Explainable Impact of Partial Supervision in Semi-Supervised Fuzzy Clustering
IEEE Transactions on Fuzzy Systems(2024)
摘要
Controlling the impact of partial supervision on the outcomes of modeling is of uttermost importance in semisupervised fuzzy clustering. Semi-Supervised Fuzzy C-Means (SSFCMeans), a specific model we consider, uses a single hyperparameter called a scaling factor α to weigh the impact of partially labeled data. This concept became widespread and was reused directly in many works building on SSFCMeans, or even applied to other fuzzy clustering algorithms such as Possibilistic C-Means. However, none of the works challenged the original interpretation of α which suggests that the impact of partial supervision is directly proportional to the scaling factor. We fill the above research gap and thoroughly analyze this relationship. We provide novel explanations of the scaling factor α in terms of the key element of fuzzy clustering - the membership values. We prove that the impact of partial supervision is a non-linear function of α. Our approach is rooted in the explainability framework, which distinguishes interpretation from an explanation and treats the latter as superior. Explaining the scaling factor leads to an explainable impact of partial supervision and enables greater control of it. Finally, built on the novel explanations, we propose a unified, analytically justified framework for selecting the value of the hyperparameter α that is based on the crossvalidation approach. We illustrate that the proposed framework enables an extensive analysis of the impact of partial supervision in SSFCMeans with a simulation experiment.
更多查看译文
关键词
Semi-supervised learning,Fuzzy clustering,Explainable artificial intelligence,Partial supervision,Fuzzy CMeans,Possibilistic C-Means
AI 理解论文
溯源树
样例
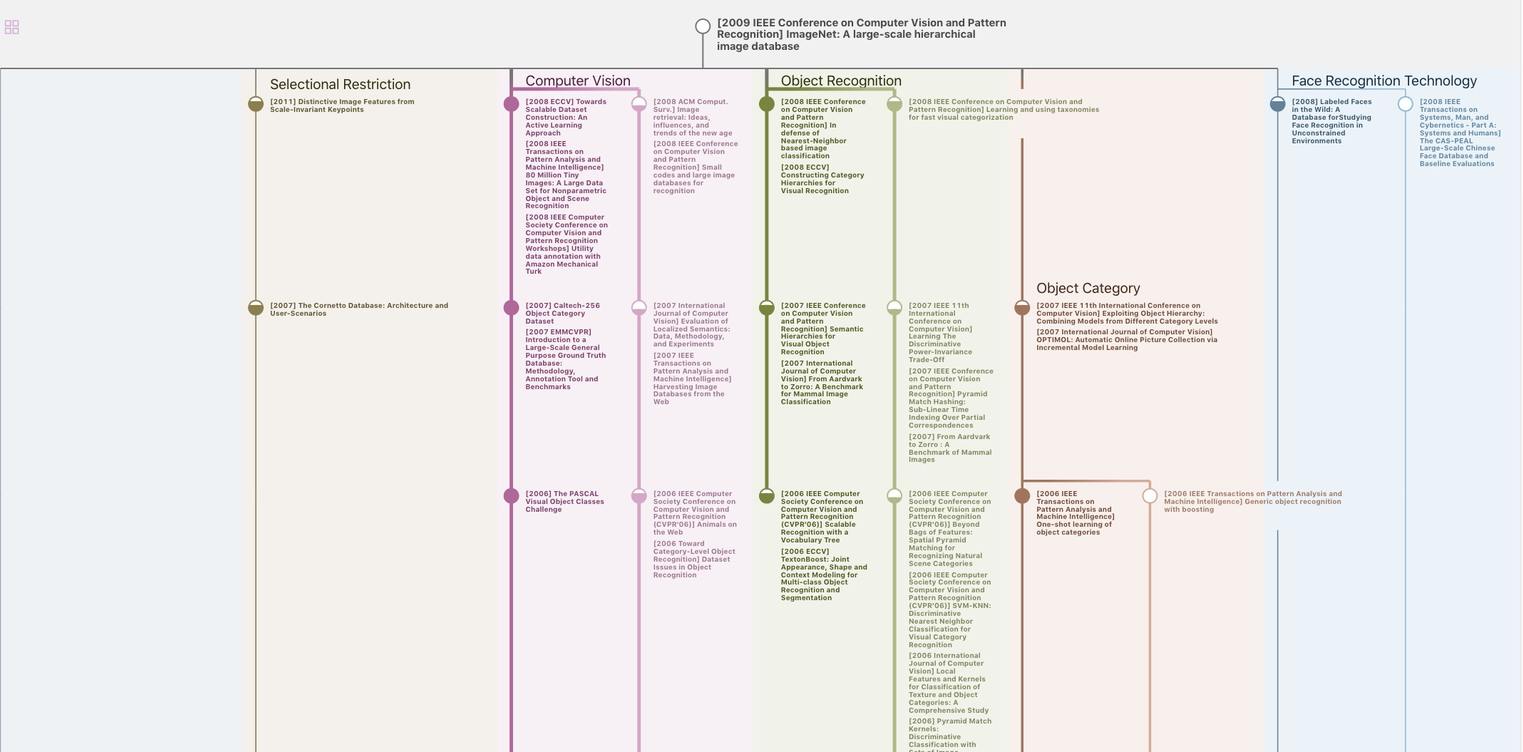
生成溯源树,研究论文发展脉络
Chat Paper
正在生成论文摘要