A novel series arc fault detection method based on CEEMDAN and IFAW-1DCNN
IEEE Transactions on Dielectrics and Electrical Insulation(2024)
摘要
Machine learning-based fault detection technology has gained significant attention in recent years. However, the practical implementation of such technologies often encounters challenges in selecting appropriate fault characteristics and network parameters. This study proposes a novel method for series arc fault detection based on the combined approach of complete ensemble empirical mode decomposition with adaptive noise (CEEMDAN) and improved fireworks algorithm-1D convolutional neural network (IFWA-1DCNN). Following CEEMDAN utilization to decompose the current signal to obtain a set of intrinsic mode functions (IMF), the Spearman correlation coefficient and energy value were utilized to select the characteristic IMF, addressing the issue of some IMF lacking or containing insufficient fault features. To address the problem of manual parameter selection for 1DCNN and the difficulty in obtaining optimal parameters, an IFWA was then proposed for parameter optimization and selection in 1DCNN. The experimental results indicated that the proposed method achieved a fault recognition accuracy of 98.23%, outperforming traditional methods that use the original current signal as input for 1DCNN. Overall, these findings highlight the enhanced recognition capability of the proposed method.
更多查看译文
关键词
Series arc fault,CEEMDAN,one-dimensional convolutional neural network,characterization intrinsic mode function extraction,improved fireworks algorithm
AI 理解论文
溯源树
样例
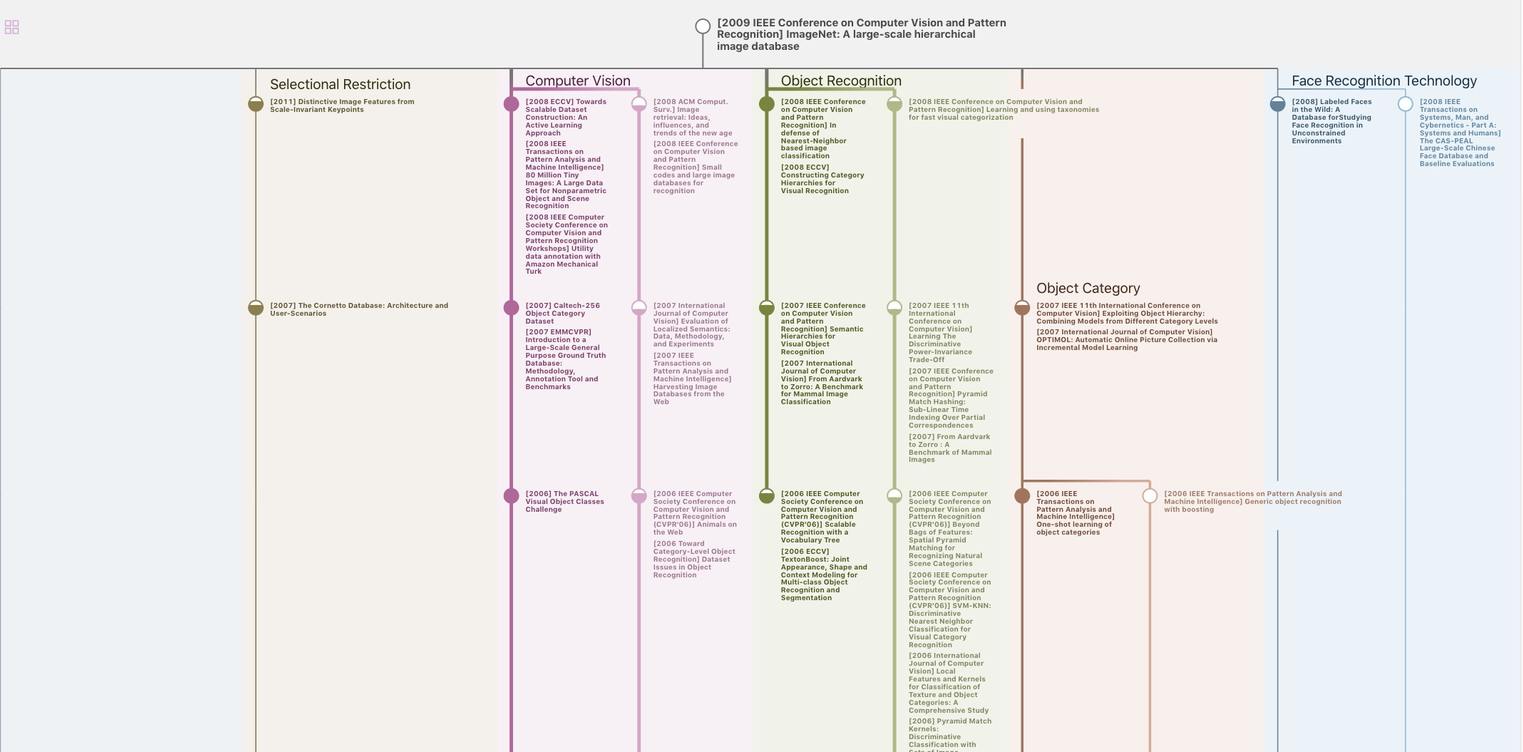
生成溯源树,研究论文发展脉络
Chat Paper
正在生成论文摘要