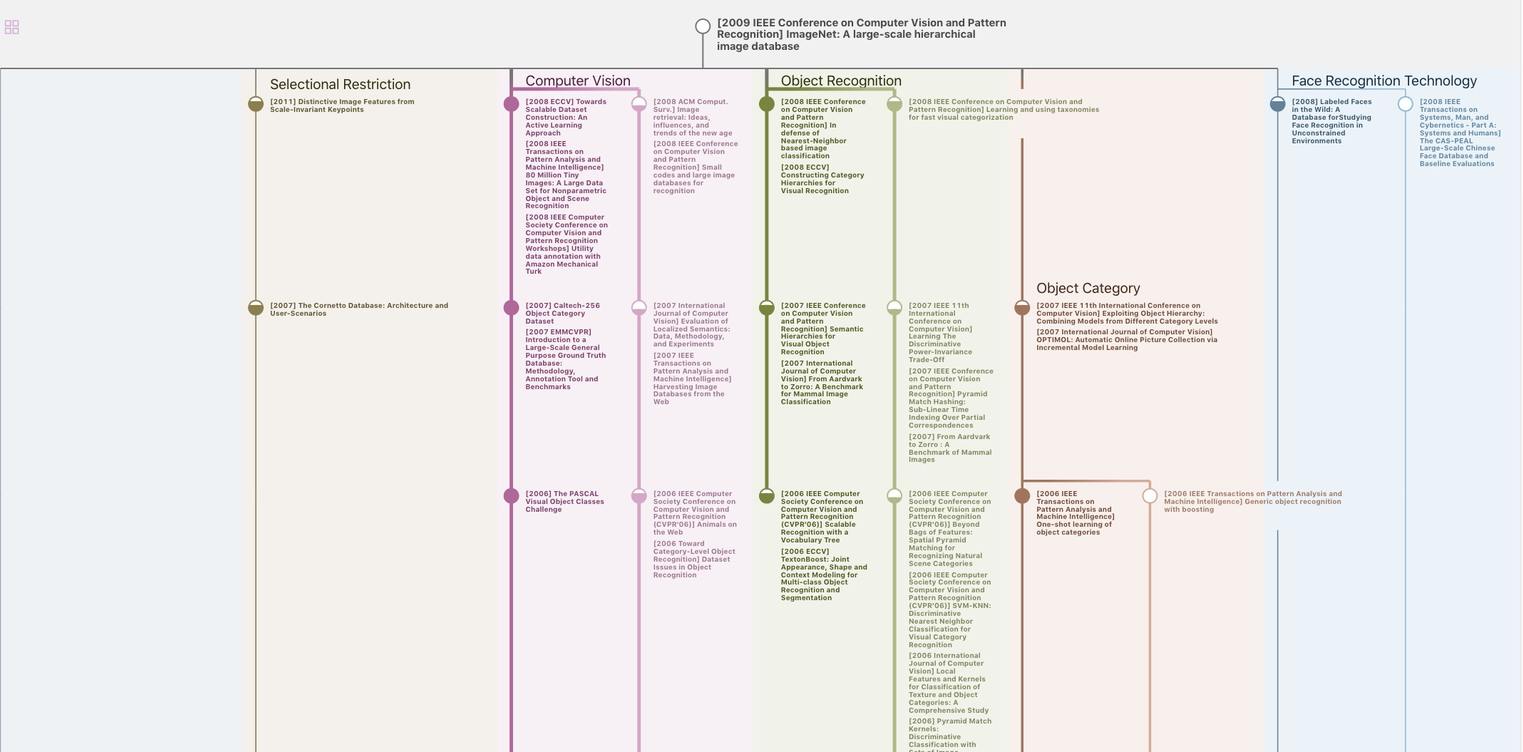
生成溯源树,研究论文发展脉络
Chat Paper
正在生成论文摘要