Transformer-Based Light Field Geometry Learning for No-Reference Light Field Image Quality Assessment
IEEE TRANSACTIONS ON BROADCASTING(2024)
摘要
Elevating traditional 2-dimensional (2D) plane display to 4-dimensional (4D) light field display can significantly enhance users' immersion and realism, because light field image (LFI) provides various visual cues in terms of multi-view disparity, motion disparity, and selective focus. Therefore, it is crucial to establish a light field image quality assessment (LF-IQA) model that aligns with human visual perception characteristics. However, it has always been a challenge to evaluate the perceptual quality of multiple light field visual cues simultaneously and consistently. To this end, this paper proposes a Transformer-based explicit learning of light field geometry for the no-reference light field image quality assessment. Specifically, to explicitly learn the light field epipolar geometry, we stack up light field sub-aperture images (SAIs) to form four SAI stacks according to four specific light field angular directions, and use a sub-grouping strategy to hierarchically learn the local and global light field geometric features. Then, a Transformer encoder with a spatial-shift tokenization strategy is applied to learn structure-aware light field geometric distortion representation, which is used to regress the final quality score. Evaluation experiments are carried out on three commonly used light field image quality assessment datasets: Win5-LID, NBU-LF1.0, and MPI-LFA. Experimental results demonstrate that our model outperforms state-of-the-art methods and exhibits a high correlation with human perception. The source code is publicly available at https://github.com/windyz77/GeoNRLFIQA.
更多查看译文
关键词
Light field,no-reference quality assessment,light field geometry,geometric distortion learning,spatial-shift tokenization
AI 理解论文
溯源树
样例
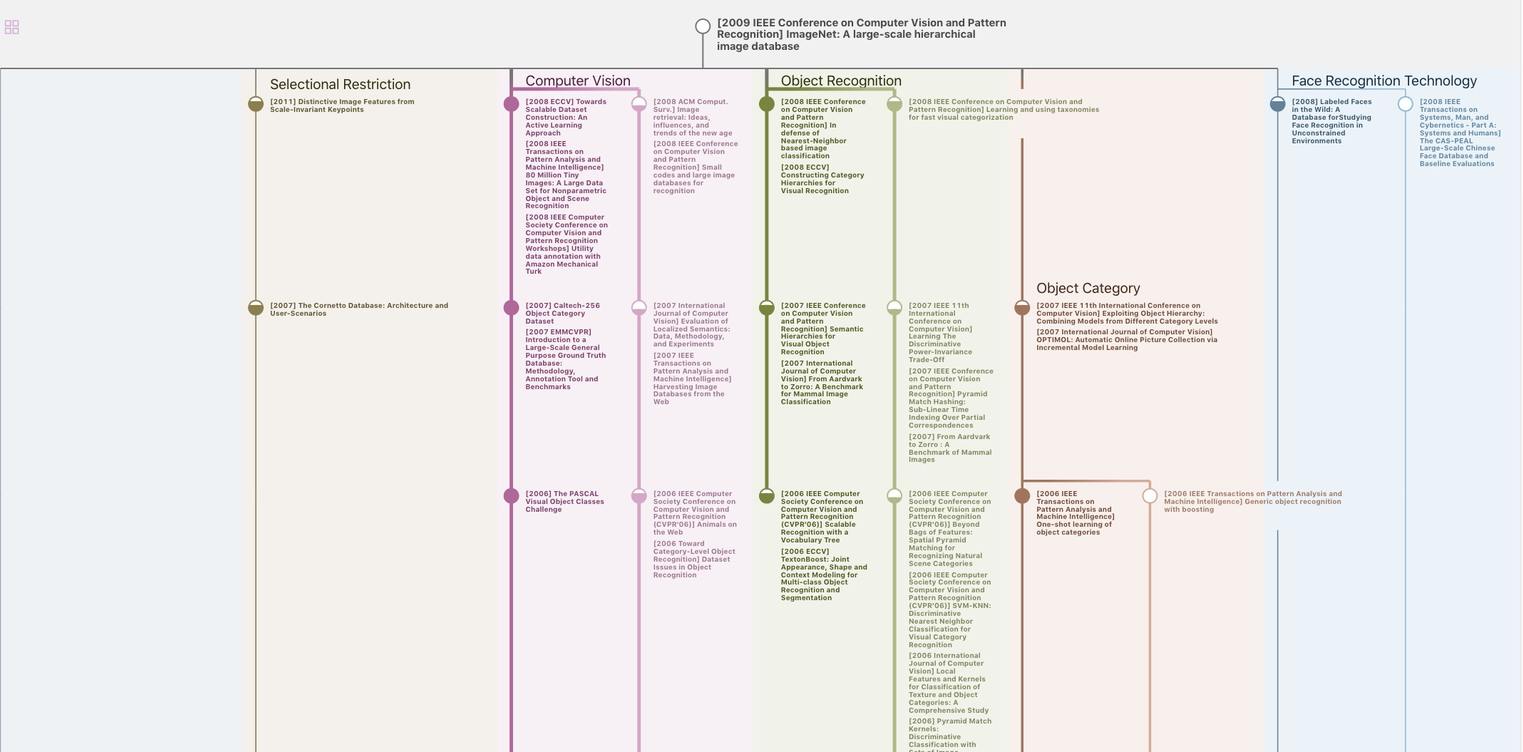
生成溯源树,研究论文发展脉络
Chat Paper
正在生成论文摘要