A Reconfigurable Near-Sensor Processor for Anomaly Detection in Limb Prostheses.
IEEE Transactions on Biomedical Circuits and Systems(2024)
摘要
This paper presents a reconfigurable near-sensor anomaly detection processor to real-time monitor the potential anomalous behaviors of amputees with limb prostheses. The processor is low-power, low-latency, and suitable for equipment on the prostheses and comprises a reconfigurable Variational Autoencoder (VAE), a scalable Self-Organizing Map (SOM) Array, and a window-size-adjustable Markov Chain, which can implement an integrated miniaturized anomaly detection system. With the reconfigurable VAE, the proposed processor can support up to 64 sensor sampling channels programmable by global configuration, which can meet the anomaly detection requirements in different scenarios. A scalable SOM array allows for the selection of different sizes based on the complexity of the data. Unlike traditional time accumulation-based anomaly detection methods, the Markov Chain is utilized to detect time-series-based anomalous data. The processor is designed and fabricated in a UMC 40-nm LP technology with a core area of 1.49 mm2 and a power consumption of 1.81 mW. It achieves real-time detection performance with 0.933 average F1 Score for the FSP dataset within 24.22 μs, and 0.956 average F1 Score for the SFDLA-12 dataset within 30.48 μs, respectively. The energy dissipation of detection for each input feature is 43.84 nJ with the FSP dataset, and 55.17 nJ with the SFDLA-12 dataset. Compared with ARM Cortex-M4 and ARM Cortex-M33 microcontrollers, the processor achieves energy and area efficiency improvements ranging from 257×, 193× and 11×, 8×, respectively.
更多查看译文
关键词
Anomaly detection,limb prostheses,near-sensor processor,variational autoencoder (VAE),self-organizing map (SOM),Markov Chain
AI 理解论文
溯源树
样例
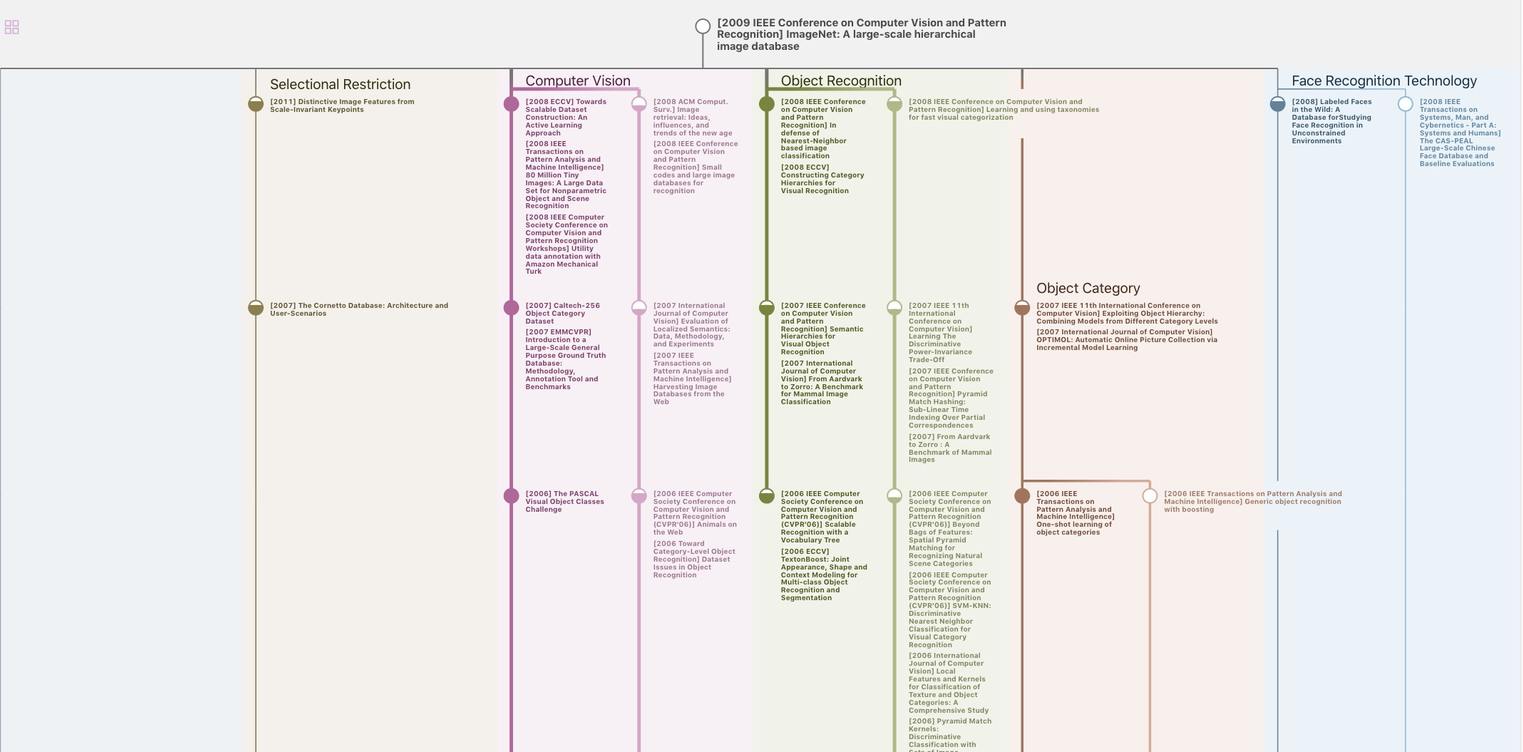
生成溯源树,研究论文发展脉络
Chat Paper
正在生成论文摘要