STIDNet: Identity-Aware Face Forgery Detection With Spatiotemporal Knowledge Distillation
IEEE TRANSACTIONS ON COMPUTATIONAL SOCIAL SYSTEMS(2024)
摘要
The impressive development of facial manipulation techniques has raised severe public concerns. Identity-aware methods, especially suitable for protecting celebrities, are seen as one of promising face forgery detection approaches with additional reference video. However, without in-depth observation of fake video's characteristics, most existing identity-aware algorithms are just naive imitation of face verification model and fail to exploit discriminative information. In this article, we argue that it is necessary to take both spatial and temporal perspectives into consideration for adequate inconsistency clues and propose a novel forgery detector named SpatioTemporal IDentity network (STIDNet). To effectively capture heterogeneous spatiotemporal information in a unified formulation, our STIDNet is following a knowledge distillation architecture that the student identity extractor receives supervision from a spatial information encoder (SIE) and a temporal information encoder (TIE) through multiteacher training. Specifically, a regional sensitive identity modelling paradigm is proposed in SIE by introducing facial blending augmentation but with uniform identity label, thus encourage model to focus on spatial discriminative region like outer face. Meanwhile, considering the strong temporal correlation between audio and talking face video, our TIE is devised in a cross-modal pattern that the audio information is introduced to supervise model exploiting temporal personalized movements. Benefit from knowledge transfer from SIE and TIE, STIDNet is able to capture individual's essential spatiotemporal identity attributes and sensitive to even subtle identity deviation caused by manipulation. Extensive experiments indicate the superiority of our STIDNet compared with previous works. Moreover, we also demonstrate STIDNet is more suitable for real-world implementation in terms of model complexity and reference set size.
更多查看译文
关键词
Deep learning,face forgery detection,knowledge distillation,video forensics
AI 理解论文
溯源树
样例
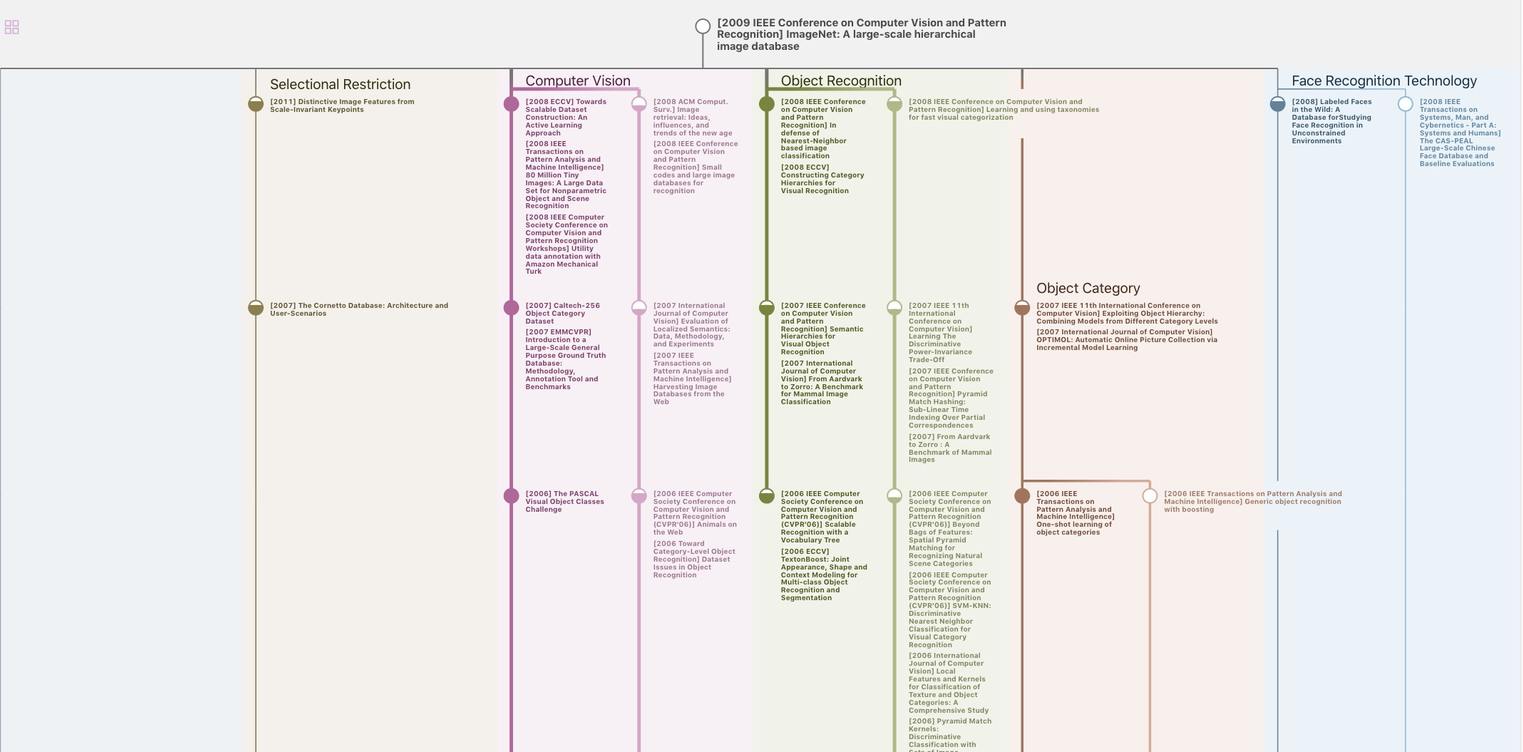
生成溯源树,研究论文发展脉络
Chat Paper
正在生成论文摘要